Papers by James Montgomery
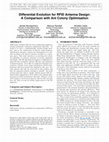
Proceedings of the 13th annual conference on Genetic and evolutionary computation - GECCO '11, 2011
Differential evolution (DE) has been traditionally applied to solving benchmark continuous optimi... more Differential evolution (DE) has been traditionally applied to solving benchmark continuous optimisation functions. To enable it to solve a combinatorially oriented design problem, such as the construction of effective radio frequency identification antennas, requires the development of a suitable encoding of the discrete decision variables in a continuous space. This study introduces an encoding that allows the algorithm to construct antennas of varying complexity and length. The DE algorithm developed is a multiobjective approach that maximises antenna efficiency and minimises resonant frequency. Its results are compared with those generated by a family of ant colony optimisation (ACO) metaheuristics that have formed the standard in this area. Results indicate that DE can work well on this problem and that the proposed solution encoding is suitable. On small antenna grid sizes (hence, smaller solution spaces) DE performs well in comparison to ACO, while as the solution space increases its relative performance decreases. However, as the ACO employs a local search operator that the DE currently does not, there is scope for further improvement to the DE approach.
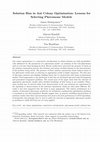
Computers & Operations Research, 2008
Ant colony optimisation is a constructive metaheuristic in which solutions are built probabilisti... more Ant colony optimisation is a constructive metaheuristic in which solutions are built probabilistically influenced by the parameters of a pheromone model-an analogue of the trail pheromones used by real ants when foraging for food. Recent studies have uncovered the presence of biases in the solution construction process, the existence and nature of which depend on the characteristics of the problem being solved. The presence of these solution construction biases induces biases in the pheromone model used, so selecting an appropriate model is highly important. The first part of this paper presents new findings bridging biases due to construction with biases in pheromone models. Novel approaches to the prediction of this bias are developed and used with the knapsack and generalised assignment problems. The second part of the paper deals with the selection of appropriate pheromone models when detailed knowledge of their biases is not available. Pheromone models may be derived either from characteristics of the way solutions are represented by the algorithm or characteristics of the solutions represented, which are often quite different. Recently it has been suggested that the latter is more appropriate. The relative performance of a number of alternative pheromone models for six well-known combinatorial optimisation problems is examined to test this hypothesis. Results suggest that, in general, modelling characteristics of solutions (rather than their representations) does lead to the best performance in ACO algorithms. Consequently, this principle may be used to guide the selection of appropriate pheromone models in problems to which ACO has not yet been applied.
Lecture Notes in Computer Science, 2002
Ant Colony Optimisation is a maturing class of meta-heuristic search algorithms for discrete opti... more Ant Colony Optimisation is a maturing class of meta-heuristic search algorithms for discrete optimisation problems that are being increasingly applied to real world problems in areas such as communications and transportation. As these techniques systematically scan the set of possible solution elements before choosing a particular one, the computational time required for each step of the algorithm can be large. One way to overcome this is to limit the number of element choices to a sensible subset, or candidate set. This paper describes some novel generic candidate set strategies and tests these on the travelling salesman and car sequencing problems. The results show that the use of candidate sets helps to find competitive solutions to the test problems in a relatively short amount of time.
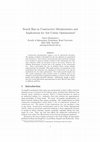
Lecture Notes in Computer Science, 2004
Constructive metaheuristics explore a tree of constructive decisions, the topology of which is de... more Constructive metaheuristics explore a tree of constructive decisions, the topology of which is determined by the way solutions are represented and constructed. Some solution representations allow particular solutions to be reached on a greater number of paths in this construction tree than other solutions, which can introduce a bias to the search. However, the ultimate determinant of search bias is the topology of the construction tree. This is particularly the case in problems where certain solution representations are infeasible. This paper presents an examination of the mechanisms that determine the topologies of construction trees and the implications for ant colony optimisation. The results provide insights into why certain assignment orders perform better in problems such as the quadratic and generalised assignment problems, in terms of both solution quality and avoiding infeasible solutions. Additionally, insight is gained into why certain pheromone representations are more effective than others on different problems.
