articles by Connor T Jerzak
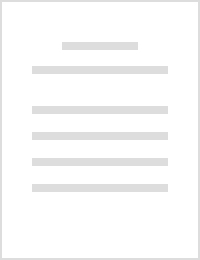
ArXiv Preprint
Earth Observation (EO) data are increasingly used in policy analysis by enabling granular es... more Earth Observation (EO) data are increasingly used in policy analysis by enabling granular estimation of treatment effects. However, a challenge in EO-based causal inference lies in balancing the trade-off between capturing fine-grained individual heterogeneity and broader contextual information. This paper introduces Multi-scale Concatenation, a family of composable procedures that transform arbitrary single-scale CATE estimation algorithms into multi-scale algorithms. We benchmark the performance of Multi-scale Concatenation on a CATE estimation pipeline combining Vision Transformer (ViT) models fine-tuned on satellite images to encode images of different scales with Causal Forests to obtain the final CATE estimate. We first perform simulation studies, showing how a multi-scale approach captures multi-level dynamics that single-scale ViT models fail to capture. We then apply the multi-scale method to two randomized controlled trials (RCTs) conducted in Peru and Uganda using Landsat satellite imagery. In the RCT analysis, the Rank Average Treatment Effect Ratio (RATE Ratio) measure is employed to assess performance without ground truth individual treatment effects. Results indicate that Multi-scale Concatenation improves the performance of deep learning models in EO-based CATE estimation without the complexity of designing new multi-scale architectures for a specific use case.

Routledge Handbook of Sport in the Middle East, 2022
In this chapter, I survey the football fandom ecosystem in Egypt. I first describe the media envi... more In this chapter, I survey the football fandom ecosystem in Egypt. I first describe the media environment around the sport and chart out the geographic structure of fan support for teams in the Egyptian Premier League. I then examine how fandom intersects with politics, both as Egyptian leaders have sought to use support around Egyptian football to build legitimacy, but also how Egyptian football fans have often been a significant political force acting independently of the ruling regime. I argue that different groups in Egypt have sought to use the intense interest in Egyptian football to pursue external goals, a dynamic that sometimes generates conflict when these goals are at odds. I conclude by outlining the social organization of football fandom. I illustrate how the most committed fans are young single men living in urban areas and who are more engaged in religion than the average citizen, after which I describe the role of women and the Coptic Christian minority in Egyptian football.

Proceedings of the Annual Conference of the North American Chapter of the Association for Computational Linguistics (NAACL), 2022
Causal inference methods that control for textbased confounders are becoming increasingly importa... more Causal inference methods that control for textbased confounders are becoming increasingly important in the social sciences and other disciplines where text is readily available. However, these methods rely on a critical assumption that there is no treatment leakage: that is, the text only contains information about the confounder and no information about treatment assignment. When this assumption does not hold, methods that control for text to adjust for confounders face the problem of posttreatment (collider) bias. However, the assumption that there is no treatment leakage may be unrealistic in real-world situations involving text, as human language is rich and flexible. Language appearing in a public policy document or health records may refer to the future and the past simultaneously, and thereby reveal information about the treatment assignment. In this article, we define the treatment-leakage problem, and discuss the identification as well as the estimation challenges it raises. Second, we delineate the conditions under which leakage can be addressed by removing the treatment-related signal from the text in a preprocessing step we define as text distillation. Lastly, using simulation, we show how treatment leakage introduces a bias in estimates of the average treatment effect (ATE) and how text distillation can mitigate this bias.

ArXiv Preprint
Observational studies require adjustment for confounding factors that are correlated with both th... more Observational studies require adjustment for confounding factors that are correlated with both the treatment and outcome. In the setting where the observed variables are tabular quantities such as average income in a neighborhood, tools have been developed for addressing such confounding. However, in many parts of the developing world, features about local communities may be scarce. In this context, satellite imagery can play an important role, serving as a proxy for the confounding variables otherwise unobserved. In this paper, we study confounder adjustment in this non-tabular setting, where patterns or objects found in satellite images contribute to the confounder bias. Using the evaluation of anti-poverty aid programs in Africa as our running example, we formalize the challenge of performing causal adjustment with such unstructured data—what conditions are sufficient to identify causal effects, how to perform estimation, and how to quantify the ways in which certain aspects of the unstructured image object are most predictive of the treatment decision. Via simulation, we also explore the sensitivity of satellite image-based observational inference to image resolution and to misspecification of the image-associated confounder. Finally, we apply these tools in estimating the effect of anti-poverty interventions in African communities from satellite imagery.

