Papers by Carlotta Domeniconi

Multi-view clustering aims at exploiting information from multiple heterogeneous views to promote... more Multi-view clustering aims at exploiting information from multiple heterogeneous views to promote clustering. Most previous works search for only one optimal clustering based on the predefined clustering criterion, but devising such a criterion that captures what users need is difficult. Due to the multiplicity of multi-view data, we can have meaningful alternative clusterings. In addition, the incomplete multi-view data problem is ubiquitous in real world but has not been studied for multiple clusterings. To address these issues, we introduce a deep incomplete multi-view multiple clusterings (DiMVMC) framework, which achieves the completion of data view and multiple shared representations simultaneously by optimizing multiple groups of decoder deep networks. In addition, it minimizes a redundancy term to simultaneously control the diversity among these representations and among parameters of different networks. Next, it generates an individual clustering from each of these shared representations. Experiments on benchmark datasets confirm that DiMVMC outperforms the state-of-the-art competitors in generating multiple clusterings with high diversity and quality.

arXiv (Cornell University), Dec 24, 2019
Crowdsourcing is a relatively economic and efficient solution to collect annotations from the cro... more Crowdsourcing is a relatively economic and efficient solution to collect annotations from the crowd through online platforms. Answers collected from workers with different expertise may be noisy and unreliable, and the quality of annotated data needs to be further maintained. Various solutions have been attempted to obtain high-quality annotations. However, they all assume that workers' label quality is stable over time (always at the same level whenever they conduct the tasks). In practice, workers' attention level changes over time, and the ignorance of which can affect the reliability of the annotations. In this paper, we focus on a novel and realistic crowdsourcing scenario involving attention-aware annotations. We propose a new probabilistic model that takes into account workers' attention to estimate the label quality. Expectation propagation is adopted for efficient Bayesian inference of our model, and a generalized Expectation Maximization algorithm is derived to estimate both the ground truth of all tasks and the label-quality of each individual crowd worker with attention. In addition, the number of tasks best suited for a worker is estimated according to changes in attention. Experiments against related methods on three realworld and one semi-simulated datasets demonstrate that our method quantifies the relationship between workers' attention and label-quality on the given tasks, and improves the aggregated labels.

Proceedings of the ... AAAI Conference on Artificial Intelligence, Apr 3, 2020
Multi-view clustering aims at integrating complementary information from multiple heterogeneous v... more Multi-view clustering aims at integrating complementary information from multiple heterogeneous views to improve clustering results. Existing multi-view clustering solutions can only output a single clustering of the data. Due to their multiplicity, multi-view data, can have different groupings that are reasonable and interesting from different perspectives. However, how to find multiple, meaningful, and diverse clustering results from multi-view data is still a rarely studied and challenging topic in multi-view clustering and multiple clusterings. In this paper, we introduce a deep matrix factorization based solution (DMClusts) to discover multiple clusterings. DMClusts gradually factorizes multi-view data matrices into representational subspaces layer-by-layer and generates one clustering in each layer. To enforce the diversity between generated clusterings, it minimizes a new redundancy quantification term derived from the proximity between samples in these subspaces. We further introduce an iterative optimization procedure to simultaneously seek multiple clusterings with quality and diversity. Experimental results on benchmark datasets confirm that DMClusts outperforms state-of-the-art multiple clustering solutions.

Traditional approaches to document classification requires labeled data in order to construct rel... more Traditional approaches to document classification requires labeled data in order to construct reliable and accurate classifiers. Unfortunately, labeled data are seldom available, and often too expensive to obtain, especially for large domains and fast evolving scenarios. Given a learning task for which training data are not available, abundant labeled data may exist for a different but related domain. One would like to use the related labeled data as auxiliary information to accomplish the classification task in the target domain. Recently, the paradigm of transfer learning has been introduced to enable effective learning strategies when auxiliary data obey a different probability distribution. A co-clustering based classification algorithm has been previously proposed to tackle cross-domain text classification. In this work, we extend the idea underlying this approach by making the latent semantic relationship between the two domains explicit. This goal is achieved with the use of Wikipedia. As a result, the pathway that allows to propagate labels between the two domains not only captures common words, but also semantic concepts based on the content of documents. We empirically demonstrate the efficacy of our semantic-based approach to cross-domain classification using a variety of real data.