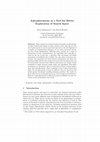
Lecture Notes in Computer Science, 2002
Many animals use chemical substances known as pheromones to induce behavioural changes in other m... more Many animals use chemical substances known as pheromones to induce behavioural changes in other members of the same species. The use of pheromones by ants in particular has lead to the development of a number of computational analogues of ant colony behaviour including Ant Colony Optimisation. Although many animals use a range of pheromones in their communication, ant algorithms have typically focused on the use of just one, a substance that encourages succeeding generations of (artificial) ants to follow the same path as previous generations. Ant algorithms for multi-objective optimisation and those employing multiple colonies have made use of more than one pheromone, but the interactions between these different pheromones are largely simple extensions of single criterion, single colony ant algorithms. This paper investigates an alternative form of interaction between normal pheromone and anti-pheromone. Three variations of Ant Colony System that apply the anti-pheromone concept in different ways are described and tested against benchmark travelling salesman problems. The results indicate that the use of anti-pheromone can lead to improved performance. However, if anti-pheromone is allowed too great an influence on ants' decisions, poorer performance may result.
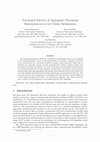
Artificial Life, 2005
Ant Colony Optimisation (ACO) is a constructive metaheuristic that uses an analogue of ant trail ... more Ant Colony Optimisation (ACO) is a constructive metaheuristic that uses an analogue of ant trail pheromones to learn about good features of solutions. Critically, the pheromone representation for a particular problem is usually chosen intuitively rather than by following any systematic process. In some representations, distinct solutions appear multiple times, increasing the effective size of the search space and potentially misleading ants as to the true learned value of those solutions. In this article, we present a novel system for automatically generating appropriate pheromone representations based on the characteristics of the problem model that ensures unique pheromone representation of solutions. This is the first stage in the development of a generalised ACO system that could be applied to a wide range of problems with little or no modification. However, the system we propose may be used in the development of any problem-specific ACO algorithm.
Proceedings of Inaugural Workshop on Artificial Life, Adelaide, Australia, 2001
Abstract. Ant colony optimisation techniques are usually guided by pheromone and heuristic cost i... more Abstract. Ant colony optimisation techniques are usually guided by pheromone and heuristic cost information when choosing the next element to add to a solution. However, while an individual element may be attractive, usually its long term consequences are neither known nor considered. For instance, a short link in a TSP may be incorporated into an ant's solution, yet, as a consequence of this link, the rest of the path may be longer than if another link was chosen. The Accumulated Experience Ant Colony uses the previous experiences ...
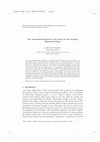
International Journal of Computational Intelligence and Applications, 2003
Ant colony optimization techniques are usually guided by pheromone and heuristic cost information... more Ant colony optimization techniques are usually guided by pheromone and heuristic cost information when choosing the next element to add to a solution. However, while an individual element may be attractive, usually its long term consequences are neither known nor considered. For instance, a short link in a traveling salesman problem may be incorporated into an ant's solution, yet, as a consequence of this link, the rest of the path may be longer than if another link was chosen. The Accumulated Experience Ant Colony uses the previous experiences of the colony to guide in the choice of elements. This is in addition to the normal pheromone and heuristic costs. Two versions of the algorithm are presented, the original and an improved AEAC that makes greater use of accumulated experience. The results indicate that the original algorithm finds improved solutions on problems with less than 100 cities, while the improved algorithm finds better solutions on larger problems.
Many algorithms have been devised for tackling combinatorial optimisation problems (COPs). Tradit... more Many algorithms have been devised for tackling combinatorial optimisation problems (COPs). Traditional Operations Research (OR) techniques such as Branch and Bound and Cutting Planes Algorithms can, given enough time, guarantee an optimal solution as they explicitly exploit features of the optimisation function they are solving. Specialised heuristics exist for most COPs that also exploit features of the optimisation function to arrive at a good, but probably not optimal, solution.
Abstract The aim of the paper is to present the results of research into component based software... more Abstract The aim of the paper is to present the results of research into component based software development by providing a specification mechanism allowing searching for components in a component repository. A new component classification framework is proposed based on which a Component Description Manager has been designed and implemented.
