Papers by Guillaume Dumas
Neurophysiologie Clinique/Clinical Neurophysiology
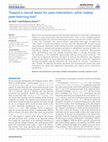
Many of the instructional practices that have been advanced as intrinsically motivating are inher... more Many of the instructional practices that have been advanced as intrinsically motivating are inherent in socio-constructivist learning environments. There is now emerging scientific evidence to explain why interactive learning environments promote the intrinsic motivation to learn. The "two-body" and "second person" approaches have begun to explore the "dark matter" of social neuroscience: the intra- and inter-individual brain dynamics during social interaction. Moreover, studies indicate that when young learners are given expanded opportunities to actively and equitably participate in collaborative learning activities they experienced feelings of well-being, contentment or even excitement. Neuroscience starts demonstrating how this naturally rewarding aspect is strongly associated with the implication of the mesolimbic dopaminergic pathway during social interaction. The production of dopamine reinforces the desire to continue the interaction, and height...
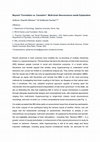
Trends in Cognitive Sciences, 2021
Recent advances in brain sciences have enabled the co-recording of multiple interacting brains (i... more Recent advances in brain sciences have enabled the co-recording of multiple interacting brains (i.e., hyperscanning [1]). This technique has led to the discovery of inter-brain synchrony (IBS) between people involved in social and interactive scenarios. In a recent article, Novembre and Iannetti argued that studies using hyperscanning to understand social behaviors are crucial but limited to correlational analysis [2]. They further developed the idea that the causal role of IBS can only be apprehended through multi-brain stimulation (MBS). Although we agree with Novembre and Iannetti that MBS is one of the most promising methods for investigating inter-brain coupling in the future, we disagree on their radical claim that it constitutes ‘the only validated empirical approach capable of teasing apart the mechanistic from the epiphenomenal interpretation of inter-brain synchrony’. In this Letter, we defend the idea that explaining IBS in terms of causal mechanisms is possible through adequate experimental designs and computational tools, with empirical approaches ranging from multi-brains (hyperscanning) to single-brain (classic social neuroscience) recordings, and even no-brain (i.e., in silico computational social neuroscience).
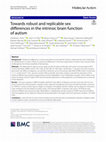
Molecular Autism, 2021
Background: Marked sex differences in autism prevalence accentuate the need to understand the rol... more Background: Marked sex differences in autism prevalence accentuate the need to understand the role of biological sex-related factors in autism. Efforts to unravel sex differences in the brain organization of autism have, however, been challenged by the limited availability of female data. Methods: We addressed this gap by using a large sample of males and females with autism and neurotypical (NT) control individuals (ABIDE; Autism: 362 males, 82 females; NT: 409 males, 166 females; 7-18 years). Discovery analyses examined main effects of diagnosis, sex and their interaction across five resting-state fMRI (R-fMRI) metrics (voxel-level Z > 3.1, cluster-level P < 0.01, gaussian random field corrected). Secondary analyses assessed the robustness of the results to different pre-processing approaches and their replicability in two independent samples: the EU-AIMS Longitudinal European Autism Project (LEAP) and the Gender Explorations of Neurogenetics and Development to Advance Autism Research. Results: Discovery analyses in ABIDE revealed significant main effects of diagnosis and sex across the intrinsic functional connectivity of the posterior cingulate cortex, regional homogeneity and voxel-mirrored homotopic connectiv-ity (VMHC) in several cortical regions, largely converging in the default network midline. Sex-by-diagnosis interactions were confined to the dorsolateral occipital cortex, with reduced VMHC in females with autism. All findings were robust to different pre-processing steps. Replicability in independent samples varied by R-fMRI measures and effects with the targeted sex-by-diagnosis interaction being replicated in the larger of the two replication samples-EU-AIMS LEAP. Limitations: Given the lack of a priori harmonization among the discovery and replication datasets available to date, sample-related variation remained and may have affected replicability.
