Papers by Annalina Caputo
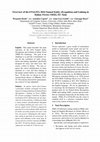
EVALITA. Evaluation of NLP and Speech Tools for Italian
English. This report describes the main outcomes of the 2016 Named Entity rEcognition and Linking... more English. This report describes the main outcomes of the 2016 Named Entity rEcognition and Linking in Italian Tweet (NEEL-IT) Challenge. The goal of the challenge is to provide a benchmark corpus for the evaluation of entity recognition and linking algorithms specifically designed for noisy and short texts, like tweets, written in Italian. The task requires the correct identification of entity mentions in a text and their linking to the proper named entities in a knowledge base. To this aim, we choose to use the canonicalized dataset of DBpedia 2015-10. The task has attracted five participants, for a total of 15 runs submitted. Italiano. In questo report descriviamo i principali risultati conseguiti nel primo task per la lingua Italiana di Named Entity rEcognition e Linking in Tweet (NEEL-IT). Il task si prefigge l'obiettivo di offrire un framework di valutazione per gli algoritmi di riconoscimento e linking di entità a nome proprio specificamente disegnati per la lingua italiana per testi corti e rumorosi, quali i tweet. Il task si compone di una fase di riconoscimento delle menzioni di entità con nome proprio nel testo e del loro successivo collegamento alle opportune entità in una base di conoscenza. In questo task abbiamo scelto come base di conoscenza la versione canonica di DBpedia 2015. Il task ha attirato cinque partecipanti per un totale di 15 diversi run.
Proceedings of the Fourteenth Workshop on Semantic Evaluation, 2020
This paper describes the system proposed for the SemEval-2020 Task 1: Unsupervised Lexical Semant... more This paper describes the system proposed for the SemEval-2020 Task 1: Unsupervised Lexical Semantic Change Detection. We focused our approach on the detection problem. Given the semantics of words captured by temporal word embeddings in different time periods, we investigate the use of unsupervised methods to detect when the target word has gained or loosed senses. To this end, we defined a new algorithm based on Gaussian Mixture Models to cluster the target similarities computed over the two periods. We compared the proposed approach with a number of similarity-based thresholds. We found that, although the performance of the detection methods varies across the word embedding algorithms, the combination of Gaussian Mixture with Temporal Referencing resulted in our best system.
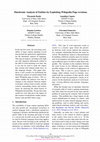
In the last few years, the increasing availability of large corpora spanning several time periods... more In the last few years, the increasing availability of large corpora spanning several time periods has opened new opportunities for the diachronic analysis of language. This type of analysis can bring to the light not only linguistic phenomena related to the shift of word meanings over time, but it can also be used to study the impact that societal and cultural trends have on this language change. This paper introduces a new resource for performing the diachronic analysis of named entities built upon Wikipedia page revisions. This resource enables the analysis over time of changes in the relations between entities (concepts), surface forms (words), and the contexts surrounding entities and surface forms, by analysing the whole history of Wikipedia internal links. We provide some useful use cases that prove the impact of this resource on diachronic studies and delineate some possible future usage.
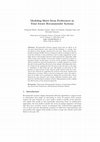
Recommender Systems suggest items that are likely to be the most interesting for users, based on ... more Recommender Systems suggest items that are likely to be the most interesting for users, based on the feedback, i.e. ratings, they provided on items already experienced in the past. Time-aware Recommender Systems (TARS) focus on temporal context of ratings in order to track the evolution of user preferences and to adapt suggestions accordingly. In fact, some people’s interests tend to persist for a long time, while others change more quickly, because they might be related to volatile information needs. In this paper, we focus on the problem of building an effective profile for short-term preferences. A simple approach is to learn the short-term model from the most recent ratings, discarding older data. It is based on the assumption that the more recent the data is, the more it contributes to find items the user will shortly be interested in. We propose an improvement of this classical model, which tracks the evolution of user interests by exploiting the content of the items, besides ...
The availability of data spanning different epochs has inspired a new analysis of cultural, socia... more The availability of data spanning different epochs has inspired a new analysis of cultural, social, and linguistic phenomena from a temporal perspective. This paper describes the application of Temporal Random Indexing (TRI) to the news context. TRI is able to build geometrical spaces of word meanings that consider several periods of time. Hence, TRI enables the analysis of the evolution in time of the meaning of a word. We propose some examples of application of TRI to the analysis of word meanings in the news scenario; this analysis enables the detection of linguistic variations that emerge in specific time intervals and that can be related to particular events.
The use of automatic methods for the study of lexical semantic change (LSC) has led to the creati... more The use of automatic methods for the study of lexical semantic change (LSC) has led to the creation of evaluation benchmarks. Benchmark datasets, however, are intimately tied to the corpus used for their creation questioning their reliability as well as the robustness of automatic methods. This contribution investigates these aspects showing the impact of unforeseen social and cultural dimensions. We also identify a set of additional issues (OCR quality, named entities) that impact the performance of the automatic methods, especially when used to discover LSC.
