Papers by Soukaina Ouhame
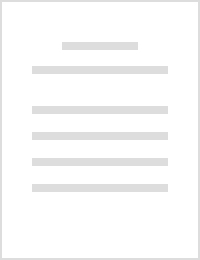
Neural Computing and Applications, Mar 10, 2021
Cloud computing provides different kind of services for users and provides with the help of inter... more Cloud computing provides different kind of services for users and provides with the help of internet. The Infrastructure as a service is a service model that provides virtual computing resources such as, networking, hardware, and storage services as needed for users. However, cloud-hosting initialization takes several minutes delay in the hardware resource allocation process. To resolve this issue, we need to predict the future amount of computing. In this paper, we propose a convolutional neural network and long short-term memory model for predicting multivariate workload which are the central processing unit, memory, and network usage. Firstly, the input data are analyzed by the vector auto regression method which filters the linear interdependencies among the multivariate data. Then, the residual data are computed and entered into the convolutional neural network layer which extracts complex features of each of the virtual machine usage components after the long short-term memory neural network, which is suitable for modeling temporal information of irregular trends in time series components in the proposed hybrid model latest activation function scaled polynomial constant unit used. The proposed model is compared with other predictive models. Based on the result, the proposed model shows the accuracy rate enhanced by approximately 3.8% to 10.9% and the error percentage rate also reduces by approximately 7% to 8.5% as compared to the other different models. It means the proposed technique improves central processing unit, memory, disk, and network usage in the network taking less amount of time due to the good predication approach compare to other models. In future research, we implement the proposed technique for VM energy section as well data predication system in cloud data center.
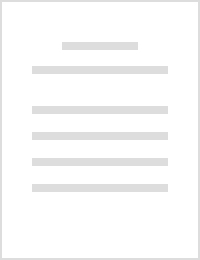
Infrastructure as a service (IaaS) is a form of cloud computing services that provides virtual co... more Infrastructure as a service (IaaS) is a form of cloud computing services that provides virtual computing resources in the form of hardware, networking, and storage services to the end users as needed in an elastic manner. However, cloud-hosting platforms introduce several minutes delay in the hardware resource allocation. The obvious solution to this issue is to predict the future need of computing resources and allocate them before being requested. This paper represents a hybrid method for predicting multivariate workload based on the Vector Autoregressive (VAR) model and the Stacked Long Short Term Memory (LSTM) model. In the proposed method, two metrics are used: CPU and memory usage, the VAR model is used to filter the linear interdependencies among the multivariate time series, and the stacked LSTM model to capture nonlinear trends in the residuals computed from the VAR model. The proposed hybrid model is compared with other hybrid predictive models: the ARMLP model, the RNN-GRU model and the ARIMA-LSTM model. Results of experiments show superior efficacy of the proposed method over the other hybrid models.
Indonesian Journal of Electrical Engineering and Computer Science, Dec 1, 2020
International journal of online and biomedical engineering, Nov 30, 2020
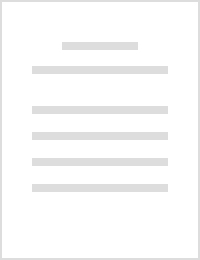
Proceedings of the New Challenges in Data Sciences: Acts of the Second Conference of the Moroccan Classification Society
Infrastructure as a service (IaaS) is a form of cloud computing services that provides virtual co... more Infrastructure as a service (IaaS) is a form of cloud computing services that provides virtual computing resources in the form of hardware, networking, and storage services to the end users as needed in an elastic manner. However, cloud-hosting platforms introduce several minutes delay in the hardware resource allocation. The obvious solution to this issue is to predict the future need of computing resources and allocate them before being requested. This paper represents a hybrid method for predicting multivariate workload based on the Vector Autoregressive (VAR) model and the Stacked Long Short Term Memory (LSTM) model. In the proposed method, two metrics are used: CPU and memory usage, the VAR model is used to filter the linear interdependencies among the multivariate time series, and the stacked LSTM model to capture nonlinear trends in the residuals computed from the VAR model. The proposed hybrid model is compared with other hybrid predictive models: the ARMLP model, the RNN-GRU model and the ARIMA-LSTM model. Results of experiments show superior efficacy of the proposed method over the other hybrid models.