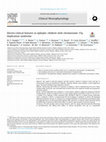
Clinical Neurophysiology, 2021
Objective:We aimed to describe epilepsy and EEG patterns related to vigilance states and age, inc... more Objective:We aimed to describe epilepsy and EEG patterns related to vigilance states and age, inchromosome15-long-arm-duplication-syndrome (dup15q) children with epilepsy, in both duplicationtypes: interstitial (intdup15) and isodicentric (idic15).Methods:Clinical data and 70 EEGs of 12 patients (5 intdup15, 7 idic15), followed from 4.5 m.o to 17y4m(median follow-up 8y3m), were retrospectively reviewed. EEGs were analyzed visually and using powerspectrum analysis.Results:Seventy video-EEGs were analyzed (1–16 per patient, median 6), follow-up lasting up to 8y10m(median 4y2m): 25 EEGs in intdup15 (8 m.o to 12y.o, median 4y6m) and 45 EEGs in idic15 (7 m.o to 12 y.o, median 15 m). Epilepsy: 6 West syndrome (WS) (2intdup15, 4idic15); 4 Lennox-Gastaut syndromes(LGS) (1 intdup15, 3 idic15), 2 evolving from WS; focal epilepsy (3 intdup15). In idic15, WS displayedadditional myoclonic seizures (3), atypical (4) or no hypsarrhythmia (2) and posterior predominant spikeand polyspike bursts (4). Beta-band rapid-rhythms (RR): present in 11 patients, power decreased duringnon-REM-sleep, localization shifted from diffuse to anterior, peak frequency increased with age.Conclusion:WS with peculiar electro-clinical features and LGS, along with beta-band RR decreasingin non-REM-sleep and shifting from diffuse to anterior localization with age are recognizable.
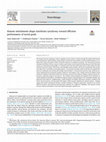
Neuroimage, 2021
The human brain has undergone massive expansion across primate evolution through life amidst mult... more The human brain has undergone massive expansion across primate evolution through life amidst multi-layered social attachments; within families, among friends, and between clan members and this enabled humans to coordinate their brains with those of others toward the execution of complex social goals. We examined how human attachments facilitate efficient, resource-sensitive performance of social goals by balancing neural and behavioral synchrony. Using hyperscanning EEG, we collected neural data from male-female pairs in three groups (N = 158, 79 pairs); long-term couples, best friends, and unfamiliar group members, during two ecologically-valid natural-istic tasks; motor coordination and empathy giving. Across groups and tasks, neural synchrony was supported by behavior coordination and orchestrated multiple neural rhythms. In the goal-directed motor task, interbrain syn-chrony implicated beta and gamma rhythms localized to sensorimotor areas. Couples showed the highest neural synchrony combined with greatest behavioral synchrony and such brain-behavior linkage resulted in speedy performance , conserving energy in the long run. The socially-oriented empathy task triggered neural synchrony in widely-distributed sensorimotor and bilateral temporal regions, integrated alpha, beta, and gamma rhythms, and implicated brain-behavior complementarity ; couples displayed the highest behavioral synchrony combined with lowest neural synchrony toward greatest felt support while strangers exhibited the opposite pattern. Findings suggest that human attachments provide a familiar backdrop of temporal regularities, required for the brain's allostatic function, and interbrain and behavioral synchrony are sculpted by familiarity and closeness toward resource-sensitive performance of survival-related social goals, toiled by two.
Molecular autism, 2020
Background: Heterogeneity in the phenotypic presentation of autism spectrum disorder (ASD) is app... more Background: Heterogeneity in the phenotypic presentation of autism spectrum disorder (ASD) is apparent in the profile and the severity of sensory features. Here, we applied factor mixture modelling (FMM) to test a multidimensional factor model of sensory processing in ASD. We aimed to identify homogeneous sensory subgroups in ASD that differ intrinsically in their severity along continuous factor scores. We also investigated sensory subgroups in relation to clinical variables: sex, age, IQ, social-communication symptoms, restricted and repetitive behaviours, adaptive functioning and symptoms of anxiety and attention-deficit/hyperactivity disorder.
Journal of Pineal Research, 2021
The human pineal gland regulates day-night dynamics of multiple physiological processes... more The human pineal gland regulates day-night dynamics of multiple physiological processes, especially through the secretion of melatonin. Using mass-spectrometry-based proteomics and dedicated analysis tools, we identify proteins in the human pineal gland and analyze systematically their variation throughout the day and com-pare these changes in the pineal proteome between control specimens and donors diagnosed with autism. Results reveal diverse regulated clusters of proteins with, among others, catabolic carbohydrate process and cytoplasmic membrane-bounded vesicle-related proteins differing between day and night and/or control versus autism pineal glands. These data show novel and unexpected processes happening in the human pineal gland during the day/night rhythm as well as specific differences be-tween autism donor pineal glands and those from controls.
