Papers by antoine lesieur
HAL (Le Centre pour la Communication Scientifique Directe), Apr 11, 2022
HAL (Le Centre pour la Communication Scientifique Directe), Oct 25, 2021
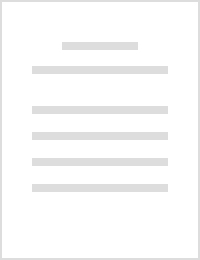
Sorbonne Université, Sep 9, 2021
La pollution sonore est un problème de santé publique bien identifié par les autorités sanitaires... more La pollution sonore est un problème de santé publique bien identifié par les autorités sanitaires. Afin d'établir l'exposition au bruit des populations, des cartes de bruit sont régulièrement générées. Ces cartes sont produites pour les principales sources de bruit. Pour le trafic routier, elles sont le résultat de simulations qui estiment le niveau de bruit à partir des données de trafic, des données météorologiques, de la topographie, de la répartition des bâtiments et de la végétation. Les cartes qui en résultent sont une estimation de la distribution spatiale des niveaux de bruit moyens sur la zone d'étude. Ces données sont limitées dans l'espace, il existe certaines incertitudes qui empêchent de connaître précisément le trafic moyen annuel sur toutes les routes de la zone d'étude. La précision des cartes de bruit est limitée par la longueur du temps de calcul, qui nécessite des modèles simplifiés de la propagation acoustique. En complément des cartes de bruit, les acteurs concernés réalisent des campagnes de mesure des niveaux de bruit. Elles mesurent l'évolution temporelle des niveaux de bruit à une série d'emplacements donnés. Ces données donnent une image plus réaliste du niveau de bruit réel que les résultats des simulations des cartes de bruit, mais elles sont très locales. Elles sont également coûteuses, ce qui interdit le maillage extensif d'une zone avec un réseau de capteurs. La combinaison des approches de modélisation et de mesure permettrait d'augmenter la quantité de données utiles à la production de cartes de bruit. Une carte de bruit qui combine les deux approches peut surmonter les limites de la simulation et de la mesure et fournir une cartographie dynamique et en temps réel des niveaux de bruit. L'objectif de cette thèse est de mettre en œuvre des méthodes dites d'assimilation de données pour réunir les avantages des deux approches, simulation et observation.Noise pollution is a public health problem well identified by health authorities. In order to establish the noise exposure of populations, noise maps are regularly generated. These maps are produced for the main sources of noise. For road traffic, they are the result of simulations that estimate the noise level from traffic data, meteorological data, topography, building distribution and vegetation. The resulting maps are an estimate of the spatial distribution of average noise levels over the study area. These data are spatially limited, there exists some uncertainties which prevent to know precisely the annual average traffic on all the roads in the study area. The accuracy of the noise maps is limited by the length of the computation time, which requires fairly simple models of the acoustic propagation. In addition to noise maps, stakeholders carry out noise level measurement campaigns. They measure the temporal evolution of noise levels at a series of given locations. These data give a more realistic reflection of the actual noise level than the results of noise map simulations, but they are very local. They are also expensive, which prohibits an extensive gridding of an area with a network of sensors. Combining modeling and measurement approaches would increase the amount of data useful for the production of noise maps. A noise map that combines the two approaches can overcome the limitations of simulation and measurement and provide dynamic, real-time mapping of noise levels. The objective of this thesis is to implement so-called data assimilation methods to unite the benefits of both approaches, simulation and observation

Proceedings of the ICA congress, 2019
Urban noise maps are usually computed by noise software which couples emission and attenuation ru... more Urban noise maps are usually computed by noise software which couples emission and attenuation rules like CNOSSOS. These models can require several hours to produce a map over a city for a single set of input data. This computational cost makes the models unusable for applications like uncertainty quantification where hundreds of simulations may be required. One solution is to replace the physical model with a meta-model which is extremely fast and yet fairly reproduces the results of the physical model. The strategy is first to reduce the dimension of both inputs and outputs of the physical model, which leads to a reduced model. This reduced model is then replaced by a statistical emulator. The emulator is trained with calls to the reduced model for a set of chosen inputs. The emulator relies on interpolation between the training output values. We applied this approach to the NoiseModelling software over the city of Lorient (France), using Kriging at the emulation step. It required a training set of 2000 calls to the physical model, but then the meta-model is 25 000 times faster than the physical model, while preserving the main behavior of the physical model, with 1.58 dB of mean quadratic error.
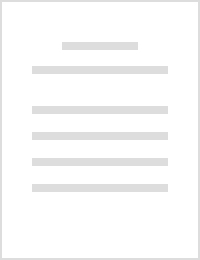
Applied Acoustics, 2021
Abstract Accurately predicting dynamic noise levels in urban environments is non-trivial. This st... more Abstract Accurately predicting dynamic noise levels in urban environments is non-trivial. This study aims to optimally combine both simulated and empirical data. Acoustic data from microphone arrays, traffic and weather data was merged with a simulated noise map, created with a statistical emulator tool (meta-model). Each hour, a noise map is generated by the meta-model with the measured traffic and weather data. This map is algorithmically merged with the measured readings to form a new composite map. The resulting analyzed map is the best linear unbiased estimator under certain assumptions. The performance is evaluated with leave-one-out cross-validation. The performance of the method depends on the accuracy of the meta-model, the input parameters of the meta-model and the structure of the error covariances between the simulated noise level errors. With 16 microphones over an area of 3 km2, this new method achieves a reduction of 30% of the root-mean-square error when compared to a meta-model only.
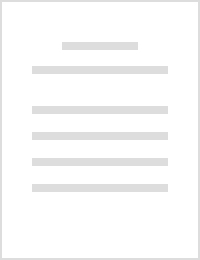
The Journal of the Acoustical Society of America, 2021
This study aims to produce dynamic noise maps based on a noise model and acoustic measurements. T... more This study aims to produce dynamic noise maps based on a noise model and acoustic measurements. To do so, inverse modeling and joint state-parameter methods are proposed. These methods estimate the input parameters that optimize a given cost function calculated with the resulting noise map and the noise observations. The accuracy of these two methods is compared with a noise map generated with a meta-model and with a classical data assimilation method called best linear unbiased estimator. The accuracy of the data assimilation processes is evaluated using a "leave-one-out" cross-validation method. The most accurate noise map is generated computing a joint state-parameter estimation algorithm without a priori knowledge about traffic and weather and shows a reduction of approximately 26% in the root mean square error from 3.5 to 2.6 dB compared to the reference meta-model noise map with 16 microphones over an area of 3 km2.

The Journal of the Acoustical Society of America, 2020
Urban noise maps are usually computed by noise software which couples emission and attenuation ru... more Urban noise maps are usually computed by noise software which couples emission and attenuation rules like CNOSSOS. These models can require several hours to produce a map over a city for a single set of input data. This computational cost makes the models unusable for applications like uncertainty quantification where hundreds of simulations may be required. One solution is to replace the physical model with a meta-model which is extremely fast and yet fairly reproduces the results of the physical model. The strategy is first to reduce the dimension of both inputs and outputs of the physical model, which leads to a reduced model. This reduced model is then replaced by a statistical emulator. The emulator is trained with calls to the reduced model for a set of chosen inputs. The emulator relies on interpolation between the training output values. We applied this approach to the NoiseModelling software over the city of Lorient (France), using Kriging at the emulation step. It required a training set of 2000 calls to the physical model, but then the meta-model is 25 000 times faster than the physical model, while preserving the main behavior of the physical model, with 1.58 dB of mean quadratic error.
Uploads
Papers by antoine lesieur