Papers by Bertrand Clarke
arXiv (Cornell University), Jan 23, 2018
arXiv (Cornell University), Aug 15, 2018
arXiv (Cornell University), Feb 16, 2016
International Statistical Review, 2011
Page 1. International Statistical Review (2011), 79, 1, 114143 doi:10.1111/j.1751-5823.2011.0013... more Page 1. International Statistical Review (2011), 79, 1, 114143 doi:10.1111/j.1751-5823.2011.00134.x Short Book Reviews Editor: Simo Puntanen Graphics for Statistics and Data Analysis with R Kevin J. Keen Chapman & Hall ...

arXiv (Cornell University), Sep 1, 2022
We give a decomposition of the posterior predictive variance using the law of total variance and ... more We give a decomposition of the posterior predictive variance using the law of total variance and conditioning on a finite dimensional discrete random variable. This random variable summarizes various features of modeling that are used to form the prediction for a future outcome. Then, we test which terms in this decomposition are small enough to ignore. This allows us identify which of the discrete random variables are most important to prediction intervals. The terms in the decomposition admit interpretations based on conditional means and variances and are analogous to the terms in a Cochran's theorem decomposition of squared error often used in analysis of variance. Thus, the modeling features are treated as factors in completely randomized design. In cases where there are multiple decompositions we suggest choosing the one that that gives the best predictive coverage with the smallest variance. Keywords-prediction intervals, posterior predictive variance, law of total variance, Bayes model averaging, stacking, ANOVA, bootstrap testing,Cochran's theorem.
Quality Engineering, 2005
ABSTRACT
Statistical theory and related fields, 2019

Journal of Applied Statistics, May 16, 2023
Background: Plant breeders want to develop cultivars that outperform existing genotypes. Some cha... more Background: Plant breeders want to develop cultivars that outperform existing genotypes. Some characteristics (here 'main traits') of these cultivars are categorical and difficult to measure directly. It is important to predict the main trait of newly developed genotypes accurately. In addition to marker data, breeding programs often have information on secondary traits (or 'phenotypes') that are easy to measure. Our goal is to improve prediction of main traits with interpretable relations by combining the two data types using variable selection techniques. However, the genomic characteristics can overwhelm the set of secondary traits, so a standard technique may fail to select any phenotypic variables. We develop a new statistical technique that ensures an appropriate representation of the phenotypic variables for prediction. Results: When two data types (markers and secondary traits) are available, we achieve improved prediction of a binary trait by two steps that are designed to ensure that a significant intrinsic effect of a phenotype is incorporated in the relation before accounting for the effects of genotypes. First, we sparsely regress the secondary traits on the markers and replace the secondary traits by their residuals to obtain the intrinsic effects of phenotypic variables with the influence of genotypes removed. Then, we develop a sparse logistic classifier using the markers and residuals so that the intrinsic phenotypes may be selected first to avoid being overwhelmed by the genotypes due to their numerical advantage. This classifier uses forward selection aided by a penalty term and can be computed effectively by a technique called the one-pass method. It compares favorably with other classifiers on simulated and real data.
Journal of Statistical Planning and Inference, 2004
Suppose X1; : : : ; Xn are IID p(•|Â;) where (Â;) ∈ R d is distributed according to the prior den... more Suppose X1; : : : ; Xn are IID p(•|Â;) where (Â;) ∈ R d is distributed according to the prior density w(•). For estimators Sn =S(X) and Tn =T (X) assumed to be consistent for some function of  and asymptotically normal, we examine the conditional Shannon mutual information (CSMI) between and Tn given and Sn, I (; Tn| ; Sn). It is seen there are several important special cases of this CSMI. We establish asymptotic formulas for various cases and identify the resulting noninformative reference priors. As a consequence, we develop the notion of data-dependent priors and a calibration for how close an estimator is to su ciency.
Canadian Journal of Statistics, 1999
The authors discuss a class of likelihood functions involving weak assumptions on data generating... more The authors discuss a class of likelihood functions involving weak assumptions on data generating mechanisms. These likelihoods may be appropriate when it is difficult to propose models for the data. The properties of these likelihoods are given and it is shown how they can be computed numerically by use of the Blahut‐Arimoto algorithm. The authors then show how these likelihoods can give useful inferences using a data set for which no plausible physical model is apparent. The plausibility of the inferences is enhanced by the extensive robustness analysis these likelihoods permit.
Statistical Theory and Related Fields, 2021
Many of the best predictors for complex problems are typically regarded as hard to interpret phys... more Many of the best predictors for complex problems are typically regarded as hard to interpret physically. These include kernel methods, Shtarkov solutions, and random forests. We show that, despite the inability to interpret these three predictors to infinite precision, they can be asymptotically approximated and admit conceptual interpretations in terms of their mathematical/statistical properties. The resulting expressions can be in terms of polynomials, basis elements, or other functions that an analyst may regard as interpretable.
R topics documented: easystab-package...................................... 2 from.hclust........... more R topics documented: easystab-package...................................... 2 from.hclust......................................... 2 from.kmeans........................................ 4 getOptTheta......................................... 6 make2dStabilityImage................................... 7 perturbationStability.................................... 9 plot.StabilityCollection................................... 11 1 2 from.hclust plot.StabilityReport..................................... 12 print.StabilityCollection................................... 14 print.StabilityReport.................................... 14 summary.StabilityCollection................................ 15 summary.StabilityReport.................................. 15 Index 16 easystab-package Clustering stability analysis using Bayesian perturbations. Description
Proceedings. IEEE International Symposium on Information Theory
Several diverse problems have solutions in terms of an information-theoretic quantity for which w... more Several diverse problems have solutions in terms of an information-theoretic quantity for which we examine the asymptotics. Let Y1, Yz, . , . , YN be a sample of random variables with distribution depending on a (possibly infinite-dimensional) parameter 8. The maximum of the mutual information IN = I(8; Y1, Yz, . . . , YN) over choices of the
Proceedings of 1994 Workshop on Information Theory and Statistics
We determine the asymptotic minimax redundancy of universal data compression in a parametric sett... more We determine the asymptotic minimax redundancy of universal data compression in a parametric setting and show that it corresponds to the use of Jeffreys prior. Statistically, this formulation of the coding problem can be interpreted in a prior selection context and in an estimation context
Thinking about Biology, 2018
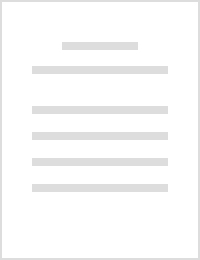
Water Research, 2021
While the microbiome of activated sludge (AS) in wastewater treatment plants (WWTPs) plays a vita... more While the microbiome of activated sludge (AS) in wastewater treatment plants (WWTPs) plays a vital role in shaping the resistome, identifying the potential bacterial hosts of antibiotic resistance genes (ARGs) in WWTPs remains challenging. The objective of this study is to explore the feasibility of using a machine learning approach, random forests (RF's), to identify the strength of associations between ARGs and bacterial taxa in metagenomic datasets from the activated sludge of WWTPs. Our results show that the abundance of select ARGs can be predicted by RF's using abundant genera (Candidatus Accumulibacter, Dechloromonas, Pesudomonas, and Thauera, etc.), (opportunistic) pathogens and indicators (Bacteroides, Clostridium, and Streptococcus, etc.), and nitrifiers (Nitrosomonas and Nitrospira, etc.) as explanatory variables. The correlations between predicted and observed abundance of ARGs (erm(B), tet(O), tet(Q), etc.) ranged from medium (0.400 < R2 < 0.600) to strong (R2 > 0.600) when validated on testing datasets. Compared to those belonging to the other two groups, individual genera in the group of (opportunistic) pathogens and indicator bacteria had more positive functional relationships with select ARGs, suggesting genera in this group (e.g., Bacteroides, Clostridium, and Streptococcus) may be hosts of select ARGs. Furthermore, RF's with (opportunistic) pathogens and indicators as explanatory variables were used to predict the abundance of select ARGs in a full-scale WWTP successfully. Machine learning approaches such as RF's can potentially identify bacterial hosts of ARGs and reveal possible functional relationships between the ARGs and microbial community in the AS of WWTPs.