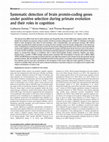
Genome research, 2021
The human brain differs from that of other primates, but the genetic basis of these differences r... more The human brain differs from that of other primates, but the genetic basis of these differences remains unclear. We investigated the evolutionary pressures acting on almost all human protein-coding genes (N = 11,667; 1:1 orthologs in primates) based on their divergence from those of early hominins, such as Neanderthals, and non-human primates. We confirm that genes encoding brain-related proteins are among the most strongly conserved protein-coding genes in the human ge-nome. Combining our evolutionary pressure metrics for the protein-coding genome with recent data sets, we found that this conservation applied to genes functionally associated with the synapse and expressed in brain structures such as the prefron-tal cortex and the cerebellum. Conversely, several genes presenting signatures commonly associated with positive selection appear as causing brain diseases or conditions, such as micro/macrocephaly, Joubert syndrome, dyslexia, and autism. Among those, a number of DNA damage response genes associated with microcephaly in humans such as BRCA1, NHEJ1, TOP3A, and RNF168 show strong signs of positive selection and might have played a role in human brain size expansion during primate evolution. We also showed that cerebellum granule neurons express a set of genes also presenting signatures of positive selection and that may have contributed to the emergence of fine motor skills and social cognition in humans. This resource is available online and can be used to estimate evolutionary constraints acting on a set of genes and to explore their relative contributions to human traits.
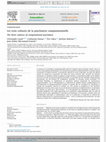
Annales Médico-psychologiques, 2021
Introduction
Whether on the social, economic or scientific level, the digital sciences tend to ch... more Introduction
Whether on the social, economic or scientific level, the digital sciences tend to change the conception of health. Computational Psychiatry, in the sense of a psychiatry based on “numbers” and information flow, has evolved rapidly.
Methods
In this article, we propose the distinction between three fields of Computational Psychiatry. A first field corresponds to “Digital Psychiatry”, i.e. a field using digital, connected, tools in the main goal to collect digital data (especially important in this period of COVID-19). A second field corresponds to “Big Psychiatry”, or Big Data Psychiatry, which deals with large amounts of data, e.g. through recent methodologies in Machine learning or artificial intelligence. A third field corresponds to “Psychiatry Modeling”, which corresponds to the utilization of formal hypothesis (i.e. mathematical models) about brain and behavior (and their dysfunctions) in line with computational neurosciences.
Results
The collection of digital data fits into methodologies of assessments and interventions in daily life, named Ecological Momentary Assessment. Of course, these digital data, which differ quantitatively and qualitatively from what psychiatry has been able to collect in its history, raise numerous epistemological and ethical questions. In the field of Big Psychiatry, most Machine learning techniques provide predictions rather than pathophysiological mechanisms, and these Machine learning techniques makes it possible to propose new delineations of disorders in a logic of stratified medicine. Lastly, resulting from studies in computational neurosciences, explanatory modeling of the brain (often called “Generative modeling”) proposes a number of theories to understand the functioning of the brain in psychiatric disorder (e.g. predictive coding, reinforcement learning, decision making theories, but also dynamical systems theories and graph and network theory).
Discussion and conclusion
This field could offer a framework to characterize the origin of the psychiatric symptoms. Obviously, these three fields are highly mutually dependent, with for instance a data access provided by Digital Psychiatry (with Digital Tools), a data processing operated by Big Psychiatry (with Machine learning) and a formalization of hypotheses offered by Generative modeling of the brain from Psychiatry Modeling. This triple organization of Computational Psychiatry offers a robust framework for personalized and precision psychiatry, articulated around statistical and mathematical methodologies, focused on prediction and explanation and using qualitatively and quantitatively varied data. However, such a framework is necessarily geared to a common subject: the patient of the psychiatric clinic.
