Papers by burhan rashid
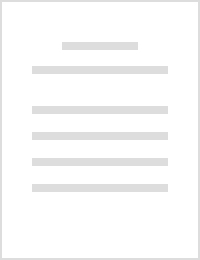
Lecture Notes in Electrical Engineering, 2020
Automated identification of herbarium species is of great interest as quite a number of these col... more Automated identification of herbarium species is of great interest as quite a number of these collections are still unidentified while others need to be updated following recent taxonomic knowledge. One challenging task in automated identification process of these species is the existence of visual noise such as plant information labels, color codes and other scientific annotations which are mostly placed at different locations on the herbarium mounting sheet. This kind of noise needs to be removed before applying different species identification models as it can significantly affect the models’ performance. In this work we propose the use of deep learning semantic segmentation model as a method for removing the background noise from herbarium images. Two different semantic segmentation models, namely DeepLab version three plus (DeepLabv3+) and the Full- Resolution Residual Networks (FRNN-A), were applied and evaluated in this study. The results indicate that FRNN-A performed slightly better with a mean Intersection of Union (IoU) of 99.2% compared to 98.1% mean IoU attained by DeepLabv3+ model on the test set. The pixel -wise accuracy for two classes (herbarium specimen and background) was found to be 99.5% and 99.7%, respectively using FRNN-A model while the DeepLabv3+ was able to segment herbarium specimen and the rest of the background with a pixel-wise accuracy of 98.4% and 99.6%, respectively. This work evidently suggests that deep learning semantic segmentation could be successfully applied as a pre-processing step in removing visual noise existing in herbarium images before applying different classification models.
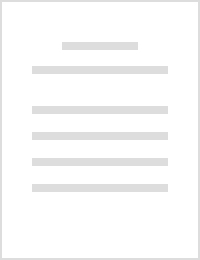
Advances in Intelligent Systems and Computing, 2018
One of the factors that often result in an unforeseen shortage or expiry of medication is the abs... more One of the factors that often result in an unforeseen shortage or expiry of medication is the absence of, or continued use of ineffective, inventory forecasting mechanisms. Unforeseen shortage of perhaps lifesaving medication potentially translates to a loss of lives, while overstocking can affect both medical budgeting as well as healthcare provision. Evidence from literature indicates that forecasting techniques can be a robust approach to address this inventory management challenge. The purpose of this study is to propose an inventory forecasting solution based on time series data mining techniques applied to transactional data of medical consumptions. Four different machine learning algorithms for time series analysis were explored and their forecasting accuracy estimates were compared. Results reveal that Gaussian Processes (GP) produced better results compared to other explored techniques (Support Vector Machine Regression (SMOreg), Multilayer Perceptron (MLP) and Linear Regression (LR)) for four weeks ahead prediction. The proposed solution is based on secondary data and can be replicated or altered to suit different constraints of other medical stores. Therefore, this work evidently suggests that the use of data mining techniques could prove a feasible solution to a prevalent challenge in medical inventory forecasting process. It also outlines the steps to be taken in this process and proposes a method to estimate forecasting risk that helps in deploying obtained results in the respective domain area.
Recent development in AI has enabled the expansion of its application to multiple domains. From m... more Recent development in AI has enabled the expansion of its application to multiple domains. From medical treatment, gaming, manufacturing to daily business processes. A huge amount of money has been poured into AI research due to its exciting discoveries. Technology giants like Google, Facebook, Amazon, and Baidu are the driving forces in the field today. But the rapid growth and excitement that the technology offers obscure us from looking at the impact it brings on our society. This short paper gives a brief history of AI and summarizes various social, economic and ethical issues that are impacting our society today. We hope that this work will provide a useful starting point and perhaps reference for newcomers and stakeholders of the field.
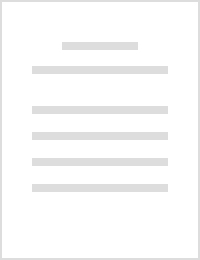
Lecture Notes in Electrical Engineering, 2020
The identification of plant species is fundamental for effective study and management of biodiver... more The identification of plant species is fundamental for effective study and management of biodiversity. For automated plant species classification, a combination of leaf features like shapes, texture and color are commonly used. However, in herbariums, the samples collected for each species are often limited and during preservation step some of the feature details disappear making automated classification a challenging task. In this study, we aimed at applying machine learning techniques in automating herbarium species identification from leaf traits extracted from images of the families Annonaceae, Euphorbiaceae and Dipterocarpaceae. Furthermore, we investigated the application of Synthetic Minority Over-sampling Technique (SMOTE) in improving classifier performance on the imbalance datasets. Three machine learning techniques namely Linear Discriminant Analysis (LDA), Random Forest (RF) and Support Vector Machine (SVM) were applied with/without SMOTE. For Annonaceae species, the best accuracy was 56% by LDA after applying SMOTE. For Euphorbiaceae, the best accuracy was 79% by SVM without SMOTE. For inter-species classification between Annonaceae and Euphorbiaceae, the best accuracy of 63% was achieved by LDA without SMOTE. An accuracy of 85% was achieved by LDA for Dipterocarpaceae species while 91% accuracy was obtained by both RF and SVM for inter-family classification between the two balanced datasets of Annonaceae and Euphorbiaceae. The results of this study show the feasibility of using extracted traits for building accurate species identification models for Family Dipterocarpaceae and Euphorbiaceae, however, the features used did not yield good results for Annonaceae family. Furthermore, there was no significant improvement when SMOTE technique was applied.
International Conference on Advances in Computing and Technology (ICACT–2020), 2020
Recent development in AI has enabled the expansion of its application to multiple domains. From m... more Recent development in AI has enabled the expansion of its application to multiple domains. From medical treatment, gaming, manufacturing to daily business processes. A huge amount of money has been poured into AI research due to its exciting discoveries. Technology giants like Google, Facebook, Amazon, and Baidu are the driving forces in the field today. But the rapid growth and excitement that the technology offers obscure us from looking at the impact it brings on our society. This short paper gives a brief history of AI and summarizes various social, economic and ethical issues that are impacting our society today. We hope that this work will provide a useful starting point and perhaps reference for newcomers and stakeholders of the field.

