Papers by Mahendra Piraveenan

Influence models enable the modelling of the spread of ideas, opinions and behaviours in social n... more Influence models enable the modelling of the spread of ideas, opinions and behaviours in social networks. Bounded rationality in social networks suggests that players make non-optimum decisions due to the limitations of access to information. Based on the premise that adopting a state or an idea can be regarded as being 'ra-tional', we propose an influence model based on the heterogeneous bounded rationality of players in a social network. We employ the quantal response equilibrium model to incorporate the bounded rationality in the context of social influence. We hypothesise that bounded rationality of following a seed or adopting the strategy of a seed is negatively proportional to the distance from that node, and it follows that closeness centrality is the appropriate measure to place influencers in a social network. We argue that this model can be used in scenarios where there are multiple types of influencers and varying pay-offs of adopting a state. We compare different seed placement mechanisms to compare and contrast the optimum method to minimise the existing social influence in a network when there are multiple and conflicting seeds. We ascertain that placing of opposing seeds according to a measure derived from a combination of the betweenness centrality values from the seeds, and the closeness centrality of the network provide the maximum negative influence. Further, we extend this model to a strategic decision-making scenario where each seed operates a strategy in a strategic game.

A number of centrality measures are available to determine the relative importance of a node in a... more A number of centrality measures are available to determine the relative importance of a node in a complex network, and betweenness is prominent among them. However, the existing centrality measures are not adequate in network percolation scenarios (such as during infection transmission in a social network of individuals, spreading of computer viruses on computer networks, or transmission of disease over a network of towns) because they do not account for the changing percolation states of individual nodes. We propose a new measure, percolation centrality, that quantifies relative impact of nodes based on their topological connectivity, as well as their percolation states. The measure can be extended to include random walk based definitions, and its computational complexity is shown to be of the same order as that of betweenness centrality. We demonstrate the usage of percolation centrality by applying it to a canonical network as well as simulated and real world scale-free and random networks.

Game theory has long been used to model cognitive decision making in societies. While traditional... more Game theory has long been used to model cognitive decision making in societies. While traditional game theoretic modelling has focussed on well-mixed populations, recent research has suggested that the topological structure of social networks play an important part in the dynamic behaviour of social systems. Any agent or person playing a game employs a strategy (pure or mixed) to optimise pay-off. Previous studies have analysed how selfish agents can optimise their payoffs by choosing particular strategies within a social network model. In this paper we ask the question that, if agents were to work towards the common goal of increasing the public good (that is, the total network utility), what strategies they should adapt within the context of a heterogeneous network. We consider a number of classical and recently demonstrated game theoretic strategies, including cooperation , defection, general cooperation, Pavlov, and zero-determinant strategies, and compare them pairwise. We use the Iterative Prisoners Dilemma game simulated on scale-free networks, and use a genetic-algorithmic approach to investigate what optimal placement patterns evolve in terms of strategy. In particular, we ask the question that, given a pair of strategies are present in a network, which strategy should be adopted by the hubs (highly connected people),

It is well known that non-vaccinated individuals may be protected from contacting a disease by va... more It is well known that non-vaccinated individuals may be protected from contacting a disease by vaccinated individuals in a social network through community protection (herd immunity). Such protection greatly depends on the underlying topology of the social network, the strategy used in selecting individuals for vaccination, and the interplay between these. In this paper, we analyse how the interplay between topology and immunization strategies influences the herd immunity of social networks. First, we introduce an area under curve measure which can quantify the levels of herd immunity in a social network. Then, using this measure, we analyse the above mentioned interplay in three ways: (1) by comparing vaccination strategies across topologies, (2) by analysing the influence of selected topological metrics, and (3) by considering the influence of network growth on herd immunity. For qualitative comparison, we consider three classical topologies (scale-free, random, and small-world) and three vaccination strategies (natural, random, and betweenness-based immunization). We show that betweenness-based vaccination is the best strategy of immunization in static networks , regardless of topology, but its prominence over other strategies diminishes in dynamically growing topologies. We find that the network features that lead to 'small-worldness' in networks (low diameter and high clustering) discourage herd immunity, regardless of the vaccination strategy, while preferential mixing (high as-sortativity) encourages it. In terms of growth, we demonstrate that herd immunity of random networks actually increases with growth, if the proportion of survivors to a secondary infection is considered, while the community protection in scale-free and small-world networks decreases with growth. Our work highlights the complex balance between social network structure and vaccination strategies in influencing community protection, and contributes a numerical measure to quantify this. Keywords Complex systems Á Structures and organization in complex systems Á Systems obeying scaling laws Á Graph theory Á Networks and genealogical trees