ArXiv Preprint
Earth observation (EO) data such as satellite imagery can have far-reaching impacts on our unde... more Earth observation (EO) data such as satellite imagery can have far-reaching impacts on our understanding of the geography of poverty, especially when coupled with machine learning (ML) and computer vision. Early research in computer vision used predictive models to estimate living conditions, especially in contexts where data availability on poverty was scarce. Recent work has progressed beyond using EO data to predict such outcomes -- now also using it to conduct causal inference. However, how such EO-ML models are used for causality remains incompletely mapped. To address this gap, we conduct a scoping review where we first document the growth of interest in using satellite images and other sources of EO data in causal analysis. We then trace the methodological relationship between spatial statistics and ML methods before discussing five ways in which EO data has been used in scientific workflows -- (1) outcome imputation for downstream causal analysis, (2) EO image deconfounding, (3) EO-based treatment effect heterogeneity, (4) EO-based transportability analysis, and (5) image-informed causal discovery. We consolidate these observations by providing a detailed workflow for how researchers can incorporate EO data in causal analysis going forward -- from data requirements to choice of computer vision model and evaluation metrics. While our discussion focuses on health and living conditions outcomes, our workflow applies to other measures of sustainable development where EO data are informative.

Proceedings of the Sixth Workshop on Natural Language Processing and Computational Social Science (NLP+ CSS 2024), 2024
We investigate the potential of large language models (LLMs) to disentangle text variables—to rem... more We investigate the potential of large language models (LLMs) to disentangle text variables—to remove the textual traces of an undesired forbidden variable in a task sometimes known as text distillation and closely related to the fairness in AI and causal inference literature. We employ a range of various LLM approaches in an attempt to disentangle text by identifying and removing information about a target variable while preserving other relevant signals. We show that in the strong test of removing sentiment, the statistical association between the processed text and sentiment is still detectable to machine learning classifiers post-LLM-disentanglement. Furthermore, we find that human annotators also struggle to disentangle sentiment while preserving other semantic content. This suggests there may be limited separability between concept variables in some text contexts, highlighting limitations of methods relying on text-level transformations and also raising questions about the robustness of disentanglement methods that achieve statistical independence in representation space.

Political Analysis, 2023
Some scholars build models to classify documents into chosen categories. Others, especially socia... more Some scholars build models to classify documents into chosen categories. Others, especially social scientists who tend to focus on population characteristics, instead usually estimate the proportion of documents in each category—using either parametric “classify-and-count” methods or “direct” nonparametric estimation of proportions without individual classification. Unfortunately, classify-and-count methods can be highly model-dependent or generate more bias in the proportions even as the percent of documents correctly classified increases. Direct estimation avoids these problems, but can suffer when the meaning of language changes between training and test sets or is too similar across categories. We develop an improved direct estimation approach without these issues by including and optimizing continuous text features, along with a form of matching adapted from the causal inference literature. Our approach substantially improves performance in a diverse collection of 73 datasets. We also offer easy-to-use software that implements all ideas discussed herein.

OSF Preprint
The impact of electoral rules on descriptive representation has generated a large body of work fo... more The impact of electoral rules on descriptive representation has generated a large body of work focused primarily on gender and secondarily on ethnicity. This study provides a parsimonious theory to explain these phenomena, centered on the size and dispersion of social groups. The theory is probed with simulations that test a complete set of counterfactuals, with a large dataset composed of mixed electoral system elections from around the world, and finally with case studies of individual elections in Russia and New Zealand. We find that multimember districts provide better representation than single-member districts only for geographically dispersed social groups that are large, but not too large, as a voting bloc (i.e., for women, understood as a group and a few ethnicities globally). Since most minority ethnic groups are concentrated in a particular region, district magnitude has little impact on their representation.

Interface, 2013
In this article, I explore the relationship between organized soccer fans— Ultras—and the Egyptia... more In this article, I explore the relationship between organized soccer fans— Ultras—and the Egyptian state. I argue that Ultra groups became politicized as they sought autonomy in public space, but faced resistance from Egyptian security forces. To make this argument, I trace the history of Ultra groups. I show how Ultras made relatively few political statements in the first years after their 2007 inception. However, these groups become increasingly politicized in reaction to police harassment. This harassment was motivated by the fact that Ultras subverted state control over public spaces. The events of the 2011 Arab Spring further politicized the Ultras and transformed them into revolutionary actors by giving them the opportunity to delegitimize the authoritarian state’s entire presence in public space. However, the greater public visibility of Ultras came at a cost, partially fracturing Ultra groups and giving state forces a desire for retaliation that was realized in the Port Said massacre. Despite these challenges, Ultra groups have continued to seek autonomy in public spaces, protesting authoritarian tendencies in the post- Mubarak era. I conclude with an afterward, explaining how Ultras not only defy authoritarianism in Egypt, but also dominant narratives about Egyptian society.

