Papers by Mustafa Ustuner
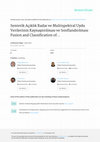
In this study, synthetic aperture radar (SAR) and multispectral data are fused with different met... more In this study, synthetic aperture radar (SAR) and multispectral data are fused with different methods in order to observe the effect of fusion methods on the accuracy of different classification techniques. At the same time, different polarizations of SAR data are included in fusion process and results are examined. The fusion methods that are used in this study are Brovey Color Normalized, Hue Saturation Value (HSV), Gram – Schmidt (GS) Spectral Sharpening and Principal Components (PC) Spectral Sharpening. Fused images are classified using k-nearest neighbor, support vector machine and radial based function neural network. The study area is chosen on Menemen Plain, which contains agricultural lands, and it is located in İzmir. Multispectral RapidEye satellite image and TerraSAR-X radar data are used for the analysis. Achieved results were presented in the tables. The highest accuracy is achieved by K-NN classification of TerraSAR-X and VH fusion with GS method as 95.74%.
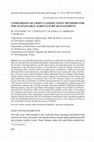
Accurate and reliable information regarding crop yields and soil conditions of agricultural field... more Accurate and reliable information regarding crop yields and soil conditions of agricultural fields are essential for the sustainable management of agricultural areas. The increasing necessity of the food due to the high population, global climate change and rapid urbanisation, the sustainable management of the agricultural resources is becoming more crucial for countries. Remote sensing technology offers a feasible solution for gathering the cost-effective, reliable and up-to-date information about crop monitoring by using high-resolution remote sensing data. Image classification is the one of most common method to obtain information from the remotely sensed images. Despite machine learning based classifiers such as Support Vector Machines (SVM) could provide high classification accuracy, the researchers have been still working to improve the classification accuracy. Recently, the utilisation of ensemble learning approaches in remote sensing classification is the research of interest for this purpose. In this study, we implemented six different supervised classification techniques and a classifier ensemble: Maximum Likelihood, Mahalanobis Distance, Minimum Distance, Spectral Angle Mapper, Parallelepiped, Support Vector Machines and Winner-takes-all (WTA) classification which is an ensemble based classifier. In this study, we investigated the comparative performance of the classifiers within overall and corn-class category for the study area located in Aydin, Turkey. Radial Basis Function (RBF) kernel was used here for the SVM classification. Results demonstrate that WTA classification outperformed other classification methods whilst the Parallelepiped obtained the lowest classification accuracy 13.24%. Moreover SVM gave the second highest overall classification accuracy of 89.90%.
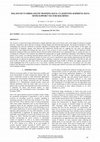
The accuracy of supervised image classification is highly dependent upon several factors such as ... more The accuracy of supervised image classification is highly dependent upon several factors such as the design of training set (sample selection, composition, purity and size), resolution of input imagery and landscape heterogeneity. The design of training set is still a challenging issue since the sensitivity of classifier algorithm at learning stage is different for the same dataset. In this paper, the classification of RapidEye imagery with balanced and imbalanced training data for mapping the crop types was addressed. Classification with imbalanced training data may result in low accuracy in some scenarios. Support Vector Machines (SVM), Maximum Likelihood (ML) and Artificial Neural Network (ANN) classifications were implemented here to classify the data. For evaluating the influence of the balanced and imbalanced training data on image classification algorithms, three different training datasets were created. Two different balanced datasets which have 70 and 100 pixels for each class of interest and one imbalanced dataset in which each class has different number of pixels were used in classification stage. Results demonstrate that ML and NN classifications are affected by imbalanced training data in resulting a reduction in accuracy (from 90.94% to 85.94% for ML and from 91.56% to 88.44% for NN) while SVM is not affected significantly (from 94.38% to 94.69%) and slightly improved. Our results highlighted that SVM is proven to be a very robust, consistent and effective classifier as it can perform very well under balanced and imbalanced training data situations. Furthermore, the training stage should be precisely and carefully designed for the need of adopted classifier.
Citation: Ustuner, M., Sanli, F. B., and Abdikan, S.: BALANCED VS IMBALANCED TRAINING DATA: CLASSIFYING RAPIDEYE DATA WITH SUPPORT VECTOR MACHINES, Int. Arch. Photogramm. Remote Sens. Spatial Inf. Sci., XLI-B7, 379-384, doi:10.5194/isprs-archives-XLI-B7-379-2016, 2016.