arXiv (Cornell University), May 29, 2019
Hashing has been widely adopted for large-scale data retrieval in many domains, due to its low st... more Hashing has been widely adopted for large-scale data retrieval in many domains, due to its low storage cost and high retrieval speed. Existing cross-modal hashing methods optimistically assume that the correspondence between training samples across modalities are readily available. This assumption is unrealistic in practical applications. In addition, these methods generally require the same number of samples across different modalities, which restricts their flexibility. We propose a flexible cross-modal hashing approach (FlexCMH) to learn effective hashing codes from weakly-paired data, whose correspondence across modalities are partially (or even totally) unknown. FlexCMH first introduces a clustering-based matching strategy to explore the local structure of each cluster, and thus to find the potential correspondence between clusters (and samples therein) across modalities. To reduce the impact of an incomplete correspondence, it jointly optimizes in a unified objective function the potential correspondence, the cross-modal hashing functions derived from the correspondence, and a hashing quantitative loss. An alternative optimization technique is also proposed to coordinate the correspondence and hash functions, and to reinforce the reciprocal effects of the two objectives. Experiments on publicly multi-modal datasets show that FlexCMH achieves significantly better results than state-of-the-art methods, and it indeed offers a high degree of flexibility for practical cross-modal hashing tasks.
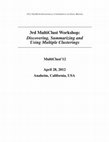
Cluster detection is a well-established data analysis task with several decades of research. Howe... more Cluster detection is a well-established data analysis task with several decades of research. However, it also includes a large variety of different subtopics investigated by different communities such as data mining, machine learning, statistics, and database systems. "Discovering, Summarizing and Using Multiple Clusterings" is one of these emerging research fields, which is developed in all of these communities. Unfortunately, it is difficult to identify related work on this topic as different research communities are using different vocabulary and are publishing at different venues. This hinders concise and focused research as the amount of literature published every year is scattered and readers might not get the overall perspective on this topic. The MultiClust workshop is therefore aiming at bringing together researchers that, somehow, are all tackling fundamentally identical-or rather similar-problems, around "Discovering, Summarizing and Using Multiple Clusterings". Yet they tackle these problems with different backgrounds, focus on different details, and include ideas from different research communities. This diversity is a major potential for this emerging field and should be highlighted by this workshop. In paper presentations and discussions, we would like to encourage the workshop participants to look at their own research problems from multiple perspectives. Bridging research areas in "Multiple Clusterings" can be observed as the general idea of the entire series of MultiClust Workshops, started at ACM SIGKDD 2010, continued at ECML PKDD 2011, up to the workshop at SDM 2012. Problems known in subspace clustering met similar problems in other areas like ensemble clustering, alternative clustering, or multi-view clustering. Related research fields such as pattern mining came into the focus. Cluster exploration and visualization is another very related field, which has been discussed in all MultiClust workshops. Keeping this tradition, the 3rd MultiClust workshop, at SIAM Data Mining 2012, again encounters insights from other related fields, such as constrained clustering, distance learning, and co-learning in multiple representations. Overall, the workshop's technical program again demonstrates the strong interest from different research communities. In particular, we have five peer-reviewed papers covering multiple research directions. They passed a competitive selection process ensuring high quality publications. Furthermore, we are pleased to have two excellent speakers giving invited talks that provide an overview on challenges in related fields: Carlotta Domeniconi (George Mason University, USA) and Shai Ben-David (University of Waterloo, Canada) contribute with their recent work in this area. And finally, in the spirit of previous workshops, the panel opens for a discussion of state-of-the-art, open challenges, and visions for future research. It wraps up the workshop by summarizing common challenges, establishing novel collaborations, and providing a guideline for topics to be addressed in following workshops. As organizers of this workshop, we are grateful for the support of the SIAM Data Mining conference, assisting us with all organization issues. Especially we would like to thank the workshop chairs, Rui Kuang and Chandan Reddy. Furthermore, we also gratefully acknowledge the MultiClust 2012 program committee for conducting thorough reviews of the submitted technical papers. We are pleased to have some of the core researchers of the covered research topics in the MultiClust committee.

arXiv (Cornell University), May 13, 2019
Multiple clustering aims at exploring alternative clusterings to organize the data into meaningfu... more Multiple clustering aims at exploring alternative clusterings to organize the data into meaningful groups from different perspectives. Existing multiple clustering algorithms are designed for singleview data. We assume that the individuality and commonality of multi-view data can be leveraged to generate high-quality and diverse clusterings. To this end, we propose a novel multi-view multiple clustering (MVMC) algorithm. MVMC first adapts multi-view self-representation learning to explore the individuality encoding matrices and the shared commonality matrix of multi-view data. It additionally reduces the redundancy (i.e., enhancing the individuality) among the matrices using the Hilbert-Schmidt Independence Criterion (HSIC), and collects shared information by forcing the shared matrix to be smooth across all views. It then uses matrix factorization on the individual matrices, along with the shared matrix, to generate diverse clusterings of high-quality. We further extend multiple co-clustering on multi-view data and propose a solution called multi-view multiple co-clustering (MVMCC). Our empirical study shows that MVMC (MVMCC) can exploit multiview data to generate multiple high-quality and diverse clusterings (co-clusterings), with superior performance to the state-of-the-art methods.

