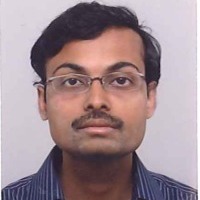
Krishna Prasad Miyapuram
Dr. Krishna Prasad Miyapuram has PhD in Cognitive Neuroscience from University of Cambridge, U.K. He has Post-doctoral research experience in both Industry and academia working at Unilever R&D in the Netherlands and at Center for Mind/Brain Sciences, University of Trento, Italy. He is currently working as Associate Professor in Cognitive Science and Computer Science at Indian Institute of Technology, IIT Gandhinagar (Gujarat, India) He has over 12 years of research experience in neuroimaging with functional MRI (predoctoral+doctoral). His Ph.D. was supported by prestigious Cambridge Nehru Fellowship, and UK research award for overseas students with excellent potential for research. Dr. Miyapuram has a multidisciplinary background with two masters degrees in Electronics and Artificial Intelligence. As a visiting researcher at ATR computational neuroscience laboratories, Kyoto, Japan, he was inspired into neuroscience research and the exciting world of studying human brain function in vivo non-invasively using fMRI. He has been then through a journey of extensive self study studying Neuroscience, Cognitive functions of learning and decision making and fMRI data analysis to an expert level in Statistical Parametric Mapping (SPM).
Research: Dr. Krishna's postdoctoral work was in ERC funded project was on predictive coding: coding the past to predict the future. His PhD thesis at Cambridge University was in Neuroeconomics studying the neural activations pertaining to stimuli predicting reward. His doctoral work extended the findings of sensory imagery into the reward domain and at the same time addressed two key methodological issues. The first was on the efficacy of visually presenting hypothetical monetary rewards to human participants in an fMRI scanner. The second was recovering signal dropouts from the orbitofrontal cortex due to air-tissue interface above the eye orbit. His postdoctoral research in Industry involved translation of fundamental research in behavioural economics into designing effective communication for commercial products.
Dr. Krishna's predoctoral research was on visuo-motor sequence learning. The main hypothesis was that during motor skill learning we transition from an early phase to an automatic phase, which are supported by distinct cortico-sub cortical loops. The publication in neuroimage journal was awarded editors' choice award for the year 2006.
Brief CV:
2011 - Postdoctoral Fellow, Center for Mind/Brain Sciences, University of Trento, Italy
2008 - 2011, Cognitive Psychologist/Neuroscientist, Unilever R&D, The Netherlands
2004 - 2008, Ph.D. Cognitive Neuroscience, fMRI of reward processing, University of Cambridge, UK
2002 - 2004, M.Tech. Artificial Intelligence, University of Hyderabad, India
2000 - 2002/4, Research Assistant, Indo-Japanese Project, fMRI of visuomotor sequence learning
2000 & 2003, Visiting Researcher, Advanced Telecommunications Research International, Kyoto, Japan
1998 - 2000, M.Sc. Electronics, University of Hyderabad, India
1999 - Summer Student, Physical Research Laboratory, Ahmedabad, India
Address: IIT Gandhingar
VGEC Campus, Chandkheda
Ahmedabad, India 382424
Research: Dr. Krishna's postdoctoral work was in ERC funded project was on predictive coding: coding the past to predict the future. His PhD thesis at Cambridge University was in Neuroeconomics studying the neural activations pertaining to stimuli predicting reward. His doctoral work extended the findings of sensory imagery into the reward domain and at the same time addressed two key methodological issues. The first was on the efficacy of visually presenting hypothetical monetary rewards to human participants in an fMRI scanner. The second was recovering signal dropouts from the orbitofrontal cortex due to air-tissue interface above the eye orbit. His postdoctoral research in Industry involved translation of fundamental research in behavioural economics into designing effective communication for commercial products.
Dr. Krishna's predoctoral research was on visuo-motor sequence learning. The main hypothesis was that during motor skill learning we transition from an early phase to an automatic phase, which are supported by distinct cortico-sub cortical loops. The publication in neuroimage journal was awarded editors' choice award for the year 2006.