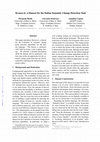
This paper introduces Kronos-it, a dataset for the evaluation of semantic change point detection ... more This paper introduces Kronos-it, a dataset for the evaluation of semantic change point detection algorithms for the Italian language. The dataset is automatically built by using a web scraping strategy. We provide a detailed description about the dataset and its generation, and four state-of-the-art approaches for the semantic change point detection are benchmarked by exploiting the Italian Google ngrams corpus. 1 Background and Motivation Computational approaches to the problem of language change have been gaining momentum over the last decade. The availability of long-term and large-scale digital corpora, and the effectiveness of methods for representing words over time, are the prerequisite behind this interest. However, only few attempts have focused on the evaluation, due to two main issues. First, the amount of data involved limits the possibility to perform a manual evaluation and, secondly, to date no open dataset for the diachronic semantic change has been made available. T...

Conversational Recommender Systems assist online users in their information-seeking and decision ... more Conversational Recommender Systems assist online users in their information-seeking and decision making tasks by supporting an interactive process with the aim of finding the most appealing items according to the user preferences. Unfortunately, collecting dialogues data to train these systems can be labour-intensive, especially for data-hungry Deep Learning models. Therefore, we propose an automatic procedure able to generate plausible dialogues from recommender systems datasets. People have information needs of varying complexity, which can be solved by an intelligent agent able to answer questions formulated in a proper way, eventually considering user context and preferences. Conversational Recommender Systems (CRS) assist online users in their information-seeking and decision making tasks by supporting an interactive process [1] with the aim of finding the most appealing items according to the user preferences. Unfortunately, collecting dialogues data required for the training ...
EVALITA Evaluation of NLP and Speech Tools for Italian - December 17th, 2020, 2020
English. This paper describes the first edition of the "Diachronic Lexical Semantics" (DIACR-Ita)... more English. This paper describes the first edition of the "Diachronic Lexical Semantics" (DIACR-Ita) task at the EVALITA 2020 campaign. The task challenges participants to develop systems that can automatically detect if a given word has changed its meaning over time, given contextual information from corpora. The task, at its first edition, attracted 9 participant teams and collected a total of 36 submission runs.
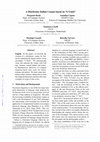
Proceedings of the Seventh Italian Conference on Computational Linguistics CLiC-it 2020, 2020
English. In this paper, we describe the creation of a diachronic corpus for Italian by exploiting... more English. In this paper, we describe the creation of a diachronic corpus for Italian by exploiting the digital archive of the newspaper "L'Unità". We automatically clean and annotate the corpus with PoS tags, lemmas, named entities and syntactic dependencies. Moreover, we compute frequency-based time series for tokens, lemmas and entities. We show some interesting corpus statistics taking into account the temporal dimension and describe some examples of usage of time series. 1 Motivation and Background Diachronic linguistics is one of the two major temporal dimensions of language study proposed by de Saussure in his Cours de languistique générale and has a long tradition in Linguistics. Recently, the increasing availability of diachronic corpora as well as the development of new NLP techniques for representing word meanings has boosted the application of computational models to investigate historical language data (Hamilton et al., 2016; Tahmasebi et al., 2018; Tang, 2018). This culminated in SemEval-2020 Unsupervised Lexical Semantic Change Detection (Schlechtweg et al., 2020), the first attempt to systematically evaluate automatic methods for language change detection. Italian is a Romance language which has undergone lots of changes in its history. Its official
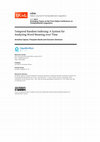
Italian Journal of Computational Linguistics, 2015
During the last decade the surge in available data spanning different epochs has inspired a new a... more During the last decade the surge in available data spanning different epochs has inspired a new analysis of cultural, social, and linguistic phenomena from a temporal perspective. This paper describes a method that enables the analysis of the time evolution of the meaning of a word. We propose Temporal Random Indexing (TRI), a method for building WordSpaces that takes into account temporal information. We exploit this methodology in order to build geometrical spaces of word meanings that consider several periods of time. The TRI framework provides all the necessary tools to build WordSpaces over different time periods and perform such temporal linguistic analysis. We propose some examples of usage of our tool by analysing word meanings in two corpora: a collection of Italian books and English scientific papers about computational linguistics. This analysis enables the detection of linguistic events that emerge in specific time intervals and that can be related to social or cultural phenomena.

International Journal of Electronic Governance, 2017
This paper introduces a semantic and personalised information retrieval (SEPIR) tool for the publ... more This paper introduces a semantic and personalised information retrieval (SEPIR) tool for the public administration of Apulia Region. SEPIR, through semantic search and visualisation tools, enables the analysis of a large amount of unstructured data and the intelligent access to information. At the core of these functionalities is an NLP pipeline responsible for the WordSpace building and the key-phrase extraction. The WordSpace is the key component of the semantic search and personalisation algorithm. Moreover, key-phrases enrich the document representation of the retrieval system and are on the basis of the bubble charts, which provide a quick overview of the main concepts involved in a document collection.We show some of the key features of SEPIR in a use case where the personalisation technique re-ranks the set of relevant documents on the basis of the user's past queries and the visualisation tools provide the users with useful information about the analysed collection.
