Papers by Giorgio Licciardi
IEEE Journal of Selected Topics in Applied Earth Observations and Remote Sensing, 2012
This paper proposes a novel approach to the retrieval of buildings' heigh... more This paper proposes a novel approach to the retrieval of buildings' height from multi-angular high spatial resolution images. To achieve this task, we combined two main concepts: multilevel morphological attribute filters, used for the definition of the objects in the image, and geometric invariant moments exploited for the characterization of the spatial properties of the previously detected shapes. The main

EURASIP Journal on Advances in Signal Processing, 2012
This article presents a novel method for the enhancement of the spatial quality of hyperspectral ... more This article presents a novel method for the enhancement of the spatial quality of hyperspectral (HS) images through the use of a high resolution panchromatic (PAN) image. Due to the high number of bands, the application of a pan-sharpening technique to HS images may result in an increase of the computational load and complexity. Thus a dimensionality reduction preprocess, compressing the original number of measurements into a lower dimensional space, becomes mandatory. To solve this problem, we propose a pan-sharpening technique combining both dimensionality reduction and fusion, making use of non-linear principal component analysis (NLPCA) and Indusion, respectively, to enhance the spatial resolution of a HS image. We have tested the proposed algorithm on HS images obtained from CHRIS-Proba sensor and PAN image obtained from World view 2 and demonstrated that a reduction using NLPCA does not result in any significant degradation in the pan-sharpening results.
IEEE Transactions on Geoscience and Remote Sensing, 2015
In this paper state-of-the-art and advanced methods for multispectral pansharpening are reviewed ... more In this paper state-of-the-art and advanced methods for multispectral pansharpening are reviewed and evaluated on two very high resolution datasets acquired by IKONOS-2 (four bands) and WorldView-2 (eight bands). The experimental analysis allows us to highlight the performances of the two main pansharpening approaches (i.e. component substitution and multiresolution analysis).
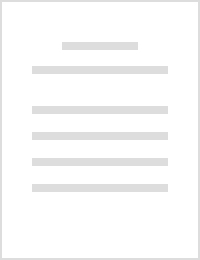
IEEE Transactions on Geoscience and Remote Sensing, 2015
ABSTRACT Many powerful pansharpening approaches exploit the functional relation between the fusio... more ABSTRACT Many powerful pansharpening approaches exploit the functional relation between the fusion of PANchromatic (PAN) and MultiSpectral (MS) images. To this purpose, the modulation transfer function of the MS sensor is typically used, being easily approximated as a Gaussian filter whose analytic expression is fully specified by the sensor gain at the Nyquist frequency. However, this characterization is often inadequate in practice. In this paper, we develop an algorithm for estimating the relation between PAN and MS images directly from the available data through an efficient optimization procedure. The effectiveness of the approach is validated both on a reduced scale data set generated by degrading images acquired by the IKONOS sensor and on full-scale data consisting of images collected by the QuickBird sensor. In the first case, the proposed method achieves performances very similar to that of the algorithm that relies upon the full knowledge of the degrading filter. In the second, it is shown to outperform several very credited state-of-the-art approaches for the extraction of the details used in the current literature.
IEEE Geoscience and Remote Sensing Letters, 2000
ABSTRACT The pansharpening process has the purpose of building a high-resolution multispectral im... more ABSTRACT The pansharpening process has the purpose of building a high-resolution multispectral image by fusing low spatial resolution multispectral and high-resolution panchromatic observations. A very credited method to pursue this goal relies upon the injection of details extracted from the panchromatic image into an upsampled version of the low-resolution multispectral image. In this letter, we compare two different injection methodologies and motivate the superiority of contrast-based methods both by physical consideration and by numerical tests carried out on remotely sensed data acquired by IKONOS and Quickbird sensors.
2014 IEEE Geoscience and Remote Sensing Symposium, 2014
2014 IEEE Geoscience and Remote Sensing Symposium, 2014
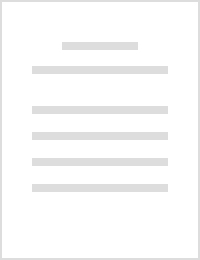
2014 IEEE International Conference on Image Processing (ICIP), 2014
Remote sensing hyperspectral images (HSI) are quite often low rank, in the sense that the data be... more Remote sensing hyperspectral images (HSI) are quite often low rank, in the sense that the data belong to a low dimensional subspace/manifold. This has been recently exploited for the fusion of low spatial resolution HSI with high spatial resolution multispectral images (MSI) in order to obtain super-resolution HSI. Most approaches adopt an unmixing or a matrix factorization perspective. The derived methods have led to state-of-the-art results when the spectral information lies in a low dimensional subspace/manifold. However, if the subspace/manifold dimensionality spanned by the complete data set is large, i.e., larger than the number of multispectral bands, the performance of these methods decrease mainly because the underlying sparse regression problem is severely ill-posed. In this paper, we propose a local approach to cope with this difficulty. Fundamentally, we exploit the fact that real world HSI are locally low rank, that is, pixels acquired from a given spatial neighborhood span a very low dimensional subspace/manifold, i.e., lower or equal than the number of multispectral bands. Thus, we propose to partition the image into patches and solve the data fusion problem independently for each patch. This way, in each patch the subspace/manifold dimensionality is low enough such that the problem is not ill-posed anymore. We propose two alternative approaches to define the hyperspectral super-resolution via local dictionary learning using endmember induction algorithms (HSRLDL- EIA). We also explore two alternatives to define the local regions, using sliding windows and binary partition trees. The effectiveness of the proposed approaches is illustrated with synthetic and semi real data.