Research in Transportation Economics, 2020
How do transportation policies impact electoral politics? Empirically, the question is difficult ... more How do transportation policies impact electoral politics? Empirically, the question is difficult to answer because policies that create new transit opportunities may involve a large number of community effects. In this manuscript, we focus on a unique kind of transportation intervention—the replacement of human-operated with electronic tolls on highways—which economic theory predicts should have a concentrated effect on local property values. We find that the shift in local property values following a government program to introduce electronic tolling is correlated with a subsequent change in support for conservative candidates in presidential elections. Geographically-linked exit polling data suggests that concern over taxation is the primary observable mechanisms. To show robustness, we use various measures, one of which is computed at the individual level. We also present placebo analyses to address worries that the correlations are driven by community change, turnout, or rising incomes. Remaining limitations are also considered.

International Journal of Politics, Culture, and Society, 2014
This analysis tackles the question of whether the democratic deficit in the European Union is ass... more This analysis tackles the question of whether the democratic deficit in the European Union is associated with repeated referendums—giving voters a second chance to vote “yes” for EU treaties. Looking at repeated referendums in Ireland on the Nice and Lisbon treaties, I argue that, broadly speaking, these referendums failed to give Irish voters the meaningful ability to contest the direction of EU policy or to choose among alternative policy options, an example of how the democratic deficit can endure even when the EU incorporates electoral mechanisms. I first discuss how this theoretical insight is evidenced by five aspects of the Irish referendums. Then, I examine three of these aspects more closely in case studies of the Nice and Lisbon referendums, focusing on how leaders (1) conceptualized the failure of the initial referendums as deriving from voter incomprehension, (2) planned repeated referendums in an additional effort to ratify the treaties, and (3) mobilized “yes” voters through extreme predictions about the consequences of second “no” outcomes. I conclude by discussing socio-economic trends seen from 2003 to 2014 and their implications for issues related to the EU’s democratic deficit.

American Political Science Review, 2024
How well do governments represent the societies they serve? A key aspect of this question concern... more How well do governments represent the societies they serve? A key aspect of this question concerns the extent to which leaders reflect the demographic features of the population they represent. To address this important issue in a systematic manner, we propose a unified approach for measuring descriptive representation. We apply this approach to newly collected data describing the ethnic, linguistic, religious, and gender identities of over fifty thousand leaders serving in 1,552 political bodies across 156 countries. Strikingly, no country represents social groups in rough proportion to their share of the population. To explain this shortfall, we focus on compositional factors—the size of political bodies as well as the number and relative size of social groups. We investigate these factors using a simple model based on random sampling and the original data described above. Our analyses demonstrate that roughly half of the variability in descriptive representation is attributable to compositional factors.

ArXiv Preprint
Scholars studying organizations often work with multiple datasets lacking shared identifiers or c... more Scholars studying organizations often work with multiple datasets lacking shared identifiers or covariates. In such situations, researchers usually use approximate string (“fuzzy”) matching methods to combine datasets. String matching, although useful, faces fundamental challenges. Even where two strings appear similar to humans, fuzzy matching often struggles because it fails to adapt to the informativeness of the character combinations. In response, a number of machine learning methods have been developed to refine string matching. Yet, the effectiveness of these methods is limited by the size and diversity of training data. This paper introduces data from a prominent employment networking site (LinkedIn) as a massive train- ing corpus to address these limitations. By leveraging information from the LinkedIn corpus regarding organizational name-to-name links, we incorporate trillions of name pair examples into various methods to enhance existing matching benchmarks and performance by explicitly maximizing match probabilities. We also show how relationships between organization names can be modeled using a network representation of the LinkedIn data. In illustrative merging tasks involving lobbying firms, we document improvements when using the LinkedIn corpus in matching calibration and make all data and methods open source.