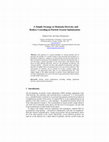
Each particle in a swarm maintains its current position and its personal best position. It is use... more Each particle in a swarm maintains its current position and its personal best position. It is useful to think of these personal best positions as a population of attractors – updates to current positions are based on attractions to these personal best positions. If the population of attractors has high diversity, it will encourage a broad exploration of the search space with particles being drawn in many different directions. However, the population of attractors can converge quickly – attractors can draw other particles towards them, and these particles can update their own personal bests to be near the first attractor. This convergence of attractors can be reduced by having a particle update the attractor it has approached rather than its own attractor/personal best. This simple change to the update procedure in particle swarm optimization incurs minimal computational cost, and it can lead to large performance improvements in multi-modal search spaces.
In order to relate machine learning problems we argue that we need to be able to articulate what ... more In order to relate machine learning problems we argue that we need to be able to articulate what is meant by a single machine learning problem. By attempting to name the various aspects of a learning problem we hope to clarify ways in which learning problems might be related to each other. We tentatively put forward a proposal for an anatomy of learning problems that will serve as scaffolding for posing questions about relations. After surveying the way learning problems are discussed in a range of repositories and services. We then argue that the terms used to describe problems to better understand a range of viewpoints within machine learning ranging from the theoretical to the practical.
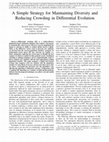
Differential evolution (DE) is a widely-effective population-based continuous optimiser that requ... more Differential evolution (DE) is a widely-effective population-based continuous optimiser that requires convergence to automatically scale its moves. However, once its population has begun to converge its ability to conduct global search is diminished, as the difference vectors used to generate new solutions are derived from the current population members' positions. In multi-modal search spaces DE may converge too rapidly, i.e., before adequately exploring the search space to identify the best region(s) in which to conduct its finer-grained search. Traditional crowding or niching techniques can be computationally costly or fail to compare new solutions with the most appropriate existing population member. This paper proposes a simple intervention strategy that compares each new solution with the population member it is most likely to be near, and prevents those moves that are below a threshold that decreases over the algorithm's run, allowing the algorithm to ultimately converge. Comparisons with a standard DE algorithm on a number of multi-modal problems indicate that the proposed technique can achieve real and sizable improvements.
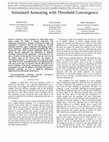
Stochastic search techniques for multi-modal search spaces require the ability to balance explora... more Stochastic search techniques for multi-modal search spaces require the ability to balance exploration with exploitation. Exploration is required to find the best region, and exploitation is required to find the best solution (i.e. the local optimum) within this region. Compared to hill climbing which is purely exploitative, simulated annealing probabilistically allows “backward” steps which facilitate exploration. However, the balance between exploration and exploitation in simulated annealing is biased towards exploitation – improving moves are always accepted, so local (greedy) search steps can occur at even the earliest stages of the search process. The purpose of “thresheld convergence” is to have these early-stage local search steps “held” back by a threshold function. It is hypothesized that early local search steps can interfere with the effectiveness of a search technique’s (concurrent) mechanisms for global search. Experiments show that the addition of thresheld convergence to simulated annealing can lead to significant performance improvements in multi-modal search spaces.
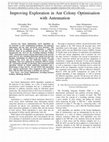
Ant Colony Optimisation (ACO) algorithms use two heuristics to solve computational problems: one ... more Ant Colony Optimisation (ACO) algorithms use two heuristics to solve computational problems: one long-term (pheromone) and the other short-term (local heuristic). This paper details the development of antennation, a mid-term heuristic based on an analogous process in real ants. This is incorporated into ACO for the Travelling Salesman Problem (TSP). Antennation involves sharing information of the previous paths taken by ants, including information gained from previous meetings. Antennation was added to the Ant System (AS), Ant Colony System (ACS) and Ant Multi-Tour System (AMTS) algorithms. Tests were conducted on symmetric TSPs of varying size. Antennation provides an advantage when incorporated into algorithms without an inbuilt exploration mechanism and a disadvantage to those that do. AS and AMTS with antennation have superior performance when compared to their canonical form, with the effect increasing as problem size increases.