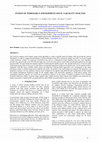
This research compares and evaluates image fusion algorithms to achieve spatially improved images... more This research compares and evaluates image fusion algorithms to achieve spatially improved images while preserving the spectral information. In order to compare the performance of fusion techniques both active and passive images were used. As an active image a high resolution, X-band, VV polarized TerraSAR-X data and as a multispectral image RapidEye data were used. RapidEye provides five optical bands in the 400-850 nm range and it is the first space-borne sensor which operationally gathers the red edge spectrum (690-730 nm) besides the standard channels of multi-spectral satellite sensors. The selected study area is in the low lands of Menemen (Izmir) Plain on the west of Gediz Basin covering both agricultural fields and residential areas. For the quality analysis, Adjustable SAR-MS Fusion (ASMF), Ehlers fusion and High Pass Filtering (HPF) approaches were investigated. In this study preliminary results of selected image fusion methods were given. The quality of the fused images was assessed with qualitative and quantitative analyses. For the qualitative analysis visual comparison was applied using different band combinations of fused image and original multispectral Rapid-Eye image. In the merged images color distortions regarding to SAR-optical synergy were investigated. Statistical analysis was carried out as quantitative analyses. In this respect Correlation Coefficient (CC), Standard Deviation Difference (SDD), Universal Image Quality Index (UIQI) and Root Mean Square Error (RMSE) were performed for quality assessments. In general HPF was performed best while ASMF was performed the worst in all results.
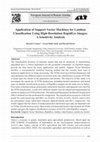
The classification accuracy of remotely sensed data and its sensitivity to classification algorit... more The classification accuracy of remotely sensed data and its sensitivity to classification algorithms have a critical importance for the geospatial community, as classified images provide the base layers for many applications and models. Support Vector Machines (SVMs), a non-parametric statistical learning method that has recently been used in numerous applications in image processing. The SVMs need user-defined parameters and each parameter has different impact on kernels hence the classification accuracy of SVMs is based upon the choice of the parameters and kernels. The objective of this study is to investigate the sensitivity of SVM architecture including internal parameters and kernel types on landuse classification accuracy of RapidEye imagery for the study area in Turkey. Four types of kernels (linear, polynomial, radial basis function, and sigmoid) were used for the SVM classification. A total of 63 different models were developed and implemented for sensitivity analysis of SVM architecture. The traditional Maximum Likelihood Classification (MLC) method was also performed for comparison. The classification accuracies of the best model for each kernel type and MLC are 85.63%, 83.94%, 83.94%, 83.82% and 81.64% for polynomial, linear, radial basis function, sigmoid kernels and MLC, respectively. The results suggest that the choice of model parameters and kernel types play an important role on SVMs classification accuracy. Best model of polynomial kernel outperformed all SVMs models and gave the highest classification accuracy of 85.63% with RapidEye imagery - See more at: http://www.aitjournal.com/articleView.aspx?ID=949#sthash.NVPVtKr3.dpuf
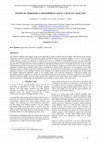
This research compares and evaluates image fusion algorithms to achieve spatially improved images... more This research compares and evaluates image fusion algorithms to achieve spatially improved images while preserving the spectral information. In order to compare the performance of fusion techniques both active and passive images were used. As an active image a high resolution, X-band, VV polarized TerraSAR-X data and as a multispectral image RapidEye data were used. RapidEye provides five optical bands in the 400-850 nm range and it is the first space-borne sensor which operationally gathers the red edge spectrum (690-730 nm) besides the standard channels of multi-spectral satellite sensors. The selected study area is in the low lands of Menemen (Izmir) Plain on the west of Gediz Basin covering both agricultural fields and residential areas. For the quality analysis, Adjustable SAR-MS Fusion (ASMF), Ehlers fusion and High Pass Filtering (HPF) approaches were investigated. In this study preliminary results of selected image fusion methods were given. The quality of the fused images was assessed with qualitative and quantitative analyses. For the qualitative analysis visual comparison was applied using different band combinations of fused image and original multispectral Rapid-Eye image. In the merged images color distortions regarding to SAR-optical synergy were investigated. Statistical analysis was carried out as quantitative analyses. In this respect Correlation Coefficient (CC), Standard Deviation Difference (SDD), Universal Image Quality Index (UIQI) and Root Mean Square Error (RMSE) were performed for quality assessments. In general HPF was performed best while ASMF was performed the worst in all results.
