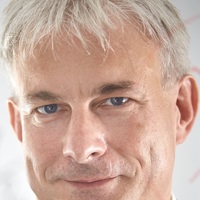
Stephan Hartmann
Stephan Hartmann is Dean of the Faculty of Philosophy, Philosophy of Science and the Study of Religion at LMU Munich, Alexander von Humboldt Professor, and Co-Director of the Munich Center for Mathematical Philosophy (MCMP). From 2007 to 2012 he worked at Tilburg University, The Netherlands, where he was Chair in Epistemology and Philosophy of Science and Director of the Tilburg Center for Logic and Philosophy of Science (TiLPS). Before moving to Tilburg, he was Professor of Philosophy in the Department of Philosophy, Logic and Scientific Method at the London School of Economics and Director of LSE's Centre for Philosophy of Natural and Social Science. He was President of the European Philosophy of Science Association (EPSA, 2013-2017) and President of the European Society for Analytic Philosophy (ESAP, 2014-2017). In 2016, he has been elected as a member of the German National Academy of Sciences, Leopoldina, and in 2019 he has been elected as a member of the Bavarian Academy for Sciences and Humanities. His primary research and teaching areas are philosophy of science, philosophy of physics, formal epistemology, social epistemology and (Bayesian) cognitive science. Hartmann published numerous articles and the books Bayesian Epistemology (with Luc Bovens, OUP 2003) and Bayesian Philosophy of Science (with Jan Sprenger, OUP 2019). His current research interests include the philosophy and psychology of reasoning and argumentation, the philosophy of physics (esp. the philosophy of open quantum systems and (imprecise) probabilities in quantum mechanics) and formal social epistemology (esp. models of deliberation and norm emergence).
Phone: +49 89 2180 3320
Address: Munich Center for Mathematical Philosophy
Ludwig-Maximilians-Universitat München
Ludwigstr. 31
80539 Munich
Germany
Phone: +49 89 2180 3320
Address: Munich Center for Mathematical Philosophy
Ludwig-Maximilians-Universitat München
Ludwigstr. 31
80539 Munich
Germany
less
InterestsView All (10)
Uploads
General Philosophy of Science by Stephan Hartmann
As a result, models have attracted philosophers’ attention and there are now sizable bodies of literature about various aspects of scientific modeling. A tangible result of philosophical engagement with models is a proliferation of model types recognized in the philosophical literature. Probing models, phenomenological models, computational models, developmental models, explanatory models, impoverished models, testing models, idealized models, theoretical models, scale models, heuristic models, caricature models, exploratory models, didactic models, fantasy models, minimal models, toy models, imaginary models, mathematical models, mechanistic models, substitute models, iconic models, formal models, analogue models, and instrumental models are but some of the notions that are used to categorize models. While at first glance this abundance is overwhelming, it can be brought under control by recognizing that these notions pertain to different problems that arise in connection with models. Models raise questions in semantics (how, if at all, do models represent?), ontology (what kind of things are models?), epistemology (how do we learn and explain with models?), and, of course, in other domains within philosophy of science.
famously thought to depend on whether y obtains in the most similar world(s) in which x obtains.
What this notion of ‘similarity’ consists in is controversial, but in recent years, graphical causal
models have proved incredibly useful in getting a handle on considerations of similarity between
worlds. One limitation of the resulting conception of similarity is that it says nothing about what
would obtain were the causal structure to be different from what it actually is, or from what we
believe it to be. In this paper, we explore the possibility of using graphical causal models to resolve
counterfactual queries about causal structure by introducing a notion of similarity between causal
graphs. Since there are multiple principled senses in which a graph G∗
can be more similar to a
graph G than a graph G∗∗, we introduce multiple similarity metrics, as well as multiple ways to
prioritize the various metrics when settling counterfactual queries about causal structure.
they are omnipresent across scientific disciplines, toy models are a surprisingly under-appreciated subject in the philosophy of science. The main
philosophical puzzle regarding toy models is that it is an unsettled question what the epistemic goal of toy modeling is. One promising proposal
for answering this question is the claim that the epistemic goal of toy models is to provide individual scientists with understanding. The aim of this
paper is to precisely articulate and to defend this claim. In particular, we
will distinguish between autonomous and embedded toy models, and, then,
argue that important examples of autonomous toy models are sometimes
best interpreted to provide how-possibly understanding, while embedded
toy models yield how-actually understanding, if certain conditions are satisfied.
