Papers by Laurent Cordier
Social Science Research Network, 2022
arXiv (Cornell University), Dec 28, 2022
Springer eBooks, Jul 3, 2007
ABSTRACT

HAL (Le Centre pour la Communication Scientifique Directe), Jul 19, 2022
The influence of especially energetic structures residing in the region around y + ≈ 4. √ Re τ , ... more The influence of especially energetic structures residing in the region around y + ≈ 4. √ Re τ , are investigated in channel flow at Re τ ≈ 5200. The motivation is to shed light on how the magnitude and length-scale properties of the smallscale near-wall turbulence are modulated by the outer structures, and whether this response is tied solely to the friction velocity-i.e., whether the near-wall turbulence is universal when scaled by the spatially and temporally varying largescale wall shear stress, rather than by its time-averaged value. A unique aspect of the present framework is the use of an autoencoder algorithm to separate the extremely large full-volume DNS fields into large-scale and small-scale motions. A second original element is a formalism that derives the modulation, conditional on large-scale fluctuations, from continuous statistical quantities represented as joint-multivariable probabilitydensity functions, thus obviating the need for any discrete representation or binning beyond that imposed by the discrete DNS solution. A third novel aspect is the use of the lengthscale-wise derivative of the second-order structure function to quantify the modulation (increase or decrease) in the length scale, again conditional on the large-scale structures.
This paper presents the control of a turbulent flow between two counter-rotating disks. A low-ord... more This paper presents the control of a turbulent flow between two counter-rotating disks. A low-order dynamical model is obtained by the use of a Proper Orthogonal Decomposition technique. The POD is performed for a nominal value of the Reynolds number. Control input is the disk rotation speed and modifies the Reynolds number. Hence, the dynamical model becomes uncertain but the extent of imprecisions are upper bounded. A sliding control methodology is considered to control the system on a limit cycle.

Frontiers in Physics, Dec 2, 2022
Editorial on the Research Topic Data-driven modeling and optimization in fluid dynamics: From phy... more Editorial on the Research Topic Data-driven modeling and optimization in fluid dynamics: From physicsbased to machine learning approaches Data-driven modeling has made a dramatic impact in computational science and engineering and, in particular, in computational fluid dynamics (CFD). One of the earliest uses of data in CFD is the proper orthogonal decomposition (POD), which was introduced by Lumley and his collaborators more than half a century ago. POD is based on a simple yet powerful idea: In the classical Galerkin framework (used in standard numerical methods, e.g., finite element or spectral methods), replace the general purpose basis functions with data-driven basis functions. This very simple idea has made a profound impact in CFD, reducing the computational cost of standard numerical methods by orders of magnitude and enabling challenging numerical simulations in shape optimization, flow control, and uncertainty quantification. Since Lumley's pioneering work, the field of data-driven modeling has witnessed a tremendous development. Probably the most exciting research area in this field is the use of machine learning. Over the last decade, the focus in data-driven modeling has shifted from physics-based strategies to machine learning approaches, in which instead of merely changing different components of classical methods (e.g., changing the basis in POD), one completely overhauls the entire framework (e.g., instead of using a Galerkin framework, one leverages machine learning algorithms to determine all the model operators). At this point, one natural question is which strategy should be used in CFD? Should one use physics-based or machine learning models? We believe that, as is often the case when discussing numerical methods, the truth is somewhere in the middle. That is, we believe that data-driven models that combine the physical and mathematical insight with machine learning strategies can revolutionize CFD and break new barriers in shape optimization, flow control, and uncertainty quantification. This Research Topic, which
Fluid mechanics and its applications, 1999
The active control of fully developed turbulent flows is of particular interest for many industri... more The active control of fully developed turbulent flows is of particular interest for many industrial applications. In such flow fields, the large-scale coherent structures contain most of the turbulent kinetic energy and are mainly responsible for vibrations, noise generation, etc… Therefore, in term of control, it seems important to describe correctly the characteristics of these structures and to predict precisely their time evolution using models as simple as possible. One of the methods proposed to mimic the dynamics of the flow is to develop low-order dynamical systems (Aubry et al., 1988) (Glauser et al., 1989) derived from the Proper Orthogonal Decomposition (POD) (Lumley, 1967; Sirovich, 1987).
HAL (Le Centre pour la Communication Scientifique Directe), 1996