The indices currently used by scholarly databases, such as Google scholar, to rank scientists, do... more The indices currently used by scholarly databases, such as Google scholar, to rank scientists, do not attach weights to the citations. Neither is the underlying network structure of citations considered in computing these metrics. This results in scientists cited by well-recognized journals not being rewarded, and may lead to potential misuse if documents are created purely to cite others. In this paper we introduce a new ranking metric, the p-index (pagerank-index), which is computed from the underlying citation network of papers, and uses the pagerank algorithm in its computation. The index is a percentile score, and can potentially be implemented in public databases such as Google scholar, and can be applied at many levels of abstraction. We demonstrate that the metric aids in fairer ranking of scientists compared to h-index and its variants. We do this by simulating a realistic model of the evolution of citation and collaboration networks in a particular field, and comparing h-index and p-index of scientists under a number of scenarios. Our results show that the p-index is immune to author behaviors that can result in artificially bloated h-index values.

In this paper, we introduce a measure to analyse the structural robustness of complex networks, w... more In this paper, we introduce a measure to analyse the structural robustness of complex networks, which is specifically applicable in scenarios of targeted, sustained attacks. The measure is based on the changing size of the largest component as the network goes through disintegration. We argue that the measure can be used to quantify and compare the effectiveness of various attack strategies. Applying this measure, we confirm the result that scale-free networks are comparatively less vulnerable to random attacks and more vulnerable to targeted attacks. Then we analyse the robustness of a range of real world networks, and show that most real world networks are least robust to attacks based on betweenness of nodes. We also show that the robustness values of some networks are more sensitive to the attack strategy as compared to others. Furthermore, robustness coefficient computed using two centrality measures may be similar, even when the computational complexities of calculating these centrality measures may be different. Given this disparity, the robustness coefficient introduced potentially plays a key role in choosing attack and defence strategies for real world networks. While the measure is applicable to all types of complex networks, we clearly demonstrate its relevance to social network analysis.

Quantifying and comparing the scientific output of researchers has become critical for government... more Quantifying and comparing the scientific output of researchers has become critical for governments , funding agencies and universities. Comparison by reputation and direct assessment of contributions to the field is no longer possible, as the number of scientists increases and traditional definitions about scientific fields become blurred. The h-index is often used for comparing scientists, but has several well-documented shortcomings. In this paper, we introduce a new index for measuring and comparing the publication records of scientists: the pagerank-index (symbolised as π). The index uses a version of pagerank algorithm and the citation networks of papers in its computation, and is fundamentally different from the existing variants of h-index because it considers not only the number of citations but also the actual impact of each citation. We adapt two approaches to demonstrate the utility of the new index. Firstly, we use a simulation model of a community of authors, whereby we create various 'groups' of authors which are different from each other in inherent publication habits, to show that the pagerank-index is fairer than the existing indices in three distinct scenarios: (i) when authors try to 'massage' their index by publishing papers in low-quality outlets primarily to self-cite other papers (ii) when authors collaborate in large groups in order to obtain more authorships (iii) when authors spend most of their time in producing genuine but low quality publications that would massage their index. Secondly, we undertake two real world case studies: (i) the evolving author community of quantum game theory, as defined by Google Scholar (ii) a snapshot of the high energy physics (HEP) theory research community in arXiv. In both case studies, we find that the list of top authors vary very significantly when h-index and pagerank-index are used for comparison. We show that in both cases, authors who have collaborated in large groups and/or published less impactful papers tend to be comparatively favoured by the h-index, whereas the pagerank-index highlights authors who have made a relatively small number of definitive contributions, or written papers which served to highlight the link between diverse disciplines, or typically worked in smaller