therefore bound to fail. We demonstrate that Howson’s argument only
applies to one of two versions of the NMA. The other version, which
resembles the form in which the argument was initially presented by
Putnam and Boyd, remains unaffected by his line of reasoning. We
provide a formal reconstruction of that version of the NMA and show
that it is valid. Finally, we demonstrate that the use of subjective
priors is consistent with the realist implication of the NMA and show
that a core worry with respect to the suggested form of the NMA can
be dispelled.
a theory H, they often believe that there are not many distinct alternatives to H. But is this belief justified? What should scientists
believe about the number of alternatives to H, and how should they
change these beliefs in the light of new evidence? These are some of
the questions that we will address in this paper. We also ask under
which conditions failure to find an alternative to H confirms the theory
in question. This kind of reasoning (which we call the No Alternatives
Argument) is frequently used in science and therefore deserves a careful philosophical analysis.
bring them about. The new mechanistic philosophers have done much to substantiate this claim, and to provide us with a better understanding of what mechanisms are and how they explain. While there is disagreement among current mechanists on various issues, they share a common core position and a seeming commitment to some form of scientific realism. But is such a commitment necessary? Is it the best way to go about mechanistic explanation? In this paper, we propose an alternative antirealist account that also fits explanatory practice in the life sciences. We pay special attention to mechanistic models, i.e. scientific models that involve a mechanism, and to the role of coherence
considerations in building such models. To illustrate our points, we consider the
mechanism for the action potential.
bring them about. The new mechanistic philosophers have done much to substantiate this claim, and to provide us with a better understanding of what mechanisms are and how they explain. While there is disagreement among current mechanists on various issues, they share a common core position and a seeming commitment to some form of scientific realism. But is such a commitment necessary? Is it the best way to go about mechanistic explanation? In this paper, we propose an alternative antirealist account that also fits explanatory practice in the life sciences. We pay special attention to mechanistic models, i.e. scientific models that involve a mechanism, and to the role of coherence
considerations in building such models. To illustrate our points, we consider the
mechanism for the action potential.
As a result, models have attracted philosophers’ attention and there are now sizable bodies of literature about various aspects of scientific modeling. A tangible result of philosophical engagement with models is a proliferation of model types recognized in the philosophical literature. Probing models, phenomenological models, computational models, developmental models, explanatory models, impoverished models, testing models, idealized models, theoretical models, scale models, heuristic models, caricature models, exploratory models, didactic models, fantasy models, minimal models, toy models, imaginary models, mathematical models, mechanistic models, substitute models, iconic models, formal models, analogue models, and instrumental models are but some of the notions that are used to categorize models. While at first glance this abundance is overwhelming, it can be brought under control by recognizing that these notions pertain to different problems that arise in connection with models. Models raise questions in semantics (how, if at all, do models represent?), ontology (what kind of things are models?), epistemology (how do we learn and explain with models?), and, of course, in other domains within philosophy of science.
famously thought to depend on whether y obtains in the most similar world(s) in which x obtains.
What this notion of ‘similarity’ consists in is controversial, but in recent years, graphical causal
models have proved incredibly useful in getting a handle on considerations of similarity between
worlds. One limitation of the resulting conception of similarity is that it says nothing about what
would obtain were the causal structure to be different from what it actually is, or from what we
believe it to be. In this paper, we explore the possibility of using graphical causal models to resolve
counterfactual queries about causal structure by introducing a notion of similarity between causal
graphs. Since there are multiple principled senses in which a graph G∗
can be more similar to a
graph G than a graph G∗∗, we introduce multiple similarity metrics, as well as multiple ways to
prioritize the various metrics when settling counterfactual queries about causal structure.
they are omnipresent across scientific disciplines, toy models are a surprisingly under-appreciated subject in the philosophy of science. The main
philosophical puzzle regarding toy models is that it is an unsettled question what the epistemic goal of toy modeling is. One promising proposal
for answering this question is the claim that the epistemic goal of toy models is to provide individual scientists with understanding. The aim of this
paper is to precisely articulate and to defend this claim. In particular, we
will distinguish between autonomous and embedded toy models, and, then,
argue that important examples of autonomous toy models are sometimes
best interpreted to provide how-possibly understanding, while embedded
toy models yield how-actually understanding, if certain conditions are satisfied.