Proceedings of the ... AAAI Conference on Artificial Intelligence, Jul 17, 2019
Multiple clustering aims at discovering diverse ways of organizing data into clusters. Despite th... more Multiple clustering aims at discovering diverse ways of organizing data into clusters. Despite the progress made, it's still a challenge for users to analyze and understand the distinctive structure of each output clustering. To ease this process, we consider diverse clusterings embedded in different subspaces, and analyze the embedding subspaces to shed light into the structure of each clustering. To this end, we provide a two-stage approach called MISC (Multiple Independent Subspace Clusterings). In the first stage, MISC uses independent subspace analysis to seek multiple and statistical independent (i.e. non-redundant) subspaces, and determines the number of subspaces via the minimum description length principle. In the second stage, to account for the intrinsic geometric structure of samples embedded in each subspace, MISC performs graph regularized semi-nonnegative matrix factorization to explore clusters. It additionally integrates the kernel trick into matrix factorization to handle non-linearly separable clusters. Experimental results on synthetic datasets show that MISC can find different interesting clusterings from the sought independent subspaces, and it also outperforms other related and competitive approaches on real-world datasets.
Proceedings of the 2021 SIAM International Conference on Data Mining (SDM), 2021
Manifold regularization methods for matrix factorization rely on the cluster assumption, whereby ... more Manifold regularization methods for matrix factorization rely on the cluster assumption, whereby the neighborhood structure of data in the input space is preserved in the factorization space. We argue that using the k-neighborhoods of all data points as regularization constraints can negatively affect the quality of the factorization, and propose an unsupervised and selective regularized matrix factorization algorithm to tackle this problem. Our approach jointly learns a sparse set of representatives and their neighbor affinities, and the data factorization.We further propose a fast approximation of our approach by relaxing the selectivity constraints on the data. Our proposed algorithms are competitive against baselines and state-of-the-art manifold regularization and clustering algorithms.
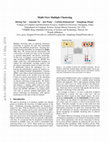
Proceedings of the Twenty-Eighth International Joint Conference on Artificial Intelligence, 2019
Multiple clustering aims at exploring alternative clusterings to organize the data into meaningfu... more Multiple clustering aims at exploring alternative clusterings to organize the data into meaningful groups from different perspectives. Existing multiple clustering algorithms are designed for single-view data. We assume that the individuality and commonality of multi-view data can be leveraged to generate high-quality and diverse clusterings. To this end, we propose a novel multi-view multiple clustering (MVMC) algorithm. MVMC first adapts multi-view self-representation learning to explore the individuality encoding matrices and the shared commonality matrix of multi-view data. It additionally reduces the redundancy (i.e., enhancing the individuality) among the matrices using the Hilbert-Schmidt Independence Criterion (HSIC), and collects shared information by forcing the shared matrix to be smooth across all views. It then uses matrix factorization on the individual matrices, along with the shared matrix, to generate diverse clusterings of high-quality. We further extend multiple c...
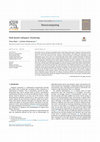
Neurocomputing, 2020
Data often exists in subspaces embedded within a high-dimensional space. Subspace clustering seek... more Data often exists in subspaces embedded within a high-dimensional space. Subspace clustering seeks to group data according to the dimensions relevant to each subspace. This requires the estimation of subspaces as well as the clustering of data. Subspace clustering becomes increasingly challenging in high dimensional spaces due to the curse of dimensionality which affects reliable estimations of distances and density. Recently, another aspect of high-dimensional spaces has been observed, known as the hubness phenomenon, whereby few data points appear frequently as nearest neighbors of the rest of the data. The distribution of neighbor occurrences becomes skewed with increasing intrinsic dimensionality of the data, and few points with high neighbor occurrences emerge as hubs. Hubs exhibit useful geometric properties and have been leveraged for clustering data in the full-dimensional space. In this paper, we study hubs in the context of subspace clustering. We present new characterizations of hubs in relation to subspaces, and design graph-based meta-features to identify a subset of hubs which are well fit to serve as seeds for the discovery of local latent subspaces and clusters. We propose and evaluate a hubnessdriven algorithm to find subspace clusters, and show that our approach is superior to the baselines, and is competitive against state-of-the-art subspace clustering methods. We also identify the data characteristics that make hubs suitable for subspace clustering. Such characterization gives valuable guidelines to data mining practitioners.