Evolutionary game theory is used to model the evolution of competing strategies in a population o... more Evolutionary game theory is used to model the evolution of competing strategies in a population of players. Evolutionary stability of a strategy is a dynamic equilibrium, in which any competing mutated strategy would be wiped out from a population. If a strategy is weak evolutionarily stable, the competing strategy may manage to survive within the network. Understanding the network-related factors that affect the evolutionary stability of a strategy would be critical in making accurate predictions about the behaviour of a strategy in a real-world strategic decision making environment. In this work, we evaluate the effect of network topology on the evolutionary stability of a strategy. We focus on two well-known strategies known as the Zero-determinant strategy and the Pavlov strategy. Zero-determinant strategies have been shown to be evolutionarily unstable in a well-mixed population of players. We identify that the Zero-determinant strategy may survive, and may even dominate in a population of players connected through a non-homogeneous network. We introduce the concept of 'topological stability' to denote this phenomenon. We argue that not only the network topology, but also the evolutionary process applied and the initial distribution of strategies are critical in determining the evolutionary stability of strategies. Further, we observe that topological stability could affect other well-known strategies as well, such as the general cooperator strategy and the cooperator strategy. Our observations suggest that the variation of evolutionary stability due to topological stability of strategies may be more prevalent in the social context of strategic evolution, in comparison to the biological context.

This paper describes a complex networks approach to study the failure tolerance of mechatronic so... more This paper describes a complex networks approach to study the failure tolerance of mechatronic software systems under various types of hardware and/or software failures. We produce synthetic system architectures based on evidence of modular and hierarchical modular product architectures and known motifs for the interconnection of physical components to software. The system architectures are then subject to various forms of attack. The attacks simulate failure of critical hardware or software. Four types of attack are investigated: degree centrality, betweenness centrality, closeness centrality and random attack. Failure tolerance of the system is measured by a 'robustness coefficient', a topological 'size' metric of the connectedness of the attacked network. We find that the betweenness centrality attack results in the most significant reduction in the robustness coefficient, confirming betweenness centrality, rather than the number of connections (i.e. degree), as the most conservative metric of component importance. A counter-intuitive finding is that " designed " system architectures, including a bus, ring, and star architecture, are not significantly more failure-tolerant than interconnections with no prescribed architecture, that is, a random architecture. Our research provides a data-driven approach to engineer the architecture of mechatronic software systems for failure tolerance .

Assortativity quantifies the tendency of nodes being connected to similar nodes in a complex netw... more Assortativity quantifies the tendency of nodes being connected to similar nodes in a complex network. Degree Assortativity can be quantified as a Pearson correlation. However, it is insufficient to explain assortative or disassortative tendencies of individual nodes or links, which may be contrary to the overall tendency of the network. A number of 'local' assortativity measures have been proposed to address this. In this paper we define and analyse an alternative formulation for node assortativity, primarily for undirected networks. The alternative approach is justified by some inherent shortcomings of existing local measures of assortativity. Using this approach, we show that most real world scale-free networks have disassortative hubs, though we can synthesise model networks which have assortative hubs. Highlighting the relationship between assortativity of the hubs and network robustness, we show that real world networks do display assortative hubs in some instances, particularly when high robustness to targeted attacks is a necessity.