The identification of plant species is fundamental for effective study and management of biodiver... more The identification of plant species is fundamental for effective study and management of biodiversity. For automated plant species classification, a combination of leaf features like shapes, texture and color are commonly used. However, in herbariums, the samples collected for each species are often limited and during preservation step some of the feature details disappear making auto- mated classification a challenging task. In this study, we aimed at applying ma- chine learning techniques in automating herbarium species identification from leaf traits extracted from images of the families Annonaceae, Euphorbiaceae and Dipterocarpaceae. Furthermore, we investigated the application of Synthetic Mi- nority Over-sampling Technique (SMOTE) in improving classifier performance on the imbalance datasets. Three machine learning techniques namely Linear Discriminant Analysis (LDA), Random Forest (RF) and Support Vector Machine (SVM) were applied with/without SMOTE. For Annonaceae species, the best ac- curacy was 56% by LDA after applying SMOTE. For Euphorbiaceae, the best accuracy was 79% by SVM without SMOTE. For inter-species classification be- tween Annonaceae and Euphorbiaceae, the best accuracy of 63% was achieved by LDA without SMOTE. An accuracy of 85% was achieved by LDA for Dip- terocarpaceae species while 91% accuracy was obtained by both RF and SVM for inter-family classification between the two balanced datasets of Annonaceae and Euphorbiaceae. The results of this study show the feasibility of using ex- tracted traits for building accurate species identification models for Family Dip- terocarpaceae and Euphorbiaceae, however, the features used did not yield good results for Annonaceae family. Furthermore, there was no significant improve- ment when SMOTE technique was applied.

One of the factors that often result in an unforeseen shortage or expiry of medication is the abs... more One of the factors that often result in an unforeseen shortage or expiry of medication is the absence of, or continued use of ineffective, inventory forecasting mechanisms. Unforeseen shortage of perhaps lifesaving medication potentially translates to a loss of lives, while overstocking can affect both medical budgeting as well as healthcare provision. Evidence from literature indicates that forecasting techniques can be a robust approach to address this inventory management challenge. The purpose of this study is to propose an inventory forecasting solution based on time series data mining techniques applied to transactional data of medical consumptions. Four different machine learning algorithms for time series analysis were explored and their forecasting accuracy estimates were compared. Results reveal that Gaussian Processes (GP) produced better results compared to other explored techniques (Support Vector Machine Regression (SMOreg), Multilayer Perceptron (MLP) and Linear Regression (LR)) for four weeks ahead prediction. The proposed solution is based on secondary data and can be replicated or altered to suit different constraints of other medical stores. Therefore, this work evidently suggests that the use of data mining techniques could prove a feasible solution to a prevalent challenge in medical inventory forecasting process. It also outlines the steps to be taken in this process and proposes a method to estimate forecasting risk that helps in deploying obtained results in the respective domain area.
incollections by burhan rashid

Automated identification of herbarium species is of great interest as quite a number of these col... more Automated identification of herbarium species is of great interest as quite a number of these collections are still unidentified while others need to be updated following recent taxonomic knowledge. One challenging task in automated identification process of these species is the existence of visual noise such as plant information labels, color codes and other scientific annotations which are mostly placed at different locations on the herbarium mounting sheet. This kind of noise needs to be removed before applying different species identification models as it can significantly affect the models’ performance. In this work we propose the use of deep learning semantic segmentation model as a method for removing the background noise from herbarium images. Two different semantic segmentation models, namely DeepLab version three plus (DeepLabv3+) and the Full- Resolution Residual Networks (FRNN-A), were applied and evaluated in this study. The results indicate that FRNN-A performed slightly better with a mean Intersection of Union (IoU) of 99.2% compared to 98.1% mean IoU attained by DeepLabv3+ model on the test set. The pixel -wise accuracy for two classes (herbarium specimen and background) was found to be 99.5% and 99.7%, respectively using FRNN-A model while the DeepLabv3+ was able to segment herbarium specimen and the rest of the background with a pixel-wise accuracy of 98.4% and 99.6%, respectively. This work evidently suggests that deep learning semantic segmentation could be successfully applied as a pre-processing step in removing visual noise existing in herbarium images before applying different classification models.
Uploads
Papers by burhan rashid
incollections by burhan rashid