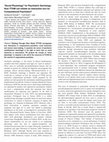
Behavioral and Brain Sciences, 2020
Thinking Through Other Minds (TTOM) encompasses new dimensions in computational psychiatry: socia... more Thinking Through Other Minds (TTOM) encompasses new dimensions in computational psychiatry: social interaction and mutual sense-making. It questions the nature of psychiatric manifestations (semiology) in the light of recent data on social interaction in neuroscience. We propose the concept of "social physiology" in response to the call by the conceivers of TTOM for the renewal of computational psychiatry. Psychiatric semiology, i.e. the science of clinical manifestations, considers that both symptoms and signs are "units of analysis". These units are actionable psychopathological features that are essential in practice for making the diagnosis and prognosis that underpin clinical decision-making (Micoulaud-Franchi et al., 2018). Psychiatric semiology is therefore very important. We would like to comment on how the concept of TTOM and the associated computational psychiatry model proposed by the authors not only questions the mechanisms underlying psychiatric manifestations (see p63-64, Veissière, Constant, Ramstead, Friston, & Kirmayer, 2019) but also the ways in which these manifestations are expressed by patients and captured by psychiatrists. Indeed, we need a computational model that questions how clinical manifestations are expressed and captured. In
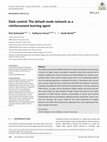
Human Brain Mapping, 2020
The default mode network (DMN) is believed to subserve the baseline mental activity in humans. It... more The default mode network (DMN) is believed to subserve the baseline mental activity in humans. Its higher energy consumption compared to other brain networks and its intimate coupling with conscious awareness are both pointing to an unknown over-arching function. Many research streams speak in favor of an evolutionarily adaptive role in envisioning experience to anticipate the future. In the present work, we propose a process model that tries to explain how the DMN may implement continuous evaluation and prediction of the environment to guide behavior. The main purpose of DMN activity, we argue, may be described by Markov decision processes that optimize action policies via value estimates through vicarious trial and error. Our formal perspective on DMN function naturally accommodates as special cases previous interpretations based on (a) predictive coding, (b) semantic associations, and (c) a sen-tinel role. Moreover, this process model for the neural optimization of complex behavior in the DMN offers parsimonious explanations for recent experimental findings in animals and humans. K E Y W O R D S artificial intelligence, human intelligence, systems neuroscience

Psychological Medicine, 2020
We agree with Georg Starke et al. (Starke et al. 2020): despite the current lack of direct clinic... more We agree with Georg Starke et al. (Starke et al. 2020): despite the current lack of direct clinical applications, artificial intelligence (AI) will undeniably transform the future of psychiatry. AI has led to algorithms that can perform more and more complex tasks by interpreting and learning from data (Dobrev 2012). AI applications in psychiatry are receiving more attention, with a 3-fold increase in the number of PubMed / MEDLINE articles on IA in psychiatry over the past three years (N=567 results). The impact of AI on the entire psychiatric profession is likely to be significant (Torous et al. 2015; Huys, Maia, and Frank 2016; Grisanzio et al. 2018; Brown et al. 2019). These effects will be felt not only through the advent of advanced applications in brain imaging (Starke et al. 2020) but also through the stratification and refinement of our clinical categories, a more profound challenge which “lies in its long-embattled nosology” (Kendler 2016).
These technical challenges are subsumed by ethical ones. In particular, the risk of non-transparency and reductionism in psychiatric practice is a burning issue. Clinical medicine has already developed the overarching ethical principles of respect for autonomy, non-maleficence, beneficence, and justice (Beauchamp and Childress 2001). The need for the principle of Explainability should be added to this list, specifically regarding the issues involved by AI (Floridi et al. 2018). Explainability concerns the understanding of how a given algorithm works (Intelligibility) and who is responsible for the way it works (Accountability). We totally agree with Starke et al. (2020) that Explainability is essential and constitutes a real challenge for future developments in AI. In addition, however, we think that this ethical issue requires dedicated pedagogical training that must be underpinned by a solid epistemological framework.
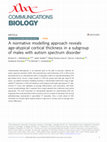
Nature Communications Biology, 2020
Understanding heterogeneity is an important goal on the path to precision medicine for autism spe... more Understanding heterogeneity is an important goal on the path to precision medicine for autism spectrum disorders (ASD). We examined how cortical thickness (CT) in ASD can be parameterized as an individualized metric of atypicality relative to typically-developing (TD) age-related norms. Across a large sample (n = 870 per group) and wide age range (5-40 years), we applied normative modelling resulting in individualized whole-brain maps of age-related CT atypicality in ASD and isolating a small subgroup with highly age-atypical CT. Age-normed CT scores also highlights on-average differentiation, and associations with beha-vioural symptomatology that is separate from insights gleaned from traditional case-control approaches. This work showcases an individualized approach for understanding ASD het-erogeneity that could potentially further prioritize work on a subset of individuals with cortical pathophysiology represented in age-related CT atypicality. Only a small subset of ASD individuals are actually highly atypical relative to age-norms. driving small on-average case-control differences.