In networked systems research, game theory is increasingly used to model a number of scenarios wh... more In networked systems research, game theory is increasingly used to model a number of scenarios where distributed decision making takes place in a competitive environment. These scenarios include peer-to-peer network formation and routing, computer security level allocation, and TCP congestion control. It has been shown, however, that such modeling has met with limited success in capturing the real-world behavior of computing systems. One of the main reasons for this drawback is that, whereas classical game theory assumes perfect rationality of players, real world entities in such settings have limited information, and cognitive ability which hinders their decision making. Meanwhile , new bounded rationality models have been proposed in networked game theory which take into account the topology of the network. In this article, we demonstrate that game-theoretic modeling of computing systems would be much more accurate if a topologically distributed bounded rationality model is used. In particular, we consider (a) link formation on peer-to-peer overlay networks (b) assigning security levels to computers in computer networks (c) routing in peer-to-peer overlay networks, and show that in each of these scenarios, the accuracy of the modeling improves very significantly when topological models of bounded rationality are applied in the modeling process. Our results indicate that it is possible to use game theory to model competitive scenarios in networked systems in a way that closely reflects real world behavior, topology, and dynamics of such systems. V

Influence models enable the modelling of the spread of ideas, opinions and behaviours in social n... more Influence models enable the modelling of the spread of ideas, opinions and behaviours in social networks. Bounded rationality in social networks suggests that players make non-optimum decisions due to the limitations of access to information. Based on the premise that adopting a state or an idea can be regarded as being 'ra-tional', we propose an influence model based on the heterogeneous bounded rationality of players in a social network. We employ the quantal response equilibrium model to incorporate the bounded rationality in the context of social influence. We hypothesise that bounded rationality of following a seed or adopting the strategy of a seed is negatively proportional to the distance from that node, and it follows that closeness centrality is the appropriate measure to place influencers in a social network. We argue that this model can be used in scenarios where there are multiple types of influencers and varying pay-offs of adopting a state. We compare different seed placement mechanisms to compare and contrast the optimum method to minimise the existing social influence in a network when there are multiple and conflicting seeds. We ascertain that placing of opposing seeds according to a measure derived from a combination of the betweenness centrality values from the seeds, and the closeness centrality of the network provide the maximum negative influence. Further, we extend this model to a strategic decision-making scenario where each seed operates a strategy in a strategic game.