BMC systems biology, 2015
High throughput techniques produce multiple functional association networks. Integrating these ne... more High throughput techniques produce multiple functional association networks. Integrating these networks can enhance the accuracy of protein function prediction. Many algorithms have been introduced to generate a composite network, which is obtained as a weighted sum of individual networks. The weight assigned to an individual network reflects its benefit towards the protein functional annotation inference. A classifier is then trained on the composite network for predicting protein functions. However, since these techniques model the optimization of the composite network and the prediction tasks as separate objectives, the resulting composite network is not necessarily optimal for the follow-up protein function prediction. We address this issue by modeling the optimization of the composite network and the prediction problems within a unified objective function. In particular, we use a kernel target alignment technique and the loss function of a network based classifier to jointly ad...

Proceedings of the 14th ACM SIGKDD international conference on Knowledge discovery and data mining, 2008
Document classification presents difficult challenges due to the sparsity and the high dimensiona... more Document classification presents difficult challenges due to the sparsity and the high dimensionality of text data, and to the complex semantics of the natural language. The traditional document representation is a word-based vector (Bag of Words, or BOW), where each dimension is associated with a term of the dictionary containing all the words that appear in the corpus. Although simple and commonly used, this representation has several limitations. It is essential to embed semantic information and conceptual patterns in order to enhance the prediction capabilities of classification algorithms. In this paper, we overcome the shortages of the BOW approach by embedding background knowledge derived from Wikipedia into a semantic kernel, which is then used to enrich the representation of documents. Our empirical evaluation with real data sets demonstrates that our approach successfully achieves improved classification accuracy with respect to the BOW technique, and to other recently developed methods.

Proceedings of the 18th ACM SIGKDD international conference on Knowledge discovery and data mining, 2012
Advances in biotechnology have made available multitudes of heterogeneous proteomic and genomic d... more Advances in biotechnology have made available multitudes of heterogeneous proteomic and genomic data. Integrating these heterogeneous data sources, to automatically infer the function of proteins, is a fundamental challenge in computational biology. Several approaches represent each data source with a kernel (similarity) function. The resulting kernels are then integrated to determine a composite kernel, which is used for developing a function prediction model. Proteins are also found to have multiple roles and functions. As such, several approaches cast the protein function prediction problem within a multi-label learning framework. In our work we develop an approach that takes advantage of several unlabeled proteins, along with multiple data sources and multiple functions of proteins. We develop a graph-based transductive multi-label classifier (TMC) that is evaluated on a composite kernel, and also propose a method for data integration using the ensemble framework, called transductive multi-label ensemble classifier (TMEC). The TMEC approach trains a graph-based multi-label classifier for each individual kernel, and then combines the predictions of the individual models. Our contribution is the use of a bi-relational directed graph that captures relationships between pairs of proteins, between pairs of functions, and between proteins and functions. We evaluate the ability of TMC and TMEC to predict the functions of proteins by using two yeast datasets. We show that our approach performs better than recently proposed protein function prediction methods on composite and multiple kernels.

Lecture Notes in Computer Science, 2006
Semi-supervised clustering uses the limited background knowledge to aid unsupervised clustering a... more Semi-supervised clustering uses the limited background knowledge to aid unsupervised clustering algorithms. Recently, a kernel method for semi-supervised clustering has been introduced, which has been shown to outperform previous semi-supervised clustering approaches. However, the setting of the kernel's parameter is left to manual tuning, and the chosen value can largely affect the quality of the results. Thus, the selection of kernel's parameters remains a critical and open problem when only limited supervision, provided in terms of pairwise constraints, is available. In this paper, we derive a new optimization criterion to automatically determine the optimal parameter of an RBF kernel, directly from the data and the given constraints. Our approach integrates the constraints into the clustering objective function, and optimizes the parameter of a Gaussian kernel iteratively during the clustering process. Our experimental comparisons and results with simulated and real data clearly demonstrate the effectiveness and advantages of the proposed algorithm.