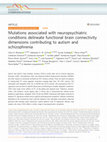
Nature Communications, 2020
,13,15 ✉ 16p11.2 and 22q11.2 Copy Number Variants (CNVs) confer high risk for Autism Spectrum Dis... more ,13,15 ✉ 16p11.2 and 22q11.2 Copy Number Variants (CNVs) confer high risk for Autism Spectrum Disorder (ASD), schizophrenia (SZ), and Attention-Deficit-Hyperactivity-Disorder (ADHD), but their impact on functional connectivity (FC) remains unclear. Here we report an analysis of resting-state FC using magnetic resonance imaging data from 101 CNV carriers, 755 individuals with idiopathic ASD, SZ, or ADHD and 1,072 controls. We characterize CNV FC-signatures and use them to identify dimensions contributing to complex idiopathic conditions. CNVs have large mirror effects on FC at the global and regional level. Thalamus, somato-motor, and posterior insula regions play a critical role in dysconnectivity shared across deletions, duplications, idiopathic ASD, SZ but not ADHD. Individuals with higher similarity to deletion FC-signatures exhibit worse cognitive and behavioral symptoms. Deletion similarities identified at the connectivity level could be related to the redundant associations observed genome-wide between gene expression spatial patterns and FC-signatures. Results may explain why many CNVs affect a similar range of neuropsychiatric symptoms.
Molecular Autism, 2020
Background: Heterogeneity in the phenotypic presentation of autism spectrum disorder (ASD) is app... more Background: Heterogeneity in the phenotypic presentation of autism spectrum disorder (ASD) is apparent in the profile and the severity of sensory features. Here, we applied factor mixture modelling (FMM) to test a multidimensional factor model of sensory processing in ASD. We aimed to identify homogeneous sensory subgroups in ASD that differ intrinsically in their severity along continuous factor scores. We also investigated sensory subgroups in relation to clinical variables: sex, age, IQ, social-communication symptoms, restricted and repetitive behaviours, adaptive functioning and symptoms of anxiety and attention-deficit/hyperactivity disorder.
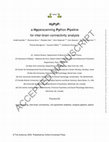
Social Cognitive and Affective Neuroscience, 2020
The bulk of social neuroscience takes a ‘stimulus-brain’ approach, typically comparing brain resp... more The bulk of social neuroscience takes a ‘stimulus-brain’ approach, typically comparing brain responses to different types of social stimuli, but most of the time in the absence of direct social interaction. Over the last two decades, a growing number of researchers have adopted a ‘brain-to-brain’ approach, exploring similarities between brain patterns across participants as a novel way to gain insight into the social brain. This methodological shift has facilitated the introduction of naturalistic social stimuli into the study design (e.g., movies), and, crucially, has spurred the development of new tools to directly study social interaction, both in controlled experimental settings and in more ecologically valid environments. Specifically, hyperscanning setups, which allow the simultaneous recording of brain activity from two or more individuals during social tasks has gained popularity in recent years. However, currently there is no agreed-upon approach to carry out such inter-brain connectivity analysis, resulting in a scattered landscape of analysis techniques. To accommodate a growing demand to standardize analysis approaches in this fast-growing research field, we have developed HyPyP, a comprehensive and easy open-source software package that allows (social) neuroscientists to carry-out and to interpret inter-brain connectivity analyses.