therefore bound to fail. We demonstrate that Howson’s argument only
applies to one of two versions of the NMA. The other version, which
resembles the form in which the argument was initially presented by
Putnam and Boyd, remains unaffected by his line of reasoning. We
provide a formal reconstruction of that version of the NMA and show
that it is valid. Finally, we demonstrate that the use of subjective
priors is consistent with the realist implication of the NMA and show
that a core worry with respect to the suggested form of the NMA can
be dispelled.
a theory H, they often believe that there are not many distinct alternatives to H. But is this belief justified? What should scientists
believe about the number of alternatives to H, and how should they
change these beliefs in the light of new evidence? These are some of
the questions that we will address in this paper. We also ask under
which conditions failure to find an alternative to H confirms the theory
in question. This kind of reasoning (which we call the No Alternatives
Argument) is frequently used in science and therefore deserves a careful philosophical analysis.
bring them about. The new mechanistic philosophers have done much to substantiate this claim, and to provide us with a better understanding of what mechanisms are and how they explain. While there is disagreement among current mechanists on various issues, they share a common core position and a seeming commitment to some form of scientific realism. But is such a commitment necessary? Is it the best way to go about mechanistic explanation? In this paper, we propose an alternative antirealist account that also fits explanatory practice in the life sciences. We pay special attention to mechanistic models, i.e. scientific models that involve a mechanism, and to the role of coherence
considerations in building such models. To illustrate our points, we consider the
mechanism for the action potential.
bring them about. The new mechanistic philosophers have done much to substantiate this claim, and to provide us with a better understanding of what mechanisms are and how they explain. While there is disagreement among current mechanists on various issues, they share a common core position and a seeming commitment to some form of scientific realism. But is such a commitment necessary? Is it the best way to go about mechanistic explanation? In this paper, we propose an alternative antirealist account that also fits explanatory practice in the life sciences. We pay special attention to mechanistic models, i.e. scientific models that involve a mechanism, and to the role of coherence
considerations in building such models. To illustrate our points, we consider the
mechanism for the action potential.
(IBE). More specifically, we present conditions under which explanatory considerations can provide a significant confirmatory boost for hypotheses that provide the best explanation of the relevant evidence. Furthermore, we show that the proposed Bayesian model of IBE is able to deal naturally with the best known criticisms of IBE such as van Fraassen’s ‘bad lot’ argument.
expressed simply in terms of a threshold degree of belief. In this context, we
examine the extent to which learning about possible alternatives may alter
one’s beliefs about a target hypothesis, even when no new ‘evidence’ linking
them to the hypothesis is acquired. Imagine the following scenario: a crime
has been committed and Alice, the police’s main suspect has been brought
to trial. There are several pieces of evidence that raise the probability
that Alice committed the crime. Her attorney’s defense strategy is not to
challenge this evidence, but instead to provide personal details about Alice’s
neighbour, Jane. While Jane is one of many people the police spoke to, they
saw no reason to investigate her further. You now learn that Jane, too, had
access to the shed where the murder weapon was stored, just like Alice. To
what extent should this alter your beliefs about Alice’s guilt? In this paper,
we provide a formal description of the problem and a solution indicating
circumstances under which learning about Jane will more or less impact
beliefs about Alice
high impact on the final decision, which we interpret as a new instance of the wellknown anchoring effect. To show this, we construct and analyze an agent-based model – inspired by the disagreement debate in social epistemology – and obtain analytical results for homogeneous groups (i.e., for groups whose members consider each other as epistemic peers) as well as simulation results for inhomogeneous groups.
expressed simply in terms of a threshold degree of belief. In this context, we
examine the extent to which learning about possible alternatives may alter
one’s beliefs about a target hypothesis, even when no new ‘evidence’ linking
them to the hypothesis is acquired. Imagine the following scenario: a crime
has been committed and Alice, the police’s main suspect has been brought
to trial. There are several pieces of evidence that raise the probability
that Alice committed the crime. Her attorney’s defense strategy is not to
challenge this evidence, but instead to provide personal details about Alice’s
neighbour, Jane. While Jane is one of many people the police spoke to, they
saw no reason to investigate her further. You now learn that Jane, too, had
access to the shed where the murder weapon was stored, just like Alice. To
what extent should this alter your beliefs about Alice’s guilt? In this paper,
we provide a formal description of the problem and a solution indicating
circumstances under which learning about Jane will more or less impact
beliefs about Alice
that individuals are more likely to endorse the valid modus ponens
(MP) inference than the equally valid modus tollens (MT)
inference. This pattern holds for both abstract task and probabilistic
task. The existing explanation for this phenomenon
within a Bayesian framework (e.g., Oaksford & Chater, 2008)
accounts for this asymmetry by assuming separate probability
distributions for both MP and MT. We propose a novel
explanation within a computational-level Bayesian account of
reasoning according to which “argumentation is learning”.