Journal of Computational Physics, 2021
A sequential estimator based on the Ensemble Kalman Filter for Data Assimilation of fluid flows i... more A sequential estimator based on the Ensemble Kalman Filter for Data Assimilation of fluid flows is presented in this research work. The main feature of this estimator is that the Kalman filter update, which relies on the determination of the Kalman gain, is performed exploiting the algorithmic features of the numerical solver employed as a model. More precisely, the multilevel resolution associated with the multigrid iterative approach for time advancement is used to generate several low-resolution numerical simulations. These results are used as ensemble members to determine the correction via Kalman filter, which is then projected on the high-resolution grid to correct a single simulation which corresponds to the numerical model. The assessment of the method is performed via the analysis of one-dimensional and two-dimensional test cases, using different dynamic equations. The results show an efficient trade-off in terms of accuracy and computational costs required. In addition, a physical regularization of the flow, which is not granted by classical KF approaches, is naturally obtained owing to the multigrid iterative calculations. The algorithm is also well suited for the analysis of unsteady phenomena and, in particular, for potential application to in-streaming Data Assimilation techniques.

Computers & Fluids, 2018
Flow over open cavities is mainly governed by a feedback mechanism due to the interaction of shea... more Flow over open cavities is mainly governed by a feedback mechanism due to the interaction of shear layer instabilities and acoustic forcing propagating upstream in the cavity. This phenomenon is known to lead to resonant tones that can reach 180 dB in the far-field and may cause structural fatigue issues and annoying noise emission. This paper concerns the use of optimal control theory for reducing the noise level emitted by the cavity. Boundary control is introduced at the cavity upstream corner as a normal velocity component. Model-based optimal control of cavity noise involves multiple simulations of the compressible Navier-Stokes equations and its adjoint, which makes it a computationally expensive optimization approach. To reduce the computational costs, we propose to use a reduced-order model (ROM) based on Proper Orthogonal Decomposition (POD) as a surrogate model of the forward simulation. For that, a control input separation method is first used to introduce explicitly the control effect in the model. Then, an accurate and robust POD ROM is derived by using an optimization-based identification procedure and generalized POD modes, respectively. Since the POD modes describe only velocities and speed of sound, we minimize a noise-related cost functional characteristic of the total enthalpy unsteadiness. After optimizing the control function with the reduced-order model, we verify the optimality of the solution using the original, high-fidelity model. A maximum noise reduction of 4.7 dB is reached in the cavity and up to 16 dB at the far-field.

Journal of Computational Physics, Dec 1, 2022
Essential features of the Multigrid Ensemble Kalman Filter (G. Moldovan, G. Lehnasch, L. Cordier,... more Essential features of the Multigrid Ensemble Kalman Filter (G. Moldovan, G. Lehnasch, L. Cordier, M. Meldi, A multigrid/ensemble Kalman filter strategy for assimilation of unsteady flows, Journal of Computational Physics 443 − 110481) recently proposed for Data Assimilation of fluid flows are investigated and assessed in this article. The analysis is focused on the improvement in performance due to the inner loop. In this step, data from solutions calculated on the higher resolution levels of the multigrid approach are used as surrogate observations to improve the model prediction on the coarsest levels of the grid. The latter represents the level of resolution used to run the ensemble members for global Data Assimilation. The method is tested over two classical one-dimensional problems, namely the linear advection problem and the Burgers' equation. The analyses encompass a number of different aspects such as different grid resolutions. The results indicate that the contribution of the inner loop is essential in obtaining accurate flow reconstruction and global parametric optimization. These findings open exciting perspectives of application to grid-dependent reduced-order models extensively used in fluid mechanics applications for complex flows, such as Large Eddy Simulation (LES).

HAL (Le Centre pour la Communication Scientifique Directe), 2016
Many previous studies have shown that the turbulent mixing layer under periodic forcing tends to ... more Many previous studies have shown that the turbulent mixing layer under periodic forcing tends to adopt a lock-on state, where the major portion of the fluctuations in the flow are synchronized at the forcing frequency. The goal of this experimental study is to apply closed-loop control in order to provoke the lock-on state, using information from the flow itself. We aim to determine the range of frequencies for which the closed-loop control can establish the lock-on, and what mechanisms are contributing to the selection of a feedback frequency. In order to expand the solution space for optimal closed-loop control laws, we use the genetic programming control (GPC) framework. The best closed-loop control laws obtained by GPC are analysed along with the associated physical mechanisms in the mixing layer flow. The resulting closed-loop control significantly outperforms open-loop forcing in terms of robustness to changes in the free-stream velocities. In addition, the selection of feedback frequencies is not locked to the most amplified local mode, but rather a range of frequencies around it.