Brief CV:
2011 - Postdoctoral Fellow, Center for Mind/Brain Sciences, University of Trento, Italy
2008 - 2011, Cognitive Psychologist/Neuroscientist, Unilever R&D, The Netherlands
2004 - 2008, Ph.D. Cognitive Neuroscience, fMRI of reward processing, University of Cambridge, UK
2002 - 2004, M.Tech. Artificial Intelligence, University of Hyderabad, India
2000 - 2002/4, Research Assistant, Indo-Japanese Project, fMRI of visuomotor sequence learning
2000 & 2003, Visiting Researcher, Advanced Telecommunications Research International, Kyoto, Japan
1998 - 2000, M.Sc. Electronics, University of Hyderabad, India
1999 - Summer Student, Physical Research Laboratory, Ahmedabad, India
Address: IIT Gandhingar
VGEC Campus, Chandkheda
Ahmedabad, India 382424
less
Related Authors
Howard Nusbaum
University of Chicago
Robert Lickliter
Florida International University
Steven Pinker
Harvard University
Michael Spivey
University of California, Merced
David Pierre Leibovitz
Carleton University
Don Ross
University College Cork
David Seamon
Kansas State University
Shaun Gallagher
University of Memphis
Armando Marques-Guedes
UNL - New University of Lisbon
Vittorio Gallese
Università degli Studi di Parma (Italy)
InterestsView All (33)
Uploads
Papers by Krishna Prasad Miyapuram
About the speaker:
Krishna Prasad (Miyapuram) has an interdisciplinary background with Masters in Electronics and Artificial Intelligence. His research area during last 10 years is primarily in the field of cognitive neuroscience. After PhD from Cambridge University, he has worked in consumer industry at Unilever in Netherlands. Beginning this year,he will be working as postdoctoral fellow at Univeristy of Trento in Italy.
The basic parameters of value-based decision making are defined from microeconomics as reward magnitude, probability, and delay. The behavioural economics approach has been investigated extensively in the financial domain. People make many decisions throughout the day not only involving finances, but also many other factors such as food and health.
Purpose:
Because money is easy to quantify and present visually, it is the reward of choice for most fMRI studies, even though it cannot be handed over to participants inside the scanner. Many of these decisions involve considering alternatives that will occur at some point in the future. The problem becomes much more challenging in case of environmental benefits.
Methods:
A typical fMRI study requires hundreds of trials and thus small amounts of monetary rewards per trial (e.g. 5p) if all trials are to be treated equally. However, small payoffs can have detrimental effects on performance due to their limited buying power. Hypothetical monetary rewards can overcome such limitations. However, people often fail to perform delay gratification. For example, consumption of low fat food and regular exercise is important for healthy living. This can be studied using the inter-temporal choice paradigm.
Result:
The efficacy of using hypothetical monetary rewards has been demonstrated from graded activation in midbrain observed in response to stimuli predicting increasing hypothetical rewards.
Conclusion:
An individual is unlikely to directly experience many environmental benefits in his/her life time. Hence there is need for transforming the basic research into practical situations.
Purpose: A typical brain imaging study has 10-20 participants whose data is extrapolated to the general population making the results obtained from the study questionable. To reach a significant conclusion it is necessary that the studies are replicated and variations across trials and within subjects are taken into consideration. Meta-analysis techniques pool a number of single studies on a particular psychological phenomenon and evaluate the consistency and specificity of the reported findings and hence forming a conclusive evidence of the mapping between the particular psychological process and brain region activation.
Methods: Here we critically review literature on the methods available for meta-analysis. These methods can be broadly classified into two: 1) The coordinate based meta-analysis (CBMA) which refers to the maximum likelihood of brain activation based on a universal three dimensional coordinate system and 2) The image based meta-analysis (IBMA) which considers the effect sizes from different studies to increase statistical power ignoring the inter-study consistency requirements.
Conclusion: Meta-analysis of single studies in neuroimaging helps exploring the data to find useful inferences like testing existing hypotheses, correspondence across domains, and developing new hypotheses which are otherwise not possible through single study analysis.
2x6 task. Behavioural analysis revealed improvements in performance measures of subjects such as speed and accuracy, pointing out successful learning of the sequence of finger movements accomplished by subjects. Traditional imaging analysis using the Statistical Parametric Mapping (SPM) approach revealed activation in several brain areas. However. SPM analysis averages out temporal changes and presents gross differences between the early and late stages of learning. As the main focus of the study is to understand the dynamic changes in brain activation induced by the learning process, the time course of activity of voxels in this area is modelled by statistical methods of time series analysis. Results from ARIMA model of activation values from the different brain regions revealed that the model parameters are distinctly different for the control/follow condition and the test/sequence condition. In the control condition subjects made finger movements in response to random visual cues and hence there was no learning. In contrast, in the sequence condition subjects progressively learned a fixed sequence of movements by responding to visual cues. A detailed analysis is required to tease out learning specific activity in the time series corresponding to the sequence epochs, for example to bring out differences in the early and late periods of the learning process. Although these results are still preliminary, they demonstrate that explicit modelling of fMRI time series is important in revealing and characterizing learning induced changes in the brain activity. Further, these methods seem more useful for investigating transient and long-term changes across the learning period than the traditional analysis methods.