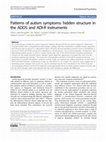
Translational Psychiatry, 2020
We simultaneously revisited the Autism Diagnostic Interview-Revised (ADI-R) and Autism Diagnostic... more We simultaneously revisited the Autism Diagnostic Interview-Revised (ADI-R) and Autism Diagnostic Observation Schedule (ADOS) with a comprehensive data-analytics strategy. Here, the combination of pattern-analysis algorithms and extensive data resources (n = 266 patients aged 7-49 years) allowed identifying coherent clinical constellations in and across ADI-R and ADOS assessments widespread in clinical practice. Our clustering approach revealed low-and high-severity patient groups, as well as a group scoring high only in the ADI-R domains, providing quantitative contours for the widely assumed autism subtypes. Sparse regression approaches uncovered the most clinically predictive questionnaire domains. The social and communication domains of the ADI-R showed convincing performance to predict the patients' symptom severity. Finally, we explored the relative importance of each of the ADI-R and ADOS domains conditioning on age, sex, and fluid IQ in our sample. The collective results suggest that (i) identifying autism subtypes and severity for a given individual may be most manifested in the ADI-R social and communication domains, (ii) the ADI-R might be a more appropriate tool to accurately capture symptom severity, and (iii) the ADOS domains were more relevant than the ADI-R domains to capture sex differences.

Médecine du Sommeil, 2020
The denomination of “personalized medicine” has been used in Sleep Medicine, especially in the co... more The denomination of “personalized medicine” has been used in Sleep Medicine, especially in the context of obstructive sleep apnea syndrome (OSAS). It refers to an “umbrella term”, which seems insufficient to conceptually define the extent of the field. Regarding the convergence between personalized medicine and Sleep Medicine, this article proposes a clarification to the concepts around personalized medicine by proposing a distinction between precision medicine and stratified medicine in OSAS. Precision medicine seeks to integrate molecular and biological research with the clinical data of each patient to develop a more precise “molecular taxonomy” of diseases by identifying endotypes related to biomarkers. The reference author in this field of OSAS is Eckert. Indeed, Eckert highlighted three potentials “endotypes” of OSAS (impairment in pharyngeal dilator muscle control and function during sleep; increased propensity for awakening during airway narrowing with low respiratory arousal threshold; respiratory control instability with high loop gain). Stratified medicine seeks to integrate research on big data to develop clusters in order to define new phenotypes by identifying sets of indicators. Especially, phenotyping allows us to set up an optimized prevention and therapy due to an appropriate adaptation to the specific characteristics of a subgroup. Thus, phenotyping improves diagnostic, prognostic and follow-up strategies. The reference author in this field is Zinchuk who does not use the term stratified medicine (but use rather precision medicine). This author highlighted six potentials “phenotypes” of OSAS (called subtypes A to F). The terminology used in this article is not intended to be definitive, but offers a framework for clarifying the concepts involved, the research programs initiated and the representations of researchers. The ambitions of a robust research program for OSAS would require a pairing of the precision and stratification approaches, by building an integrative framework for the future of Sleep Medicine. This program allows us to define phenotypes related to mechanically explanatory endotypes, and to define endotypes related to clinically useful phenotypes.
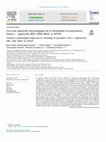
AMPSY, 2019
This first article, in a series of two, on the physiological approach of psychiatric semiology pr... more This first article, in a series of two, on the physiological approach of psychiatric semiology proposes to explore the place of physiology and clinical semiology in three systems of psychiatric classifications currently proposed in research: the Diagnostic and Statistical Manual of Mental Disorders (DSM), the Research Domain Criteria Project (RDoC), and the Hierarchical Taxonomy of Psychopathology project (HiTOP). For each of these systems will be presented the general principle, the link with physiology and semiology, and the main limitations. Physiology and clinical manifestation occupy a variable place and role in these three systems of psychiatric classifications. The DSM proposes a list of semiological criteria that are relevant to the clinical history of psychiatry, but the choice of these criteria and their organization remains guided by purely practical and nosographic issues with a very limited place for physiology. The RDoC project does not propose a list of semiological criteria. Its clinical and practical relevance is clearly questionable, but this project has the merit of emphasizing the importance of physiology in the medical approach of mental disorders. The HiTOP project does not in itself propose a list of semiological criteria, but proposes a hierarchical organizational framework of the nosography allowing to associate a set of signs and symptoms with supposed underlying psychopathological mechanisms. The challenge remains to coherently hang these psychopathological mechanisms to physiopathological mechanisms of mental disorders according to an integrative perspective of mental disorders.