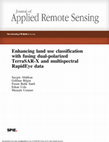
The contribution of dual-polarized synthetic aperture radar (SAR) to optical data for the accurac... more The contribution of dual-polarized synthetic aperture radar (SAR) to optical data for the accuracy of land use classification is investigated. For this purpose, different image fusion algorithms are implemented to achieve spatially improved images while preserving the spectral information. To compare the performance of the fusion techniques, both the microwave X-band dual-polarized TerraSAR-X data and the multispectral (MS) optical image RapidEye data are used. Our test site, Gediz Basin, covers both agricultural fields and artificial structures. Before the classification phase, four data fusion approaches: (1) adjustable SAR-MS fusion, (2) Ehlers fusion, (3) high-pass filtering, and (4) Bayesian data fusion are applied. The quality of the fused images was evaluated with statistical analyses. In this respect, several methods are performed for quality assessments. Then the classification performances of the fused images are also investigated using the support vector machines as a kernel-based method, the random forests as an ensemble learning method, the fundamental k-nearest neighbor, and the maximum likelihood classifier methods comparatively. Experiments provide promising results for the fusion of dual polarimetric SAR data and optical data in land use/cover mapping
Enhancing land use classification with fusing dual-polarized TerraSAR-X and multispectral RapidEye data. Available from: http://dx.doi.org/10.1117/1.JRS.9.096054
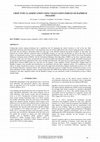
Cutting-edge remote sensing technology has a significant role for managing the natural resources ... more Cutting-edge remote sensing technology has a significant role for managing the natural resources as well as the any other applications about the earth observation. Crop monitoring is the one of these applications since remote sensing provides us accurate, up-to-date and cost-effective information about the crop types at the different temporal and spatial resolution. In this study, the potential use of three different vegetation indices of RapidEye imagery on crop type classification as well as the effect of each indices on classification accuracy were investigated. The Normalized Difference Vegetation Index (NDVI), the Green Normalized Difference Vegetation Index (GNDVI), and the Normalized Difference Red Edge Index (NDRE) are the three vegetation indices used in this study since all of these incorporated the near-infrared (NIR) band. RapidEye imagery is highly demanded and preferred for agricultural and forestry applications since it has red-edge and NIR bands. The study area is located in Aegean region of Turkey. Radial Basis Function (RBF) kernel was used here for the Support Vector Machines (SVMs) classification. Original bands of RapidEye imagery were excluded and classification was performed with only three vegetation indices. The contribution of each indices on image classification accuracy was also tested with single band classification. Highest classification accuracy of 87, 46%
was obtained using three vegetation indices. This obtained classification accuracy is higher than the classification accuracy of any dual-combination of these vegetation indices. Results demonstrate that NDRE has the highest contribution on classification accuracy compared to the other vegetation indices and the RapidEye imagery can get satisfactory results of classification accuracy without
original bands
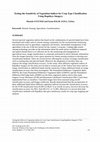
Several spectral vegetation indices that based on the combinations of spectral bands have been de... more Several spectral vegetation indices that based on the combinations of spectral bands have been developed and widely used in remote sensing community for the environmental monitoring and assessment such as agriculture, vegetation and forestry. Sustainable management of the agriculture is the one of the key factors for the country’s economy. Cutting-edge remote sensing technology provides us the information about the earth surface in different temporal and spatial domain hence is in efficient use for the monitoring and management of agricultural areas. Image classification is commonly performed to derive thematic information from the images therefore it has a great attention by remote sensing scientists to develop new classification methods. There are several factors affecting the accuracy of image classification such as training data and spectral bands. Moreover the integration of ancillary data can improve the image classification accuracy. In this study, three different vegetation indices of RapidEye imagery for the study area located in western part of the Turkey have been used on image classification as additional bands and the sensitivity of these additional bands on image classification accuracy has been investigated. The Normalized Difference Vegetation Index (NDVI), the Green Normalized Difference Vegetation Index (GNDVI), and the Normalized Difference Red Edge Index (NDRE) are the three vegetation indices used in this study. Support Vector Machines (SVM) and Maximum Likelihood Classification (MLC) have been implemented here as classifiers. Classified images have been assessed using overall classification accuracy and Kappa coefficient. Results demonstrated that the incorporation of these additional bands (three different vegetation indices) increase the classification accuracy for the SVM classification from 83,96% to 86,01 % as overall accuracy, however decrease the classification accuracy for MLC from 80,21% to 73,38%. Moreover it is proven than both classification methods with RapidEye data give satisfactory results for crop type mapping and analysis
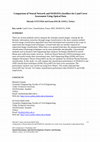
There are several methods used to interpret the remotely-sensed images .Among the all, thematic i... more There are several methods used to interpret the remotely-sensed images .Among the all, thematic information extraction through image classification is the most common method. Several image classification algorithms have been developed and examined in the scope of supervised and unsupervised techniques. Ground truth data are initially required for supervised image classification. When there is no ground truth data, the unsupervised image classification (clustering) techniques can be more preferable and plausible. Conventional methods such as Iterative Self Organizing Data Analysis Technique (ISODATA) and K-Means give sufficient results, however Artificial Neural Network (ANN) techniques have more successful results than conventional ones. Self Organizing Maps (SOM) and Fuzzy Adaptive Resonance Theory (FuzzyART) are the two methods for Artificial Neural Network classification. In this study, we will compare the classification performance of ISODATA and ANN classification techniques (SOM, FuzzyART) for land cover assessment. The performance analysis was carried out using Error Matrix. The results indicated that FuzzyART has %4 and %12 better classification performance than ISODATA and SOM, respectively.