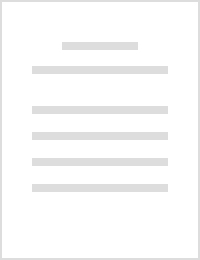
Neural Computing and Applications, 2021
Cloud computing provides different kind of services for users and provides with the help of inter... more Cloud computing provides different kind of services for users and provides with the help of internet. The Infrastructure as a service is a service model that provides virtual computing resources such as, networking, hardware, and storage services as needed for users. However, cloud-hosting initialization takes several minutes delay in the hardware resource allocation process. To resolve this issue, we need to predict the future amount of computing. In this paper, we propose a convolutional neural network and long short-term memory model for predicting multivariate workload which are the central processing unit, memory, and network usage. Firstly, the input data are analyzed by the vector auto regression method which filters the linear interdependencies among the multivariate data. Then, the residual data are computed and entered into the convolutional neural network layer which extracts complex features of each of the virtual machine usage components after the long short-term memory neural network, which is suitable for modeling temporal information of irregular trends in time series components in the proposed hybrid model latest activation function scaled polynomial constant unit used. The proposed model is compared with other predictive models. Based on the result, the proposed model shows the accuracy rate enhanced by approximately 3.8% to 10.9% and the error percentage rate also reduces by approximately 7% to 8.5% as compared to the other different models. It means the proposed technique improves central processing unit, memory, disk, and network usage in the network taking less amount of time due to the good predication approach compare to other models. In future research, we implement the proposed technique for VM energy section as well data predication system in cloud data center.
Artificial Intelligence Review, 2021

Indonesian Journal of Electrical Engineering and Computer Science, 2020
Cloud computing is an advanced technology which provides services with the help of internet. Thes... more Cloud computing is an advanced technology which provides services with the help of internet. These services work under the rule of pay and gain. The services consist of hardware and software used in different fields of life. Due to growth of cloud computing the number of users are increased and their demand for better services also increased with the passage of time. Cloud computing faces different issues. One of them is resource scheduling. In this paper a new technique is used for improvement of scheduling in cloud computing. The improvement took place in GWO algorithm. Two main sections of this algorithm are modified, which are local search section and fitness function value. The above proposed technique is used to improve three main parameters of scheduling that are energy consumption, throughput and average network executation time in VM for cloud computing. The techniques results are compared with ABC algorithm and GWO algorithm. The results show that proposed algorithm impr...
Journal of Computational Science, 2018

International Journal of Advances in Applied Sciences (IJAAS), 2024
The widespread demand for hosting application services in the cloud has been fueled by the deploy... more The widespread demand for hosting application services in the cloud has been fueled by the deployment of cloud data centers (CDCs) on a global scale. Furthermore, modern apps' resource needs have sharply increased, especially in industries that use a lot of data. As a result, more cloud servers have been made available, resulting in higher energy usage and, ecological problems. Large-scale data centers have been developed as a result of the rapidly increasing demand for cloud services, allowing application service providers to rent data center space for application deployment by user-required quality of service (QoS). These data centers use a lot of electricity, which raises running expenses and produces more carbon dioxide (CO2) emissions. Modern cloud computing environments must also provide QoS for their users, necessitating a trade-off between power performance, energy consumption, and service-level agreement (SLA) compliance. We present an intelligent resource management policy using enforcement learning for CDCs. The objective is to continuously consolidate and dynamically allocate virtual machines (VMs). Utilizing live migration and disabling inactive nodes to reduce power consumption in this cloud environment while maintaining service quality. To enable dynamic resource management, a better power-performance tradeoff, and significantly lower energy consumption, we integrate several artificial intelligence concepts. Based on the result the proposed approach is more efficient as compared with other techniques.
Uploads
Papers by Soukaina Ouhame