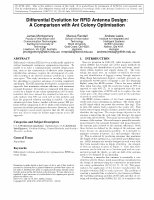
… Computation (GECCO'11), Jan 1, 2011
Differential evolution (DE) has been traditionally applied to solving benchmark continuous optimi... more Differential evolution (DE) has been traditionally applied to solving benchmark continuous optimisation functions. To enable it to solve a combinatorially oriented design problem, such as the construction of effective radio frequency identification antennas, requires the development of a suitable encoding of the discrete decision variables in a continuous space. This study introduces an encoding that allows the algorithm to construct antennas of varying complexity and length. The DE algorithm developed is a multiobjective approach that maximises antenna efficiency and minimises resonant frequency. Its results are compared with those generated by a family of ant colony optimisation (ACO) metaheuristics that have formed the standard in this area. Results indicate that DE can work well on this problem and that the proposed solution encoding is suitable. On small antenna grid sizes (hence, smaller solution spaces) DE performs well in comparison to ACO, while as the solution space increases its relative performance decreases. However, as the ACO employs a local search operator that the DE currently does not, there is scope for further improvement to the DE approach.
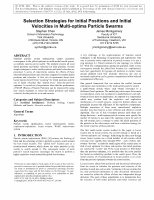
Evolutionary Computation (GECCO'11), Jan 1, 2011
Standard particle swarm optimization cannot guarantee convergence to the global optimum in multi-... more Standard particle swarm optimization cannot guarantee convergence to the global optimum in multi-modal search spaces, so multiple swarms can be useful. The multiple swarms all need initial positions and initial velocities for their particles. Several simple strategies to select initial positions and initial velocities are presented. A series of experiments isolates the effects of these selected initial positions and velocities compared to random initial positions and velocities. A first set of experiments shows how locust swarms benefit from "scouting" for initial positions and the use of initial velocities that "launch away" from the previous optimum. A second set of experiments show that the performance of WoSP (Waves of Swarm Particles) can be improved by using new search strategies to select the initial positions and initial velocities for the particles in its sub-swarms.
Evolutionary Computation (GECCO'11), Jan 1, 2011
The automotive deployment problem is a real-world constrained multiobjective assignment problem i... more The automotive deployment problem is a real-world constrained multiobjective assignment problem in which software components must be allocated to processing units distributed around a car's chassis. Prior work has shown that evolutionary algorithms such as NSGA-II can produce good quality solutions to this problem. This paper presents a population-based ant colony optimisation (PACO) approach that uses a single pheromone memory structure and a range of local search operators. The PACO and prior NSGA-II are compared on two realistic problem instances. Results indicate that the PACO is generally competitive with NSGA-II and performs more effectively as problem complexity-size and number of objectives-is increased.
… Sixth IEEE International Conference on e …, Jan 1, 2010
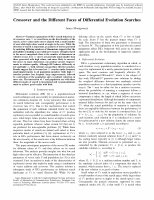
… Computation (CEC), 2010 IEEE Congress on, Jan 1, 2010
Common explanations of DE's search behaviour as its crossover rate Cr is varied focus on the dire... more Common explanations of DE's search behaviour as its crossover rate Cr is varied focus on the directionality of the search, as low values make moves aligned with a small number of axes while high values search at angles to the axes. While the direction of search is important, an analysis of moves generated by mutating differing numbers of dimensions suggests that the probability of making a successful move is more strongly related to the move's magnitude than to the number of dimensions in which it occurs. Low Cr moves are generally much smaller than those generated with high values, and more likely to succeed, but moves in many dimensions can produce greater improvements in solution quality. Although DE behaves differently at low and high Cr, both extremes can produce effective searches. Results suggest this is because low Cr searches make frequent, small improvements to all population members while high Cr searches produce less frequent, large improvements, followed by contraction of the population and a resultant reduction in move size. The interaction of F and population size with these different modes of search is investigated and recommendations made to achieve good results with both.
Uploads
Papers by James Montgomery