Papers by CHAKOUR Chouaib
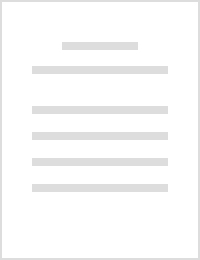
This paper presents a new adaptive kernel principal component analysis algorithm (AKPCA) for nonl... more This paper presents a new adaptive kernel principal component analysis algorithm (AKPCA) for nonlinear time-varying process monitoring. The basic idea is to use a neuronal principal component analysis based on the kernel version of the generalized Hebbian algorithm. The proposed algorithm follows a new methodology to update the KPCA model. At each time instant, when a new data is available, the KPCA model is updated accordingly without having
to re-explore all previous data. By using the proposed algorithm, the performance of process monitoring is improved in two aspects; the speed computation and adaptation of the KPCA model, and the storage memory complexity. To identify faults in a dynamic process, the
reconstruction based contribution approach is used and adapted in real time. The results for applying this algorithm on the Tennessee Eastman process shows its feasibility and advantageous performances.
Applied Mathematics & Information Sciences An International Journal
In this paper, a new algorithm for adaptive kernel principal component analysis (AKPCA) is propo... more In this paper, a new algorithm for adaptive kernel principal component analysis (AKPCA) is proposed for dynamic process monitoring. The proposed AKPCA algorithm combine two existing algorithms, the recursive weighted PCA (RWPCA) and the moving window kernel PCA algorithms. For fault detection and isolation, a set of structured residuals is generated by using a partial AKPCA models. Each partial AKPCA model is performed on subsets of variables. The structured residuals are utilized in composing an isolation scheme, according to a properly designed incidence matrix. The results for applying this algorithm on the nonlinear time varying processes of the Tennessee Eastman shows its feasibility and advantageous performances.
IEEE conference publication

On-line control using multivariate statistical methods has been widely used for largescale nonlin... more On-line control using multivariate statistical methods has been widely used for largescale nonlinear industrial processes monitoring. Kernel principal component analysis (KPCA) is a nonlinear monitoring method that cannot be employed for dynamic systems. The adaptive KPCA (AKPCA) is developed for nonlinear and time varying processes based on moving window KPCA (MWKPCA) and weighted PCA (WPCA) to update online the KPCA model and its corresponding control limits. In the proposed AKPCA the model of history data is used to build new model after the new sample is obtained. The results for applying this algorithm on the nonlinear time varying processes of the Tennessee Eastman benchmark shows its feasibility and advantageous performances. Key-words : adaptive kernel principal component analysis, multivariate statistical methods, nonlinear time-varying processes, moving window and weighted principle component analysis.

Dans ce article, nous présentons une approche statistique multi variée et non linéaire pour la mo... more Dans ce article, nous présentons une approche statistique multi variée et non linéaire pour la modélisation et le diagnostic des systèmes non linéaires. Cette approche, appelée analyse en composantes principales à noyau, est basée sur une transformation non linéaire des données en utilisant une fonction Noyau (Kernel). Cette transformation effectue un changement de base qui permet de projeter les données dans un nouvel espace où les relations entre les variables sont linéaires. La modélisation est ainsi facilitée, par l'application de l'ACP linéaire, puisque on passe d'un système initialement non linéaire, à un autre linéaire. Par contre l'espace de représentation sera de dimension plus importante que l'espace de départ. Cela peut générer un temps de calcul plus grand. Le principe de base de l'analyse en composantes principales à noyau, le principe de modélisation et la génération de résidus pour la détection et la localisation de défauts sont présentés. Les résultats de l'application de cette approche sur le benchmark Tennessee Eastman mettent en évidence les performances et l'intérêt de la méthode utilisée dans le domaine du diagnostic.

While principal component analysis (PCA) has found wide application in process monitoring, slow a... more While principal component analysis (PCA) has found wide application in process monitoring, slow and normal process changes often occur in real processes, which lead to false alarms for a fixed-model monitoring approach. In this paper, two recursive PCA algorithms for adaptive process monitoring are studied. the first algorithm is based on moving window "Moving Window PCA", and the second is based on forgetting factors "Recursive Weighted PCA". Furthermore, by changing the size and the shift of the window, also for the forgetting factor, we will see the influence of that change on the monitoring performance. Then, adaptive forgetting factors will be used, for increasing the robustness against outliers. Using the same concept of varying forgetting factors, we propose a new recursive algorithm for adaptive process monitoring based on moving window. By using the current model and the updated mean and covariance structures and an adaptive moving window, a new model is derived recursively. Based on the updated PCA representation, one monitoring metric, the Q-statistic (SPE) is calculated and their control limits are updated. The feasibility and advantages of each algorithms is illustrated by application to Tennessee Eastman process.

Dans cet article nous allons présenter une méthode statistique pour le diagnostic deséventuelles ... more Dans cet article nous allons présenter une méthode statistique pour le diagnostic deséventuelles défauts en temps réel fondu sur la combinaison de l'analyse en composantes principales et la transformée en ondelette. Ces approches sont potentiellement trés efficaces pour la détection et la localisation des défauts et des perturbations dans les processus. Nous présentons dans ce papier l'algorithme de base de modélisation par l'analyse en composantes principale multi-échelles (MSPCA : Multi Scale PCA) en temps déféré et en temps réel. Ainsi la MSPCA permet d'améliorer nettement la détection des défauts par rapportà l'ACP classique. L'approche MSPCA nous donne la possibilitè d'utilisé une méthode souple, simpleà mètre en ouvre pour la localisation des défauts, c'est la MSPCA partielle sans dégradation des signatures expérimentales. La MSPCA est utilisée pour la détection et la localisation des défauts, de tel sorte que les données mesurées sont décomposés en approximation et de détailsà différenteś echelles. Pour les systèmes dynamiques, l'adaptative MSPCA (utilisant une fenètre glissante) est développés pour mettreà jour la structure du modèle MSPCAà chaqueéchelle. Pour illustrer les approches proposés, les résultats de l'application de ces techniques sur le processus chimique Tennessee Eastman (TECP) seront présentés.
Neuronal Principal Component Analysis for Nonlinea by CHAKOUR Chouaib
Uploads
Papers by CHAKOUR Chouaib
to re-explore all previous data. By using the proposed algorithm, the performance of process monitoring is improved in two aspects; the speed computation and adaptation of the KPCA model, and the storage memory complexity. To identify faults in a dynamic process, the
reconstruction based contribution approach is used and adapted in real time. The results for applying this algorithm on the Tennessee Eastman process shows its feasibility and advantageous performances.
Neuronal Principal Component Analysis for Nonlinea by CHAKOUR Chouaib
to re-explore all previous data. By using the proposed algorithm, the performance of process monitoring is improved in two aspects; the speed computation and adaptation of the KPCA model, and the storage memory complexity. To identify faults in a dynamic process, the
reconstruction based contribution approach is used and adapted in real time. The results for applying this algorithm on the Tennessee Eastman process shows its feasibility and advantageous performances.