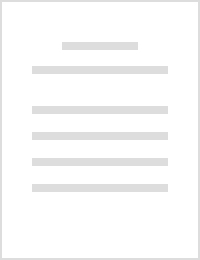
In this study, synthetic aperture radar (SAR) and multispectral data are fused with different met... more In this study, synthetic aperture radar (SAR) and multispectral data are fused with different methods in order to observe the effect of fusion methods on the accuracy of different classification techniques. At the same time, different polarizations of SAR data are included in fusion process and results are examined. The fusion methods that are used in this study are Brovey Color Normalized, Hue Saturation Value (HSV), Gram - Schmidt (GS) Spectral Sharpening and Principal Components (PC) Spectral Sharpening. Fused images are classified using k-nearest neighbor, support vector machine and radial based function neural network. The study area is chosen on Menemen Plain, which contains agricultural lands, and it is located in İzmir. Multispectral RapidEye satellite image and TerraSAR-X radar data are used for the analysis. Achieved results were presented in the tables. The highest accuracy is achieved by K-NN classification of TerraSAR-X and VH fusion with GS method as 95.74%.
Thesis Chapters by Mustafa Ustuner
Based on CLC (CORINE Land Cover) 2006 data, the land cover in Turkey consists of 42.35% agricultu... more Based on CLC (CORINE Land Cover) 2006 data, the land cover in Turkey consists of 42.35% agricultural lands and 54.04% forest and semi natural vegetation.Therefore, reliable and detailed information regarding LULC are crucially important for environmental decision makers and local authorities on strategic planning and sustainable land management.
Conference Presentations by Mustafa Ustuner
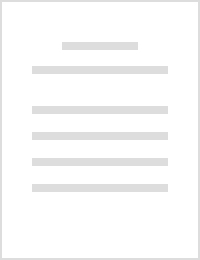
[English] In this study, Sentinel-1A SAR imagery for land use/cover classification and its impact... more [English] In this study, Sentinel-1A SAR imagery for land use/cover classification and its impacts on classification algorithms were addressed. Sentinel-1A imagery has dual polarization (VV and VH) and freely available from ESA. Istanbul was selected as the study region. After the pre-processing steps including the applying the precise orbit file, calibration, multilooking, speckle filtering and terrain correction, the imagery was classified as the following step. Three classification algorithms (SVM, RF and KNN) were implemented and the impacts of additional bands (VV-VH, VV+VH etc.) were investigated. Results demonstrated that highest classification accuracy of this study was obtained by SVM classification with the original bands (VV and VH) of Sentinel-1A imagery. Moreover, it was concluded that additional bands had different impacts on each classifier within accuracy. .................................................................................................................................................................................... [Türkçe] Bu çalışmada, Sentinel-1A SAR (Sentetik Açıklıklı Radar) görüntüsünün arazi örtüsü ve kullanımı sınıflandırmasında kullanılabilirliği ve sınıflandırma algoritmaları üzerindeki etkileri araştırılmıştır. İkili/çift polarizasyona (DD, DY; D:Düşey, Y:Yatay) sahip Sentinel-1A görüntüsü Avrupa Uzay Ajansı’ndan ücretsiz olarak elde edilmiştir. Çalışma alanı olarak İstanbul seçilmiştir. Elde edilen SAR görüntüsünün, yörünge düzeltmesi, veri kalibrasyonu, multilooking işlemi, benek (speckle) filtrelemesi ve arazi düzeltmesi (terrain correction) işlemlerinin ardından sınıflandırma işlemine geçilmiştir. Çalışma kapsamında üç farklı sınıflandırma tekniği (Destek Vektör Makineleri (DVM), Rastgele Orman(RO) ve K-En Yakın Komşuluk(K-EYK)) kullanılmış ve orijinal iki banttan oluşturulmuş diğer bantların (DD-DY, DD+DY vb.) etkisi incelenmiştir. Çalışmada en yüksek sınıflandırma doğruluğu (%81.90) orijinal iki bandın DVM yöntemi ile sınıflandırılması sonucu elde edilmiştir. Diğer bantların sınıflandırma doğruluğuna olan etkisinin seçilen algoritmaya göre farklılık gösterdiği gözlenmiştir.