Journal of Classification, 2018
Many of the best classifiers are ensemble methods such as bagging, random forests, boosting, and ... more Many of the best classifiers are ensemble methods such as bagging, random forests, boosting, and Bayes model averaging. We give conditions under which each of these four classifiers can be regarded as a Bayes classifier. We also give conditions under which stacking achieves the minimal Bayes risk. We compare the four classifiers with a logistic regression classifier to assess the cost of interpretability. First we characterize the increase in risk from using an ensemble method in a logistic classifier versus using it directly. Second, we characterize the change in risk from applying logistic regression to an ensemble method versus using the logistic classifier itself. Third, we give necessary and sufficient conditions for the logistic classifier to be worse than combining the logistic classifier and the Bayes classifier. Hence these results extend to ensemble classifiers that are asymptotically Bayes.

Statistical Analysis and Data Mining: The ASA Data Science Journal, 2015
We present a new technique for comparing models using a median form of cross-validation and least... more We present a new technique for comparing models using a median form of cross-validation and least median of squares estimation (MCV-LMS). Rather than minimizing the sums of squares of residual errors, we minimize the median of the squared residual errors. We compare this with a robustified form of cross-validation using the Huber loss function and robust coefficient estimators (HCV). Through extensive simulations we find that for linear models MCV-LMS outperforms HCV for data that is representative of the data generator when the tails of the noise distribution are heavy enough and asymmetric enough. We also find that MCV-LMS is often better able to detect the presence of small terms. Otherwise, HCV typically outperforms MCV-LMS for 'good' data. MCV-LMS also outperforms HCV in the presence of enough severe outliers. One of MCV and HCV also generally gives better model selection for linear models than the conventional version of crossvalidation with least squares estimators (CV-LS) when the tails of the noise distribution are heavy or asymmetric or when the coefficients are small and the data is representative. CV-LS only performs well when the tails of the error distribution are light and symmetric and the coefficients are large relative to the noise variance. Outside of these contexts and the contexts noted above, HCV outperforms CV-LS and MCV-LMS. We illustrate CV-LS, HVC, and MCV-LMS via numerous simulations to map out when each does best on representative data and then apply all three to a real dataset from econometrics that includes outliers.
Uploads
Papers by Bertrand Clarke