概率分佈一覽
閱讀設定
![]() |
以下係常用嘅概率分佈同相關概念一覽。
概率分佈(probability distribution)係指一個表明某個變數每個可能數值出現嘅機會率嘅函數,
當中 就係個概率分佈;呢個函數可以畫做一個表,X 軸代表個目標變數嘅數值,Y 軸代表嗰個目標變數嘅每個數值出現嘅機率;是但搵個變數 , 喺總體當中有一個概率分佈,表示 每個可能數值 出現嘅機率,呢個分佈喺實際上係不可知嘅,研究者淨係有得攞樣本,量度樣本當中嘅概率分佈(喺個樣本入面, 嘅每個可能數值出現嘅機率大約係幾多),靠噉嚟估計個總體嘅分佈[1]。
喺廿一世紀統計學上,比較常用嘅概率分佈有以下呢啲:
離散概率分佈
[編輯]内文:離散概率分佈
離散概率分佈(discrete probability distribution):指所描述嘅變數 嘅可能數值係離散嘅概率分佈[2]。
- 概率質量函數(probability mass function,PMF):描述一個離散概率分佈嘅函數;一個離散概率分佈嘅 PMF 會講明嗰個概率分佈嘅每一個離散可能數值出現嘅機會率[2]:
- ,啲可能性嘅機率冚唪唥加埋係 1;
- ,每個可能性嘅機率大過 0;
- ,啲可能性以外嘅數值出現嘅機會率係 0。

- 離散均勻分佈(discrete uniform distribution):每個可能離散數值出現嘅機率都一樣,概率質量函數係[2]:
- ,當中 係 有幾多個可能數值。
- 伯努利分佈(Bernoulli distribution):描述嘅變數 得兩個可能數值,數值係 1 嘅機會率係 ,數值係 0 嘅機會率係 ,概率質量函數 係[3]:
- 二項分佈(binomial distribution):描述 次結果二元嘅試驗;想像有個結果係二元-得兩個可能結果(1 同 0)-嘅試驗,例如掟銀仔,做 咁多次,每次試驗嘅結果都有 咁多機會率係 1, 咁多機會率係 0,而每次試驗嘅結果都係獨立嘅(一次試驗嘅結果唔受其他試驗嘅結果影響)。概率質量函數 ,即係得出 咁多個 1 嘅機會率係[3]:

- 幾何分佈(geometric distribution):可以指兩個唔同嘅概率分佈,兩者都涉及一個結果二元嘅試驗[6]:
- 做咗個試驗 次,終於得到 1 次陽性結果,而之前嗰啲試驗結果冚唪唥都係陰性:
- 代表要做幾多次陰性試驗,先可以得到一次陽性結果:
- 做咗個試驗 次,終於得到 1 次陽性結果,而之前嗰啲試驗結果冚唪唥都係陰性:

- 撥桑分佈(Poisson distribution):模擬嘅事件有已知嘅平均發生率,而每件事件嘅發生彼此之間獨立,發生嘅次數設做 ,概率質量函數係[7]:
- ,當中 係預期會發生嘅次數(唔一定係整數)。
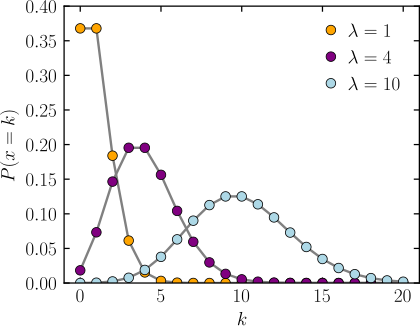
連續概率分佈
[編輯]内文:連續概率分佈
連續概率分佈(continuous probability distribution):指所描述嘅變數 嘅可能數值係連續嘅[2]。
- 概率密度函數(probability density function,PDF):描述一個連續概率分佈嘅函數;一個連續概率分佈嘅 PDF 會講明嗰個概率分佈嘅每一個可能數值出現嘅機會率大約係幾多[2],
- 。
- 均勻分佈(continuous uniform distribution,簡稱 uniform distribution):喺 (最細可能數值)同 (最大可能數值)之間嘅每個可能數值 出現嘅機會率都一樣,概率密度函數係[2]:
- 常態分佈(normal distribution):統計分析上最常用嘅概率分佈之一;喺常態分佈下,出現得最頻密嘅數值會係個平均數 ,而離平均數愈遠嘅數值就愈少會出現,畫做圖嘅話會出一條鐘形線(bell curve);常見可以用常態分佈模擬嘅變數有人類嘅智商-多數人嘅智商數值都傾向於平均數,愈極端嘅數值愈少出現,即係話好少有智商極高或者極低嘅人。常態分佈個概率密度函數係( 係個分佈嘅標準差)[1]:

- 對數正態分佈(log-normal distribution):指一個隨機變數嘅對數呈常態分佈;如果話 呢個隨機變數呈對數正態分佈嘅話,噉 呈常態分佈[8]。
- 柏里圖分佈(Pareto distribution):常用嚟模擬人口隨時間增長嘅一個概率分佈[9],概率密度函數如下[10]:
- 當中 係指 嘅最細可能數值,而 係一個正嘅參數。

- 指數分佈(exponential distribution):喺物理學上係常用嚟模擬一啲慢慢衰減嘅物理量嘅函數,例如係核衰變噉;喺統計學上,呢個函數可以用嚟模擬一啲機會率()會隨住時間()過去慢慢下降嘅事件,指數分佈嘅概率密度函數如下[11]:

分佈概念
[編輯]身高間距 | 頻率 | 累計頻率 |
---|---|---|
< 5.0 呎 | 25 | 25 |
5.0 - 5.5 呎 | 35 | 60 |
5.5 - 6.0 呎 | 20 | 80 |
6.0 - 6.5 呎 | 20 | 100 |
- 累計函數(cumulative distribution function):描述一個概率分佈之下 嘅累計值會點隨 變化嘅函數 ; 表示「由個樣本嗰度隨機抽一個個體,個個體嘅 (叫呢個值做 )細過或者等如 」嘅機會率,
- 無論連續定離散嘅概率分佈都可以有相應嘅累計函數[13]。

- 對稱度(symmetry):一個概率分佈可以有嘅一個屬性,攞個概率分佈當中嘅一個 值,個分佈喺 左邊嗰部份同個分佈喺 右邊嗰部份形狀上愈相似,個概率分佈以 為中心嘅對稱度就愈高;喺實際應用上,量度一個概率分佈嘅對稱度嗰陣會用嘅 值通常會係個分佈嘅平均值[14]。
- 動差(moment):泛指描述一個函數(例如概率分佈)嘅形狀嘅指標數值[15]。

- 抽樣分佈(sampling distribution):攞一個基於隨機抽樣嘅統計量,個統計量嘅概率分佈就係佢個抽樣分佈[17]。
- 聯合概率分佈(joint probability distribution):一個聯合概率分佈同時描述緊多過一個變數嘅分佈;一個兩變數聯合概率分佈會有打橫嘅 X 軸 Y 軸以及打戙嘅 Z 軸,總共三條軸,X 軸 Y 軸分別描述嗰兩個變數 同 嘅數值,而 X 軸同 Y 軸成嘅平面當中每一點嘅高度(Z 值)反映咗「 係呢個數值而且同時 係呢個數值」嘅機會率。當變數有多過兩個嗰陣同一道理[18]。