Proceedings of the Second Conference on Causal Learning and Reasoning (CLeaR)
Randomized controlled trials (RCTs) are considered the gold standard for estimating the Average T... more Randomized controlled trials (RCTs) are considered the gold standard for estimating the Average Treatment Effect (ATE) of interventions. One important use of RCTs is to study the causes of global poverty—a subject explicitly cited in the 2019 Sveriges Riksbank Prize in Economic Sciences in Memory of Alfred Nobel awarded to Duflo, Banerjee, and Kremer “for their experimental approach to alleviating global poverty.” Because the ATE is a population summary, researchers often want to better understand how the treatment effect varies across different populations by con- ditioning on tabular variables such as age and ethnicity that were measured during the RCT data collection. Although such variables carry substantive importance, they are often only observed only near the time of the experiment: exclusive use of such variables may fail to capture historical, geographical, or neighborhood-specific contributors to effect variation. In global poverty research, when the geographical location of the experiment units is approximately known, satellite imagery can provide a window into such historical and geographical factors important for understanding heterogeneity. However, there is no causal inference method that specifically enables applied researchers to analyze Conditional Average Treatment Effects (CATEs) from images. In this paper, we develop a deep probabilistic modeling framework that identifies clusters of images with similar treatment effect distributions, enabling researchers to analyze treatment effect variation by image. Our interpretable image CATE model also emphasizes an image sensitivity factor that quantifies the importance of image segments in contributing to the mean effect cluster prediction. We compare the proposed methods against alternatives in simulation; additionally, we show how the model works in an actual RCT, estimating the effects of an anti-poverty intervention in northern Uganda and obtaining a posterior predictive distribution over treatment effects for the rest of the country where no experimental data was collected. We make code for all modeling strategies available in an open-source software package and discuss their applicability in other domains (such as the biomedical sciences) where image data are also prevalent.

ArXiv Preprint
The causalimages R package enables causal inference with image and image sequence data, providing... more The causalimages R package enables causal inference with image and image sequence data, providing new tools for integrating novel data sources like satellite and bio-medical imagery into the study of cause and effect. One set of functions enables image-based causal inference analyses. For example, one key function decomposes treatment effect heterogeneity by images using an interpretable Bayesian framework. This allows for determining which types of images or image sequences are most responsive to interventions. A second modeling function allows researchers to control for confounding using images. The package also allows investigators to produce embeddings that serve as vector sum-maries of the image or video content. Finally, infrastructural functions are also provided, such as tools for writing large-scale image and image sequence data as sequentialized byte strings for more rapid image analysis. causalimages therefore opens new capabilities for causal inference in R, letting researchers use informative imagery in substantive analyses in a fast and accessible manner

ArXiv Preprint
Randomized controlled experiments are susceptible to imbalance on covariates predictive of the ou... more Randomized controlled experiments are susceptible to imbalance on covariates predictive of the outcome. Rerandomization and deterministic treatment assignment are two proposed solutions. This paper explores the relationship between rerandomization and deterministic assignment, showing how deterministic assignment is an extreme case of rerandomization. The paper argues that in small experiments, both fully randomized and fully deterministic assignment have limitations. Instead, the researcher should consider setting the rerandomization acceptance probability based on an analysis of covariates and assumptions about the data structure to achieve an optimal alignment between randomness and balance. This allows for the calcula-tion of minimum p-values along with valid permutation tests and fiducial intervals. The paper also introduces tools, including a new, open-source R package named fastrerandomize, to implement rerandomization and explore options for optimal rerandomization acceptance thresholds.

ArXiv Preprint
Many social and environmental phenomena are associated with macroscopic changes in the built envi... more Many social and environmental phenomena are associated with macroscopic changes in the built environment, captured by satellite imagery on a global scale and with daily temporal resolution. While widely used for prediction, these images and especially image sequences remain underutilized for causal inference, especially in the context of randomized controlled trials (RCTs), where causal identification is established by design. In this paper, we develop and compare a set of general tools for analyzing Conditional Average Treatment Effects (CATEs) from temporal satellite data that can be applied to any RCT where geographical identifiers are available. Through a simulation study, we analyze different modeling strategies for estimating CATE in sequences of satellite images. We find that image sequence representation models with more parameters generally yield a greater ability to detect heterogeneity. To explore the role of model and data choice in practice, we apply the approaches to two influential RCTs—Banerjee et al. (2015), a poverty study in Cusco, Peru, and Bolsen et al. (2014), a water conservation experiment in Georgia, USA. We benchmark our image sequence models against image-only, tabular-only, and combined image-tabular data sources, summarizing practical implications for investigators in a multivariate analysis. Land cover classifications over satellite images facilitate interpretation of what image features drive heterogeneity. We also show robustness to data and model choice of satellite-based generalization of the RCT results to larger geographical areas outside the original. Overall, this paper shows how satellite sequence data can be incorporated into the analysis of RCTs, and provides evidence about the implications of data, model, and evaluation metric choice for causal analysis.
Uploads
articles by Connor T Jerzak