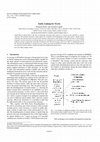
Encyclopedia with Semantic Computing and Robotic Intelligence, 2017
Named Entity Linking (NEL) is the task of semantically annotating entity mentions in a portion of... more Named Entity Linking (NEL) is the task of semantically annotating entity mentions in a portion of text with links to a knowledge base. The automatic annotation, which requires the recognition and disambiguation of the entity mention, usually exploits contextual clues like the context of usage and the coherence with respect to other entities. In Twitter, the limits of 140 characters originates very short and noisy text messages that pose new challenges to the entity linking task. We propose an overview of NEL methods focusing on approaches specifically developed to deal with short messages, like tweets. NEL is a fundamental task for the extraction and annotation of concepts in tweets, which is necessary for making the Twitter’s huge amount of interconnected user-generated contents machine readable and enable the intelligent information access.
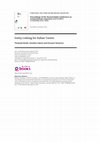
Proceedings of the Second Italian Conference on Computational Linguistics CLiC-it 2015
English. Linking entity mentions in Italian tweets to concepts in a knowledge base is a challengi... more English. Linking entity mentions in Italian tweets to concepts in a knowledge base is a challenging task, due to the short and noisy nature of these short messages and the lack of specific resources for Italian. This paper proposes an adaptation of a general purpose Named Entity Linking algorithm, which exploits the similarity measure computed over a Distributional Semantic Model, in the context of Italian tweets. In order to evaluate the proposed algorithm, we introduce a new dataset of tweets for entity linking that we developed specifically for the Italian language. Italiano. La creazione di collegamenti tra le menzioni di un'entità in tweet in italiano ed il corrispettivo concetto in una base di conoscenzaè un compito problematico a causa del testo nei tweet, generalmente corto e rumoroso, e della mancanza di risorse specifiche per l'italiano. In questo studio proponiamo l'adattamento di un algoritmo generico di Named Entity Linking, che sfrutta la misura di similarità semantica calcolata su uno spazio distribuzionale, nel contesto dei tweet in Italiano. Al fine di valutare l'algoritmo proposto, inoltre, introduciamo un nuovo dataset di tweet per il task di entity linking specifico per la lingua italiana.
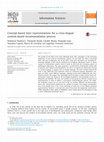
Information Sciences, 2016
The growth of the Web is the most influential factor that contributes to the increasing importanc... more The growth of the Web is the most influential factor that contributes to the increasing importance of text retrieval and filtering systems. On one hand, the Web is becoming more and more multilingual, and on the other hand users themselves are becoming increasingly polyglot. In this context, platforms for intelligent information access as search engines or recommender systems need to evolve to deal with this increasing amount of multilingual information. This paper proposes a content-based recommender system able to generate cross-lingual recommendations. The idea is to exploit user preferences learned in a given language, to suggest item in another language. The main intuition behind the work is that, differently from keywords which are inherently language dependent, concepts are stable across different languages, allowing to deal with multilingual and crosslingual scenarios. We propose four knowledge-based strategies to build concept-based representation of items, by relying on the knowledge contained in two knowledge sources, i.e. Wikipedia and BabelNet. We learn user profiles by leveraging the different concept-based representations, in order to define a cross-lingual recommendation process. The empirical evaluation carried out on two state of the art datasets, DBbook and Movielens , shows that concept-based approaches are suitable to provide cross-lingual recommendations, even though there is not a clear advantage of using one of the different proposed representations. However, it emerges that most of the times the approaches based on Ba-belNet outperform those based on Wikipedia, which clearly shows the advantage of using a native multilingual knowledge source.
Information Filtering and Retrieval, 2016
Abstract. In this work, we propose a method for document re-ranking, which exploits negative feed... more Abstract. In this work, we propose a method for document re-ranking, which exploits negative feedback represented by non-relevant documents. The concept of non-relevance is modelled through the quantum negation operator. The evaluation carried out on a standard collection shows the effectiveness of the proposed method in both the classical Vector Space Model and a Semantic Document Space.
Abstract. Distributional approaches are based on a simple hypothesis: the meaning of a word can b... more Abstract. Distributional approaches are based on a simple hypothesis: the meaning of a word can be inferred from its usage. The application of that idea to the vector space model makes possible the construction of a WordSpace in which words are represented by mathematical points in a geometric space. Similar words are represented close in this space and the definition of “word usage” depends on the definition of the context used to build the space, which can be the whole document, the sentence in which the word occurs, a fixed ...
We report the results of UNIBA participation in the first SemEval-2012 Semantic Textual Similarit... more We report the results of UNIBA participation in the first SemEval-2012 Semantic Textual Similarity task. Our systems rely on distributional models of words automatically inferred from a large corpus. We exploit three different semantic word spaces: Random Indexing (RI), Latent Semantic Analysis (LSA) over RI, and vector permutations in RI. Runs based on these spaces consistently outperform the baseline on the proposed datasets.
Communications of SIWN (formerly: System and Information Sciences Notes), special issue on DART, Aug 1, 2008
Uploads
Papers by Annalina Caputo