Uploads
Papers by Guillaume Dumas
Whether on the social, economic or scientific level, the digital sciences tend to change the conception of health. Computational Psychiatry, in the sense of a psychiatry based on “numbers” and information flow, has evolved rapidly.
Methods
In this article, we propose the distinction between three fields of Computational Psychiatry. A first field corresponds to “Digital Psychiatry”, i.e. a field using digital, connected, tools in the main goal to collect digital data (especially important in this period of COVID-19). A second field corresponds to “Big Psychiatry”, or Big Data Psychiatry, which deals with large amounts of data, e.g. through recent methodologies in Machine learning or artificial intelligence. A third field corresponds to “Psychiatry Modeling”, which corresponds to the utilization of formal hypothesis (i.e. mathematical models) about brain and behavior (and their dysfunctions) in line with computational neurosciences.
Results
The collection of digital data fits into methodologies of assessments and interventions in daily life, named Ecological Momentary Assessment. Of course, these digital data, which differ quantitatively and qualitatively from what psychiatry has been able to collect in its history, raise numerous epistemological and ethical questions. In the field of Big Psychiatry, most Machine learning techniques provide predictions rather than pathophysiological mechanisms, and these Machine learning techniques makes it possible to propose new delineations of disorders in a logic of stratified medicine. Lastly, resulting from studies in computational neurosciences, explanatory modeling of the brain (often called “Generative modeling”) proposes a number of theories to understand the functioning of the brain in psychiatric disorder (e.g. predictive coding, reinforcement learning, decision making theories, but also dynamical systems theories and graph and network theory).
Discussion and conclusion
This field could offer a framework to characterize the origin of the psychiatric symptoms. Obviously, these three fields are highly mutually dependent, with for instance a data access provided by Digital Psychiatry (with Digital Tools), a data processing operated by Big Psychiatry (with Machine learning) and a formalization of hypotheses offered by Generative modeling of the brain from Psychiatry Modeling. This triple organization of Computational Psychiatry offers a robust framework for personalized and precision psychiatry, articulated around statistical and mathematical methodologies, focused on prediction and explanation and using qualitatively and quantitatively varied data. However, such a framework is necessarily geared to a common subject: the patient of the psychiatric clinic.
These technical challenges are subsumed by ethical ones. In particular, the risk of non-transparency and reductionism in psychiatric practice is a burning issue. Clinical medicine has already developed the overarching ethical principles of respect for autonomy, non-maleficence, beneficence, and justice (Beauchamp and Childress 2001). The need for the principle of Explainability should be added to this list, specifically regarding the issues involved by AI (Floridi et al. 2018). Explainability concerns the understanding of how a given algorithm works (Intelligibility) and who is responsible for the way it works (Accountability). We totally agree with Starke et al. (2020) that Explainability is essential and constitutes a real challenge for future developments in AI. In addition, however, we think that this ethical issue requires dedicated pedagogical training that must be underpinned by a solid epistemological framework.
Whether on the social, economic or scientific level, the digital sciences tend to change the conception of health. Computational Psychiatry, in the sense of a psychiatry based on “numbers” and information flow, has evolved rapidly.
Methods
In this article, we propose the distinction between three fields of Computational Psychiatry. A first field corresponds to “Digital Psychiatry”, i.e. a field using digital, connected, tools in the main goal to collect digital data (especially important in this period of COVID-19). A second field corresponds to “Big Psychiatry”, or Big Data Psychiatry, which deals with large amounts of data, e.g. through recent methodologies in Machine learning or artificial intelligence. A third field corresponds to “Psychiatry Modeling”, which corresponds to the utilization of formal hypothesis (i.e. mathematical models) about brain and behavior (and their dysfunctions) in line with computational neurosciences.
Results
The collection of digital data fits into methodologies of assessments and interventions in daily life, named Ecological Momentary Assessment. Of course, these digital data, which differ quantitatively and qualitatively from what psychiatry has been able to collect in its history, raise numerous epistemological and ethical questions. In the field of Big Psychiatry, most Machine learning techniques provide predictions rather than pathophysiological mechanisms, and these Machine learning techniques makes it possible to propose new delineations of disorders in a logic of stratified medicine. Lastly, resulting from studies in computational neurosciences, explanatory modeling of the brain (often called “Generative modeling”) proposes a number of theories to understand the functioning of the brain in psychiatric disorder (e.g. predictive coding, reinforcement learning, decision making theories, but also dynamical systems theories and graph and network theory).