- 獨立同分佈(independent and identically distributed,iid):係概率論同統計學上嘅一個概念;如果話一柞隨機性變數(或者事件)係「獨立同分佈」嘅話,意思係佢哋嘅概率分佈完全一樣(每次抽嗰陣個結果嘅概率分佈一樣),而且彼此之間獨立(抽一次嘅結果唔會受打前抽到嘅數值影響)[19]。
- 中央極限定理(central limit theorem,CLT):概率論同統計學上最重要嘅定理之一;根據 CLT,想像有個變數 ,只要三條條件成立:
註釋
[編輯]睇埋
[編輯]攷
[編輯]- ↑ 1.0 1.1 Ash, Robert B. (2008). Basic probability theory (Dover ed.). Mineola, N.Y.: Dover Publications. pp. 66–69.
- ↑ 2.0 2.1 2.2 2.3 2.4 2.5 1941-, Çınlar, E. (Erhan) (2011). Probability and stochastics. New York: Springer. p. 51.
- ↑ 3.0 3.1 Bertsekas, Dimitri P. (2002). Introduction to Probability. Tsitsiklis, John N., Τσιτσικλής, Γιάννης Ν. Belmont, Mass.: Athena Scientific.
- ↑ Murphy, K. P. (2012). Machine learning: a probabilistic perspective, p. 35. MIT press.
- ↑ Ostrovski, Vladimir (May 2017). "Testing equivalence of multinomial distributions". Statistics & Probability Letters. 124: 77–82.
- ↑ Gallager, R.; van Voorhis, D. (March 1975). "Optimal source codes for geometrically distributed integer alphabets (Corresp.)". IEEE Transactions on Information Theory. 21 (2): 228–230.
- ↑ Haight, Frank A. (1967), Handbook of the Poisson Distribution, New York, NY, USA: John Wiley & Sons.
- ↑ 8.0 8.1 Johnson, Norman L.; Kotz, Samuel; Balakrishnan, N. (1994), "14: Lognormal Distributions", Continuous univariate distributions. Vol. 1, Wiley Series in Probability and Mathematical Statistics: Applied Probability and Statistics (2nd ed.), New York: John Wiley & Sons.
- ↑ Reed, William J.; et al. (2004). "The Double Pareto-Lognormal Distribution – A New Parametric Model for Size Distributions". Communications in Statistics – Theory and Methods. 33 (8): 1733–53.
- ↑ VAN MONTFORT, M.A.J. (1986). "The Generalized Pareto distribution applied to rainfall depths". Hydrological Sciences Journal. 31 (2): 151–162.
- ↑ Elfessi, Abdulaziz; Reineke, David M. (2001). "A Bayesian Look at Classical Estimation: The Exponential Distribution". Journal of Statistics Education. 9 (1).
- ↑ Manikandan, S (1 January 2011). "Frequency distribution". Journal of Pharmacology & Pharmacotherapeutics. 2 (1): 54–55.
- ↑ Deisenroth,Faisal,Ong, Marc Peter,A Aldo, Cheng Soon (2019). Mathematics for Machine Learning. Cambridge University Press. p. 181.
- ↑ 14.0 14.1 Ali, Mir M. (1980). "Characterization of the Normal Distribution Among the Continuous Symmetric Spherical Class". Journal of the Royal Statistical Society. Series B (Methodological). 42 (2): 162–164.
- ↑ Spanos, Aris (1999). Probability Theory and Statistical Inference. New York: Cambridge University Press. pp. 109–130.
- ↑ 16.0 16.1 MacGillivray, HL (1992). "Shape properties of the g- and h- and Johnson families". Communications in Statistics - Theory and Methods. 21: 1244–1250.
- ↑ 17.0 17.1 Altman, Douglas G; Bland, J Martin (2005-10-15). "Standard deviations and standard errors". BMJ: British Medical Journal. 331 (7521): 903.
- ↑ Hazewinkel, Michiel, ed. (2001) [1994], "Joint distribution", Encyclopedia of Mathematics, Springer Science+Business Media B.V. / Kluwer Academic Publishers.
- ↑ 19.0 19.1 Dinov, Ivo; Christou, Nicolas; Sanchez, Juana (2008). "Central Limit Theorem: New SOCR Applet and Demonstration Activity". Journal of Statistics Education. ASA. 16 (2).