Since the rapid population growth and climate change over the world, the need of the food and wat... more Since the rapid population growth and climate change over the world, the need of the food and water for human life have been increasing hence the sustainable management of agricultural resources has been becoming crucially important for increased agricultural production as well as food security measures. New earth observation satellites offers high spatial resolution with different variety of spectral features and fast revisit rates of the same location, and this provide an advantage to manage the natural resources. In this study, we examined the impacts of texture features of red-edge spectral band for classifying crops in Aydin Province, located in Turkey. The study area is located in Aegean region of Turkey and comprised of intensive cultivation areas. Different texture features (mean, variance, homogeneity, contrast, dissimilarity, entropy, second moment, and correlation) from Gray Level Co-occurrence Matrix (GLCM) using different window sizes have been computed. Support Vector Machines (SVM), is one of the machine learning algorithms and recently preferred since its superior image classification performance, has been carried out here for the classification step. Radial Basis Function (RBF) has been selected here as a kernel type for SVM classification. We also compared the classification results of texture features with original RapidEye imagery. Our experimental results indicate that the size of processing window play an important role on the classification accuracy of the red-edge band texture features and the classification accuracy of RapidEye original bands outperformed the others as 89.90% overall accuracy.

Information extraction through remote sensing data is important for policy and decision makers as... more Information extraction through remote sensing data is important for policy and decision makers as extracted information provide base layers for many application of real world. Classification of remotely sensed data is the one of the most common methods of extracting information however it is still a challenging issue because several factors are affecting the accuracy of the classification. Resolution of the imagery, number and homogeneity of land cover classes, purity of training data and characteristic of adopted classifiers are just some of these challenging factors. Object based image classification has some superiority than pixel based classification for high resolution images since it uses geometry and structure information besides spectral information. Vegetation indices are also commonly used for the classification process since it provides additional spectral information for vegetation, forestry and agricultural areas. In this study, the impacts of the Normalized Difference Vegetation Index (NDVI) and Normalized Difference Red Edge Index (NDRE) on the classification accuracy of RapidEye imagery were investigated. Object based Support Vector Machines were implemented for the classification of crop types for the study area located in Aegean region of Turkey. Results demonstrated that the incorporation of NDRE increase the classification accuracy from 79,96% to 86,80% as overall accuracy, however NDVI decrease the classification accuracy from 79,96% to 78,90%. Moreover it is proven than object based classification with RapidEye data give promising results for crop type mapping and analysis.
Uploads
Papers by Mustafa Ustuner
Citation: Ustuner, M., Sanli, F. B., and Abdikan, S.: BALANCED VS IMBALANCED TRAINING DATA: CLASSIFYING RAPIDEYE DATA WITH SUPPORT VECTOR MACHINES, Int. Arch. Photogramm. Remote Sens. Spatial Inf. Sci., XLI-B7, 379-384, doi:10.5194/isprs-archives-XLI-B7-379-2016, 2016.
Enhancing land use classification with fusing dual-polarized TerraSAR-X and multispectral RapidEye data. Available from: http://dx.doi.org/10.1117/1.JRS.9.096054
was obtained using three vegetation indices. This obtained classification accuracy is higher than the classification accuracy of any dual-combination of these vegetation indices. Results demonstrate that NDRE has the highest contribution on classification accuracy compared to the other vegetation indices and the RapidEye imagery can get satisfactory results of classification accuracy without
original bands
Thesis Chapters by Mustafa Ustuner
Conference Presentations by Mustafa Ustuner
Citation: Ustuner, M., Sanli, F. B., and Abdikan, S.: BALANCED VS IMBALANCED TRAINING DATA: CLASSIFYING RAPIDEYE DATA WITH SUPPORT VECTOR MACHINES, Int. Arch. Photogramm. Remote Sens. Spatial Inf. Sci., XLI-B7, 379-384, doi:10.5194/isprs-archives-XLI-B7-379-2016, 2016.
Enhancing land use classification with fusing dual-polarized TerraSAR-X and multispectral RapidEye data. Available from: http://dx.doi.org/10.1117/1.JRS.9.096054
was obtained using three vegetation indices. This obtained classification accuracy is higher than the classification accuracy of any dual-combination of these vegetation indices. Results demonstrate that NDRE has the highest contribution on classification accuracy compared to the other vegetation indices and the RapidEye imagery can get satisfactory results of classification accuracy without
original bands