Several growth models have been proposed in the literature for scale-free complex networks, with ... more Several growth models have been proposed in the literature for scale-free complex networks, with a range of fitness-based attachment models gaining prominence recently. However, the processes by which such fitness-based attachment behaviour can arise are less well understood, making it difficult to compare the relative merits of such models. This paper analyses an evolutionary mechanism that would give rise to a fitness-based attachment process. In particular, it is proven by analytical and numerical methods that in homogeneous networks, the minimisation of maximum exposure to network unfitness (defined as the inverse of the summation of node fitness values) leads to attachment probabilities that are proportional to node fitness. This result is then extended to heterogeneous networks, with supply chain networks being used as an example. INTRODUCTION Network analysis has emerged as an effective way of studying complex and distributed systems, because it often offers a fine balance between simplicity and realism in modelling such systems [1]. Preferential-attachment models, such as the Barabási-Albert model where attachment probabilities are proportional to target node degree, have been the most prominent among them in the past decade [2]. Recently, a number of node fitness-based attachment models [3, 4, 5, 6, 7, 8, 9, 10, 11, 12] have been gaining prominence. It is noted that the concept of node fitness in this context is parallel to the concept of utility, as used in discrete choice models, where utility is a dimensionless measure of attraction which can be expressed as a function of attributes weighted by their relative importance (for further information regarding the role of utility, refer to [13]). Similarly, node fitness is a dimensionless measure of the inherent competitive ability (or the 'attractiveness') that a node has, which influences the rate at which it acquires links from other nodes as the network evolves over time. One such attribute could be node degree, which if used results in the Barabási-Albert model, but other attributes of the node also could be considered individually or collectively. An important feature of networks that evolve through node-degree and node-fitness based attachment growth models is that they can exhibit scale free topology, which is found in abundance among biological, technical and social networks [6, 14]. It has been widely accepted that the node-fitness based attachment models (such as the Bianconi-Barabási model [4] or the Ghadge model [3]) are more realistic when compared to the classical Barabási-Albert model in capturing the growth process of real world networks [4, 6]. Nevertheless, the behavioural bases from which such fitness-based attachment behaviour would arise, and the evolutionary mechanisms which shape that behaviour, are less clearly understood. In particular, while many papers have offered intuitive and general explanations as to why increased fitness of a node would attract more links [3, 4, 5, 6], the proportionality between fitness and attachment probabilities assumed in these models is yet to be explained by a rigorous evolutionary framework. A chain is only as strong as its weakest link, goes an old adage. This paper proposes the minimisation of maximum exposure to least fit nodes (or the avoidance of the " weakest links ") as a behavioural basis for the fitness-based proportional attachment rule. To prove this hypothesis, we analyse complex networks where each node is assigned a fitness value, and attachments between nodes are based on node probabilities that minimise the maximum exposure to network unfitness, where unfitness of the network is defined as the inverse of the summation of node fitness values. Two cases are considered: 1. Homogeneous networks, where all nodes are of the same type so attachment is possible between any pair of nodes. Note that we use the word 'homogeneous' in this sense and not in the sense that the topology is homogeneous (e.g. a lattice) as is sometimes the case in literature [15, 16, 17]. Some real world examples of such networks could be social networks, both online and offline [16, 17, 18], contact networks in epidemiological studies where any individual can come into contact with any other individual [19, 20, 21, 22], or the World Wide Web (WWW) where any website could link to any other website [6, 16], to name a few. In fact, most real world social, biological, and technical networks studied in the literature will fall into this category, where link formation is not constrained by node type [6, 16]. In our behavioural model for homogeneous networks, attachments are based on node selection probabilities that minimise maximum exposure to the least fit nodes. 2. Heterogeneous networks, where nodes are distinguished by type, and only links between certain types are feasible. Networks characterised as bi-partite or k-partite graphs in the literature [16, 23] are heterogeneous networks in this sense. Some real world examples of such heterogeneous networks include collaboration networks where the actors and collaborative activities are both represented as nodes [24], genes and conditions both represented as nodes in microarray data, customers and items both represented as nodes in collaborative filtering [23], pollination networks where pollinators feed only on a subset of available flowers [25], or contact networks in illnesses that spread not from person to person directly but through a vector, such as dengue or malaria [26, 27]. The constraints on attachment in heterogeneous networks can take many forms, and these constraints are context-dependent. Some of these constraints are trivial. Therefore, rather than attempting to analyse all sub-types of heterogeneous networks that can possibly arise from such constraints, we choose here to focus on one interesting sub-type: tiered networks, where each tier contains nodes of a certain type unique to that tier, and from any given tier, only connections to/from the tier 'below' or 'above' are feasible. Hierarchical and multi-layer (tiered) networks have been much studied in recent years, both in terms of topology and in the context of networked games [28, 29, 30, 31, 32, 33], and networks of this type are frequently used to represent supply chains, where four tiers are commonly modelled, namely suppliers, manufacturers, distributers and retailers [34, 35]. Other real world examples for such tiered networks may include tiered bipartite graphs used in gene regulatory network analysis, where for example, a top layer of unobserved regulators can connect with a bottom layer of observed mRNA expression values [36], or food webs where each trophic level (producer, primary consumers, secondary consumers, etc) serves as a tier and connections are only permitted between adjacent tiers [37]. In tiered networks, feasible sets of nodes take the form of paths, so the problem is to find path selection probabilities that minimise maximum exposure to least fit nodes, and then base attachments on the path selection probabilities. The two cases are distinguished by the node types available and the constraints on link formation, not by resultant topology. Indeed, fitness-based proportional attachment models have been used to model each case, which is why we study them, and each case can result in scale-free topologies with power-law degree distributions [3, 4, 5, 6, 15, 16, 17, 18, 23, 25, 34, 35]. In particular, tiered networks such as supply chain networks are often scale-free with power-law degree distributions [38, 39], even though the tiered visualisation often obscures this. For each case (homogeneous networks and heterogeneous networks), we formulate a linear program, which minimises the maximum exposure to network unfitness. By solving the linear programs, we prove (analytically and numerically) that, when network unfitness is defined as inverse of the summation of node fitness, minimising maximum expected network unfitness leads to a node attachment probability that is proportional to the node fitness. Rearrangement of the corresponding Lagrangian equations reveals an equivalent mini-max problem [40], which has a useful game theoretic interpretation, as we will show[41]. An iterative solution process, which mimics a mixed-strategy, non-cooperative, two-player, zero-sum game, is then presented for each case, with convergence to equilibrium proven. For the case of path selection, the inequality constraints in the linear program generate dual variables, which convert the mini-max problem into a shortest path problem, thereby avoiding full set or path enumeration. We then implement this iterative solution process as a computer program and compute the attachment probabilities, which confirm our results obtained by direct solution of the linear program. We use networks of finite size in each numerical example. The iterative solution process describes an evolutionary mechanism whereby weaker nodes are progressively avoided. Therefore, we demonstrate that such a mechanism could result in attachment probabilities that are proportional to node fitness. BACKGROUND

