Papers by Mayooran Thevaraja
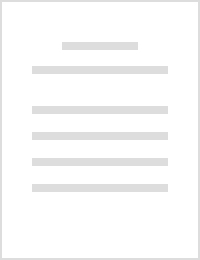
Advances in Intelligent Systems and Computing, 2019
In this Big-data and computational innovation era, advanced level analysis and modelling strategi... more In this Big-data and computational innovation era, advanced level analysis and modelling strategies are essential in data science to understanding the individual activities which occur within very complex behavioral, socio-economic and ecological systems. However, the scales at which models can be developed, and the subsequent problems they can inform, are often limited by our inability or challenges to effectively understand data that mimic interactions at the finest spatial, temporal, or organizational resolutions. Linear regression analysis is the one of the widely used methods for investigating such relationship between variables. Multicollinearity is one of the major problem in regression analysis. Multicollinearity can be reduced by using the appropriate regularized regression methods. This study aims to measure the robustness of regularized regression models such as ridge and Lasso type models designed for the high dimensional data having the multicollinearity problems. Empir...
American Journal of Applied Mathematics and Statistics, 2016
Solving a system of equations by Ax=b, where A is a n n matrix and b and n 1 vector, can sometime... more Solving a system of equations by Ax=b, where A is a n n matrix and b and n 1 vector, can sometime be a daunting task because solving for x can be difficult. If you were given an algorithm that was efficient, that’s great! What if you could make it solve the problem even faster? That’s even better. We will first take a look at establishing the basics of the successive over-relaxation method (SOR for short), then we’ll look at a real-world problem we applied the SOR method to, solving the heat-equation when a constant boundary temperature is applied to a flat plate.
Sri Lankan Journal of Applied Statistics, 2014
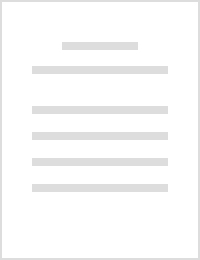
Researchers are often interested in studying the relationships between one variable and several o... more Researchers are often interested in studying the relationships between one variable and several other variables. Regression analysis is the statistical method for investigating such relationship, and it is one of the most commonly used statistical Methods in many scientific fields such as financial data analysis, medicine, biology, agriculture, economics, engineering, sociology, geology. However, the primary form of the regression analysis, ordinary least squares (OLS) is not suitable for actuarial applications because the relationships are often nonlinear, and the probability distribution of the response variable may be non-Gaussian distribution. One of the methods that have been successful in overcoming these challenges is the generalized linear model (GLM), which requires that the response variable have a distribution from the exponential family. In this research work, we study copula regression as an alternative method to OLS and GLM. The significant advantage of a copula regres...
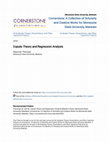
Researchers are often interested to study in the relationships between one variable and several o... more Researchers are often interested to study in the relationships between one variable and several other variables. Regression analysis is the statistical method for investigating such relationship and it is one of the most commonly used statistical Methods in many scientific fields such as financial data analysis, medicine, biology, agriculture, economics, engineering, sociology, geology, etc. But basic form of the regression analysis, ordinary least squares (OLS) is not suitable for actuarial applications because the relationships are often nonlinear and the probability distribution of the response variable may be non-Gaussian distribution. One of the method that has been successful in overcoming these challenges is the generalized linear model (GLM), which requires that the response variable have a distribution from the exponential family. In this research work, we study copula regression as an alternative method to OLS and GLM. The major advantage of a copula regression is that the...