Ocean Engineering, Dec 1, 2022
Experiments on circular and square plate models were performed in a low-speed water tunnel at a f... more Experiments on circular and square plate models were performed in a low-speed water tunnel at a fixed Reynolds number [O (10 4)] for inclinations between 0 • and 90 • with respect to the flow direction. The experiments focus on drag force evolution and the associated flow structures to predict the drag regimes across the range of inclinations considered. Measurements show that drag increases rapidly with inclination for small angles, while at high angles it being nearly independent of inclination. The change between these drag regimes takes place at an inclination of 54 • , with hydrogen bubble and PIV flow visualizations indicating this to be accompanied by a change in the wake flow structure as well. Trailing vortices at low angles suggests the existence of induced drag, while shedding of shear layers from all around model circumference at high angles points to the dominance of pressure drag as in the case of bluff bodies.
HAL (Le Centre pour la Communication Scientifique Directe), Sep 8, 2019
73rd Annual Meeting of the APS Division of Fluid Dynamics, Nov 22, 2020
HAL (Le Centre pour la Communication Scientifique Directe), Jun 16, 2021
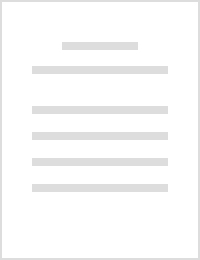
We investigate experimentally a novel model-free control strategy, called Machine Learning Contro... more We investigate experimentally a novel model-free control strategy, called Machine Learning Control (MLC), for aerodynamic drag reduction of a 3D bluff body. Fluidic actuation is applied at the blunt trailing edge of the body combined with a curved deflection surface. The impact of actuation on the flow is monitored with base pressure sensors. The applied model-free control strategy detects and exploits nonlinear actuation mechanisms in an unsupervised manner with the aim of minimizing the drag. Key enabler is linear genetic programming as simple and efficient framework for systems with multiple inputs (actuators) and multiple outputs (sensors). The ansatz of control laws include periodic forcing, multi-frequency forcing and sensor-based feedback control. Approximately 33% base pressure recovery is achieved by the optimal control law for a turbulent flow at Re_H ≈ 3 A 10 5 based on body height.

Les perturbations acoustiques présentes dans unécoulement de cavité bidimensionnel et compressibl... more Les perturbations acoustiques présentes dans unécoulement de cavité bidimensionnel et compressible sont analysées et contrôlées au moyen de simulations numériques directes (DNS) et d'une loi de rétroaction en retour de sortie liant l'actionneur de type jet synthétiqueà des prises de pression pariétales. La loi de contrôle est obtenue en bâtissant un modèle réduit dynamique non linéaire de l'écoulement non contrôlé et forcé par une excitationà large spectre puis en menant uneétude de contrôle actif de type "Linear Quadratic Regulator". La loi de contrle implémentée dans les DNS conduità une réduction significative et globale desémissions acoustiques (jusqu'à 10 dB). Le contrôle reste efficace bien en dehors de la fenêtre temporelle d'observation qui a servià l'obtention du modèle réduit. L'approche continue d'amortir les perturbations pour un contrôle de moyenne amplitude induisant des effets non linéaires.

HAL (Le Centre pour la Communication Scientifique Directe), 2020
Model-based control strategies require a dynamical model that is sufficiently accurate and robust... more Model-based control strategies require a dynamical model that is sufficiently accurate and robust with respect to the variation of the control parameters. When this model can not be determined using first principle equations, then identification techniques are needed. In this work, we present a general framework for identifying the parameters of a POD reduced-order model. The model obtained directly by POD Galerkin projection of the N-S equations is, in general, not robust. Here, we obtain a scalable identification of the parameters by a combined implementation of machine learning and data assimilation (DA) approaches. Recent advances in data driven techniques have given the possibility to learn the driving partial differential equations by using neural networks. However, without a partial knowledge of the underlying dynamics, the learning time may increase prohibitively with the number of parameters. To circumvent this difficulty, this work combines: i) PDE discovery methods to identify the parameters in the model, by using the physics-informed neural network 1 , and ii) Dual Ensemble Kalman filter 2 , a DA technique to correct both the predicted state and parameters.
Uploads
Papers by Laurent Cordier