Socio–ecological systems are increasingly modelled by games played on complex networks. While the... more Socio–ecological systems are increasingly modelled by games played on complex networks. While the concept of Nash equilibrium assumes perfect rationality, in reality players in such systems display heterogeneous bounded rationality. Here we present a topological model of bounded rationality in socio-ecological systems, using the rational-ity parameter of the Quantal Response Equilibrium. We argue that the system ratio-nality could be measured by the average Kullback-Leibler divergence between Nash and Quantal Response Equilibria, and that the convergence towards Nash equilibria on average corresponds to increased system rationality. Using this model, we show that when a randomly connected socio-ecological system is topologically optimised in order to converge towards Nash equilibria, scale-free and small world features emerge. Therefore, optimising system rationality is an evolutionary reason for the emergence of scale-free and small-world features in socio-ecological systems. Further, we show that in games where multiple equilibria are possible, the correlation between the scale-freeness of the system and the fraction of links with multiple equilibria goes through a rapid transi-1 tion when the average system rationality increases. Our results explain the influence of the topological structure of socio-ecological systems in shaping their collective cognitive behaviour, and provide an explanation for the prevalence of scale-free and small-world characteristics in such systems.
2014 Ieee Symposium on Evolving and Autonomous Learning Systems, Dec 1, 2014
2014 Ieee Symposium on Foundations of Computational Intelligence, Dec 1, 2014
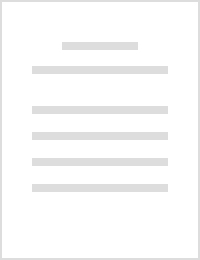
Computational and Mathematical Organization Theory, 2015
ABSTRACT Longitudinal networks evolve over time through the creation and/or deletion of links amo... more ABSTRACT Longitudinal networks evolve over time through the creation and/or deletion of links among a set of actors (e.g., individuals or organizations). A longitudinal network can be viewed as a single static network (i.e., structure of network is fixed) that aggregates all the edges observed over some time period or as a series of static networks observed in different point of time over the entire network observation period (i.e., structure of network is changing over time). The understanding of the underlying structural changes of longitudinal networks and contributions of individual actors to these changes enable researchers to investigate different structural properties of such networks. By following a topological approach (i.e., static topology and dynamic topology), this paper first proposes a framework to analyze longitudinal social networks. In static topology, social networks analysis (SNA) methods are applied to the aggregated network of entire observation period. Smaller segments of network data (i.e., short-interval network) that are accumulated in less time compared to the entire network observation period are used in the dynamic topology for analysis purposes. Based on this framework, this study then conducts topological analysis of two longitudinal networks to explore over time actor-level dynamics during different phases of these two networks. The proposed topological framework can be utilized to explore structural vulnerabilities and evolutionary trend of various longitudinal social networks (e.g., disease spread network and computer virus network). This will eventually lead to better authorization and control over such networks. For network science researchers, this framework will bring new research opportunities to enhance our present knowledge about different aspects (e.g., network disintegration and contribution of individual actor’s to network evolution) of longitudinal social networks.