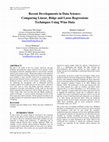
Big data is the reality of the 21st century. However, big data modeling and prediction require ad... more Big data is the reality of the 21st century. However, big data modeling and prediction require advanced level analytics which encompasses both the computing-intensive and statistics-oriented analysis tools in data science. Regression analysis is the statistical method for predictive modeling, and it is one of the most commonly used methods in many scientific fields such as engineering, the physical and chemical sciences, economics, management, life and biological sciences, and the social sciences, sociology, geology, etc. Satisfying the assumptions such as collinearity between variables ought to be a significant issue in data science. Advanced level tools such as Lasso and Ridge regression methods are designed to overcome such problem. In this study we discussed about comparing linear regression with the Ridge and Lasso. The Vinho Verde white wine test data from the Minho (northwest) region of Portugal is used to analyze advantages of each of the three regression analysis methods. All the required calculations and graphical displays are performed using the R software for statistical computing.
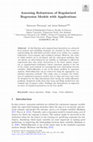
ICMSEM 2019. Advances in Intelligent Systems and Computing, vol 1001. Springer, Cham., 2019
In this Big-data and computational innovation era, advanced level analysis and modelling strategi... more In this Big-data and computational innovation era, advanced level analysis and modelling strategies are essential in data science to understanding the individual activities which occur within very complex behavioral, socioeconomic and ecological systems. However, the scales at which models can be developed, and the subsequent problems they can inform, are often limited by our inability or challenges to effectively understand data that mimic interactions at the finest spatial, temporal , or organizational resolutions. Linear regression analysis is the one of the widely used methods for investigating such relationship between variables. Multicollinearity is one of the major problem in regression analysis. Multicollinearity can be reduced by using the appropriate reg-ularized regression methods. This study aims to measure the robust-ness of regularized regression models such as ridge and Lasso type models designed for the high dimensional data having the multicollinearity problems. Empirical results show that Lasso and Ridge models have less residual sum of squares values. Findings also demonstrate an improved accuracy of estimated parameters on the best model.
Solving a system of equations by = , where A is a × matrix and b and × 1 vector, can sometime be ... more Solving a system of equations by = , where A is a × matrix and b and × 1 vector, can sometime be a daunting task because solving for x can be difficult. If you were given an algorithm that was efficient, that's great! What if you could make it solve the problem even faster? That's even better. We will first take a look at establishing the basics of the successive over-relaxation method (SOR for short), then we'll look at a real-world problem we applied the SOR method to, solving the heat-equation when a constant boundary temperature is applied to a flat plate.
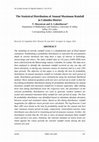
The modeling of extreme rainfall events is a fundamental part of flood hazard estimation. Establi... more The modeling of extreme rainfall events is a fundamental part of flood hazard estimation. Establishing a probability distribution to represent the precipitation depth at various durations has long been a topic of interest in hydrology, meteorology and others. The daily rainfall data of 110 years have been collected from the Meteorology station, Colombo, Sri Lanka. The data were then analyzed to identify the maximum rainfall received on any one day (24 hours duration), in during any monsoon season (4 seasons) and in a year (365 days period). The objective of this paper is to identify the best fit probability distribution of annual maximum rainfall in Colombo district for each period of study. Distribution parameters were estimated by using the maximum likelihood method. Three statistical goodness of fit test were carried out in order to find the best fitting probability distribution among 45 probability distributions for annual maximum rainfall and maximum rainfall for 4 seasons separately. After finding three best fitting distributions from the respective tests, the parameters of the selected probability distributions are used to generate random numbers for actual and estimated maximum daily rainfall for each period of study. The best fit probability distribution was identified based on minimum absolute deviation between actual and estimated values. Based on this fitting distribution, rainfall magnitudes for different return periods were calculated. The log-Pearson 3 and Burr (4P) were found as the best fit probability model for the annual and first inter monsoon season period of study, respectively. Generalized extreme value distribution was observed in remaining period of monsoon seasons. Further, the distribution reveals that the 216 mm or more of annual maximum daily rainfall return period is ten years. Similarly, the relevant estimates of return levels are listed against the return periods for extreme rainfall events during the four seasons of a year.
The modelling of extreme rainfall events is fundamental part of flood hazard estimation.
Uploads
Papers by Mayooran Thevaraja