Discussion and conclusion
This field could offer a framework to characterize the origin of the psychiatric symptoms. Obviously, these three fields are highly mutually dependent, with for instance a data access provided by Digital Psychiatry (with Digital Tools), a data processing operated by Big Psychiatry (with Machine learning) and a formalization of hypotheses offered by Generative modeling of the brain from Psychiatry Modeling. This triple organization of Computational Psychiatry offers a robust framework for personalized and precision psychiatry, articulated around statistical and mathematical methodologies, focused on prediction and explanation and using qualitatively and quantitatively varied data. However, such a framework is necessarily geared to a common subject: the patient of the psychiatric clinic.
These technical challenges are subsumed by ethical ones. In particular, the risk of non-transparency and reductionism in psychiatric practice is a burning issue. Clinical medicine has already developed the overarching ethical principles of respect for autonomy, non-maleficence, beneficence, and justice (Beauchamp and Childress 2001). The need for the principle of Explainability should be added to this list, specifically regarding the issues involved by AI (Floridi et al. 2018). Explainability concerns the understanding of how a given algorithm works (Intelligibility) and who is responsible for the way it works (Accountability). We totally agree with Starke et al. (2020) that Explainability is essential and constitutes a real challenge for future developments in AI. In addition, however, we think that this ethical issue requires dedicated pedagogical training that must be underpinned by a solid epistemological framework.
References
DUMAS, G., MARTINERIE, J., SOUSSIGNAN, R., & NADEL, J. (2012). Does the brain know who is at the origin of what in an imitative interaction? Frontiers in Human Neuroscience. Special issue: Towards a neuroscience of social interaction, doi: 103389/fnhum.2012.00128.
DUMAS, G., NADEL, J., SOUSSIGNAN, R., MARTINERIE, J., & GARNERO, L. (2010). Inter-brain synchronizations during social interaction. PlosOne. , 5: doi:10.1371/journal.pone.0012166.
GUIONNET, S., NADEL, J., DELAVEAU, P., SPERDUTI, M., & FOSSATI, P. (2011). Reciprocal imitation: Towards a neural basis of social interaction. Cerebral Cortex, DOI 10 1093 cercor/bhr177
NADEL, J. (2014). How imitation boots development in infancy and Autism Spectrum disorder. Oxford: Oxford University Press
Collective cognition: The Neurophysiology of Social Neuroscience
The HackYourPhD community was born out of an acknowledgement that current ways of performing research frequently generate frustration, conflicts, and isolation. The crisis in research is sometimes covered in the media: job insecurity, rush to publication creating pressure and dishonest practices, privatization of knowledge through the grip of scientific publishing houses. This is a vision from the inside – that of research practitioners.
This picture may appear rather negative to society at large, which often does not understand how research works. Research is a “black box” for the majority of the population. There is a divide. Citizens often hold researchers in high esteem, but the crisis of trust is deepening, because of the lack of dialogue. However, there is hope: today’s knowledge society makes the emergence of citizen researchers both desirable and possible.
HackYourPhD brings together students, researchers, engaged citizens, hacktivists, tinkerers from all horizons, entrepreneurs, and everyone who is interested in the production and the sharing of knowledge in the wider sense. This collective aims to bring concrete solutions to complex issues and to build much-needed collaborative relationships between those involved in knowledge production. This is required for collective intelligence to come into existence and bring answers to urgent issues of society.
We wish, through common activities carried out by members of the community, to create healthy dynamics among researchers and to open the “black box” so that research may serve society rather than entrenched interests.
*******
In this article, the authors present the main issues that need to be addressed in order to renew and redefine the traditional approach to Altered States of Consciousness (ASCs). After a brief introduction shedding light on the genesis of this special issue and the rationale for the study of ASCs, attention is given to some conceptual clarifications required in the study of ASCs. The authors suggest that the alteration of consciousness can take place in four distinct ways: the alteration may concern the content, the quality, the modality and the level of consciousness. Next, several problems and methodological challenges posed by the scientific and naturalistic study of ASC are examined. Granted the inherent complexity of ASCs, the authors underscore the importance of integrative – transdisciplinary – and multidimensional approaches. All the articles included in the thematic issue are finally introduced and put into context.