Proceedings of the 2007 Annual Conference on International Conference on Computer Engineering and Applications, 2007
This paper describes our research in technology for the management and control of distributed ene... more This paper describes our research in technology for the management and control of distributed energy resource agents. An agent-based management and control system is being developed to enable largescale deployment of distributed energy resources. Local intelligent agents will allow consumers who are connected at low levels in the distribution network to manage their energy requirements and participate in coordinated responses to network stimuli. Such responses can be used to ease the volatility of wholesale electricity prices and assist constrained networks during summer and winter demand peaks. In our system, coordination of energy resources is decentralized. The coordination mechanism is asynchronous and adapts to change in an unsupervised manner, making it intrinsically scalable and robust.

In this paper, we explore the relationship between the topological characteristics of a complex n... more In this paper, we explore the relationship between the topological characteristics of a complex network and its robustness to sustained targeted attacks. Using synthesised scale-free, small-world and random networks, we look at a number of network measures, including assortativity, modularity, average path length, clustering coefficient, rich club profiles and scale-free exponent (where applicable) of a network, and how each of these influence the robustness of a network under targeted attacks. We use an established robustness coefficient to measure topological robustness, and consider sustained targeted attacks by order of node degree. With respect to scale-free networks, we show that assortativity, modularity and average path length have a positive correlation with network robustness, whereas clustering coefficient has a negative correlation. We did not find any correlation between scale-free exponent and robustness, or rich-club profiles and robustness. The robustness of small-world networks on the other hand, show substantial positive correlations with assortativity, modularity, clustering coefficient and average path length. In comparison, the robustness of Erdos-Renyi random networks did not have any significant correlation with any of the network properties considered. A significant observation is that high clustering decreases topological robustness in scale-free networks, yet it increases topological robustness in small-world networks. Our results highlight the importance of topological characteristics in influencing network robustness, and illustrate design strategies network designers can use to increase the robustness of scale-free and small-world networks under sustained targeted attacks.

Proceedings of the 2013 Ieee Acm International Conference on Advances in Social Networks Analysis and Mining, Aug 25, 2013
ABSTRACT We study evolution of coordination in social systems by simulating a coordination game i... more ABSTRACT We study evolution of coordination in social systems by simulating a coordination game in an ensemble of scale-free and small-world networks and comparing the results. We give particular emphasis to the role information about the pay-offs of neighbours plays in nodes adapting strategies, by limiting this information up to various levels. We find that if nodes have no chance to evolutionarily adapt, then non-coordination is a better strategy, however when nodes adapt based on information of the neighbour payoffs, coordination quickly emerges as the better strategy. We find phase transitions in number of coordinators with respect to the relative pay-off of coordination, and these phase transitions are sharper in small-world networks. We also find that when pay-off information of neighbours is limited, small-world networks are able to better cope with this limitation than scale-free networks. We observe that provincial hubs are the quickest to evolutionarily adapt strategies, in both scale-free and small world networks. Our findings confirm that evolutionary tendencies of coordination heavily depend on network topology.
Uploads
Papers by Mahendra Piraveenan