We show that the asymmetry must appear for certain prior
probability distributions, under the assumption that the conditional
inference provides the agent with new information that
is integrated into the existing knowledge by minimizing the
Kullback-Leibler divergence between the posterior and prior
probability distribution. We also show under which conditions
we would expect the opposite pattern, an MT-MP asymmetry.
with particular reference to Hawking radiation. First, we prove that such experiments can be confirmatory in Bayesian terms based upon appeal to ‘universality
arguments’. Second, we provide a formal model for the scaling behaviour of the
confirmation measure for multiple distinct realisations of the analogue system
and isolate a generic saturation feature. Finally, we demonstrate that different potential analogue realisations could provide different levels of confirmation. Our
results provide a basis both to formalise the epistemic value of analogue experiments that have been conducted and to advise scientists as to the respective
epistemic value of future analogue experiments.
methods by accuracy of finding the true (correct) ranked list.
Our research reveals that under most common circumstances
simple methods such as the average or majority actually tend
to outperform computationally-intensive distance-based methods. We then conduct a study to compare how actual people aggregate ranks in a group setting. Our finding is that individuals tend to adopt the group mean in a third of all revisions, making it the most popular strategy for belief revision.
- Provides clear, comprehensive, and accessible explanations
- Discusses a wide range of questions, from philosophical foundations to practical applications in science
- Combines mathematical modeling with conceptual analysis, simulations, case studies, and empirical results
Thinking through these challenges needs a new kind of interdisciplinary research community. Many sources of expertise and insight are likely to be relevant, and this community needs to be very well-connected in several dimensions – ‘horizontally’ between academic disciplines, ‘vertically’ to the policy and technology worlds, and of course geographically. AI is a global technology, and many of the challenges and opportunities of AI will be global in nature. Accordingly, getting AI right is not just an engineering challenge, but also a challenge for many other societal and academic sectors, including the humanities. Put another way, there is an engineering challenge of a ‘sociological’ kind, about how best to foster the necessary research community.
The field of decision theory is ideally placed to make contributions here, at several levels. AI innovations, including techniques from machine learning, are increasingly used to make decisions with significant social and ethical consequences, ranging from determining the news feeds on social media to making sentencing and parole recommendations in the criminal justice system. Decision theory provides and studies the standards by which such decisions are evaluated and improved. What is a rational decision? How can we train machines to make rational decisions? What is the relationship between human decision-making and machine decision-making? How can one make machine decision-making transparent (i.e. understandable to a human agent)? Which role does cognitive science play in these developments?
Perhaps even more importantly, the field of decision theory itself is highly interdisciplinary, with a strong presence in disciplines such as philosophy, mathematical logic, economics, psychology, and cognitive science, amongst others. In addition, of course, it has foundational links to computer science and machine learning. So it is ideally placed to contribute to the sociological challenge. It offers very fertile ground in which to foster the kind of rich interdisciplinary community needed for the challenges of AI, short term and long term.
This special issue stems from a conference series established with these goals in mind. Decision Theory and the Future of AI began in 2017 as a collaboration between the Leverhulme Centre for the Future of Intelligence (CFI) and the Centre for the Study of Existential Risk (CSER) at Cambridge, and Munich Center for Mathematical Philosophy (MCMP) at LMU Munich. The first two conferences were held at Trinity College, Cambridge in 2017 and LMU Munich in 2018. The first meeting outside Europe was held at ANU, Canberra, in 2019, in conjunction with ANU’s Humanising Machine Intelligence project. A fourth conference was planned at PKU, Beijing, in 2020, before Covid intervened. We will be back!
Several of the papers in this special issue were presented at one of these conferences, while others were submitted in response to an open call for papers. The range of topics, and even more so the range of authors and their home disciplines and affiliations, is a tribute to the richness of the territor