Lecture Notes in Computer Science, 2006
A critical problem in clustering research is the definition of a proper metric to measure distanc... more A critical problem in clustering research is the definition of a proper metric to measure distances between points. Semi-supervised clustering uses the information provided by the user, usually defined in terms of constraints, to guide the search of clusters. Learning effective metrics using constraints in high dimensional spaces remains an open challenge. This is because the number of parameters to be estimated is quadratic in the number of dimensions, and we seldom have enough sideinformation to achieve accurate estimates. In this paper, we address the high dimensionality problem by learning an ensemble of subspace metrics. This is achieved by projecting the data and the constraints in multiple subspaces, and by learning positive semi-definite similarity matrices therein. This methodology allows leveraging the given side-information while solving lower dimensional problems. We demonstrate experimentally using high dimensional data (e.g., microarray data) the superior accuracy achieved by our method with respect to competitive approaches.

2008 Eighth IEEE International Conference on Data Mining, 2008
Traditional approaches to document classification requires labeled data in order to construct rel... more Traditional approaches to document classification requires labeled data in order to construct reliable and accurate classifiers. Unfortunately, labeled data are seldom available, and often too expensive to obtain. Given a learning task for which training data are not available, abundant labeled data may exist for a different but related domain. One would like to use the related labeled data as auxiliary information to accomplish the classification task in the target domain. Recently, the paradigm of transfer learning has been introduced to enable effective learning strategies when auxiliary data obey a different probability distribution. A co-clustering based classification algorithm has been previously proposed to tackle cross-domain text classification. In this work, we extend the idea underlying this approach by making the latent semantic relationship between the two domains explicit. This goal is achieved with the use of Wikipedia. As a result, the pathway that allows to propagate labels between the two domains not only captures common words, but also semantic concepts based on the content of documents. We empirically demonstrate the efficacy of our semantic-based approach to cross-domain classification using a variety of real data.

2009 IEEE International Conference on Data Mining Workshops, 2009
Document classification is a key task for many text mining applications. However, traditional tex... more Document classification is a key task for many text mining applications. However, traditional text classification requires labeled data to construct reliable and accurate classifiers. Unfortunately, labeled data are seldom available. In this work, we propose a universal text classifier, which does not require any labeled document. Our approach simulates the capability of people to classify documents based on background knowledge. As such, we build a classifier that can effectively group documents based on their content, under the guidance of few words describing the classes of interest. Background knowledge is modeled using encyclopedic knowledge, namely Wikipedia. The universal text classifier can also be used to perform document retrieval. In our experiments with real data we test the feasibility of our approach for both the classification and retrieval tasks.

2008 Eighth IEEE International Conference on Data Mining, 2008
This paper presents Online Topic Model (OLDA), a topic model that automatically captures the them... more This paper presents Online Topic Model (OLDA), a topic model that automatically captures the thematic patterns and identifies emerging topics of text streams and their changes over time. Our approach allows the topic modeling framework, specifically the Latent Dirichlet Allocation (LDA) model, to work in an online fashion such that it incrementally builds an up-to-date model (mixture of topics per document and mixture of words per topic) when a new document (or a set of documents) appears. A solution based on the Empirical Bayes method is proposed. The idea is to incrementally update the current model according to the information inferred from the new stream of data with no need to access previous data. The dynamics of the proposed approach also provide an efficient mean to track the topics over time and detect the emerging topics in real time. Our method is evaluated both qualitatively and quantitatively using benchmark datasets. In our experiments, the OLDA has discovered interesting patterns by just analyzing a fraction of data at a time. Our tests also prove the ability of OLDA to align the topics across the epochs with which the evolution of the topics over time is captured. The OLDA is also comparable to, and sometimes better than, the original LDA in predicting the likelihood of unseen documents.
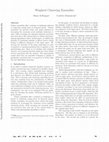
Proceedings of the 2006 SIAM International Conference on Data Mining, 2006
Cluster ensembles offer a solution to challenges inherent to clustering arising from its ill-pose... more Cluster ensembles offer a solution to challenges inherent to clustering arising from its ill-posed nature. Cluster ensembles can provide robust and stable solutions by leveraging the consensus across multiple clustering results, while averaging out emergent spurious structures that arise due to the various biases to which each participating algorithm is tuned. In this paper, we address the problem of combining multiple weighted clusters which belong to different subspaces of the input space. We leverage the diversity of the input clusterings in order to generate a consensus partition that is superior to the participating ones. Since we are dealing with weighted clusters, our consensus function makes use of the weight vectors associated with the clusters. The experimental results show that our ensemble technique is capable of producing a partition that is as good as or better than the best individual clustering.
Uploads
Papers by Carlotta Domeniconi