Papers by Dominik Michels
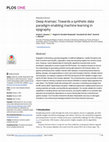
PLoS ONE, 2024
Epigraphy increasingly turns to modern artificial intelligence (AI) technologies such as machine ... more Epigraphy increasingly turns to modern artificial intelligence (AI) technologies such as machine learning (ML) for extracting insights from ancient inscriptions. However, scarce labeled data for training ML algorithms severely limits current techniques, especially for ancient scripts like Old Aramaic. Our research pioneers an innovative methodology for generating synthetic training data tailored to Old Aramaic letters. Our pipeline synthesizes photo-realistic Aramaic letter datasets, incorporating textural features, lighting, damage, and augmentations to mimic real-world inscription diversity. Despite minimal real examples, we engineer a dataset of 250,000 training and 25,000 validation images covering the 22 letter classes in the Aramaic alphabet. This comprehensive corpus provides a robust volume of data for training a residual neural network (ResNet) to classify highly
degraded Aramaic letters. The ResNet model demonstrates 95 accuracy in classifying real images from the 8th century BCE Hadad statue inscription (KAI 214). Additional experiments validate performance on varying materials and styles, proving effective generalization. Our results validate the model’s capabilities in handling diverse real-world scenarios, proving the viability of our synthetic data approach and avoiding the dependence on scarce training data that has constrained epigraphic analysis. Our innovative framework elevates interpretation accuracy on damaged inscriptions, thus enhancing knowledge extraction from these historical resources.
Uploads
Papers by Dominik Michels
degraded Aramaic letters. The ResNet model demonstrates 95 accuracy in classifying real images from the 8th century BCE Hadad statue inscription (KAI 214). Additional experiments validate performance on varying materials and styles, proving effective generalization. Our results validate the model’s capabilities in handling diverse real-world scenarios, proving the viability of our synthetic data approach and avoiding the dependence on scarce training data that has constrained epigraphic analysis. Our innovative framework elevates interpretation accuracy on damaged inscriptions, thus enhancing knowledge extraction from these historical resources.
degraded Aramaic letters. The ResNet model demonstrates 95 accuracy in classifying real images from the 8th century BCE Hadad statue inscription (KAI 214). Additional experiments validate performance on varying materials and styles, proving effective generalization. Our results validate the model’s capabilities in handling diverse real-world scenarios, proving the viability of our synthetic data approach and avoiding the dependence on scarce training data that has constrained epigraphic analysis. Our innovative framework elevates interpretation accuracy on damaged inscriptions, thus enhancing knowledge extraction from these historical resources.