Workshop on Hyperspectral Image and Signal Processing: Evolution in Remote Sensing, 2009
In this paper the potential of neural networks has been applied to hyperspectral data and exploit... more In this paper the potential of neural networks has been applied to hyperspectral data and exploited either for classification purposes or for data feature extraction and dimensionality reduction. For this latter task, a topology named autoassociative neural network has been used. In its complete form, the processing scheme uses a neural network architecture consisting of two stages: the first stage
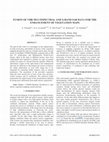
2012 IEEE International Geoscience and Remote Sensing Symposium, 2012
The goal of this work is to investigate on the enhancement, in terms of accuracy and number of cl... more The goal of this work is to investigate on the enhancement, in terms of accuracy and number of classes, of the vegetation mapping through the joint use of multi-sensors data. Several stacks of Spotlight COSMO-SkyMed, acquired both in HH and VV polarization, and Multispectral World-View2 images, taken in the same or different seasons, have been compounded and exploited to identify six types of natural surfaces by means of a Neural Network classifier. While the information content of the eight bands of the multispectral data may be sufficient to discriminate the classes of interest, the single polarization of each SAR image has to be integrated by extracting further features, such as textural parameters. The assessment of the provided vegetation maps has been carried out in terms of per class accuracy, overall accuracy and K coefficient. The achieved results demonstrate the improvement of the classifications obtained by fusing more information from multi-sensors acquisitions.
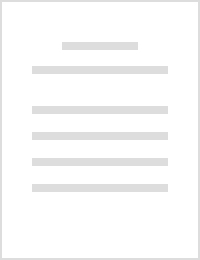
Remote sensing hyperspectral images (HSI) are quite often low rank, in the sense that the data be... more Remote sensing hyperspectral images (HSI) are quite often low rank, in the sense that the data belong to a low dimensional subspace/manifold. This has been recently exploited for the fusion of low spatial resolution HSI with high spatial resolution multispectral images (MSI) in order to obtain super-resolution HSI. Most approaches adopt an unmixing or a matrix factorization perspective. The derived methods have led to state-of-the-art results when the spectral information lies in a low dimensional subspace/manifold. However, if the subspace/manifold dimensionality spanned by the complete data set is large, i.e., larger than the number of multispectral bands, the performance of these methods decrease mainly because the underlying sparse regression problem is severely ill-posed. In this paper, we propose a local approach to cope with this difficulty. Fundamentally, we exploit the fact that real world HSI are locally low rank, that is, pixels acquired from a given spatial neighborhood span a very low dimensional subspace/manifold, i.e., lower or equal than the number of multispectral bands. Thus, we propose to partition the image into patches and solve the data fusion problem independently for each patch. This way, in each patch the subspace/manifold dimensionality is low enough such that the problem is not ill-posed anymore. We propose two alternative approaches to define the hyperspectral super-resolution via local dictionary learning using endmember induction algorithms (HSRLDL- EIA). We also explore two alternatives to define the local regions, using sliding windows and binary partition trees. The effectiveness of the proposed approaches is illustrated with synthetic and semi real data.
This work describes the estimation of the above ground live biomass (
Polarimetric SAR interferometric data can provide estimates of forest biomass density. There are ... more Polarimetric SAR interferometric data can provide estimates of forest biomass density. There are different approaches to deal with the inversion problem, such as neural networks and the traditional optimal estimation approach. This paper presents a study to evaluate their performance by means of quantitative indexes addressing both the computation time and the retrieval accuracy. Better forest parameters estimates have been
Since a few months TerraSAR-X has been acquiring X-band SAR images of the earth surface from spac... more Since a few months TerraSAR-X has been acquiring X-band SAR images of the earth surface from space. This contribution reports on a study carried out to understand the main textural features of the X-band radar return from various kinds of surfaces and in particular to assess the potential of images acquired by X-band space borne radars in mapping fire scars
In this paper we test an unsupervised neural network approach for extracting features from very h... more In this paper we test an unsupervised neural network approach for extracting features from very high resolution X-band SAR images. The purpose of this study is buildings recognition in images of low density urban areas, acquired by COSMO-Skymed and TerraSAR-X satellites, by means of Pulse Coupled Neural Network (PCNN), a relatively novel unsupervised algorithm based on models of the visual cortex of small mammals. The features retrieved from georeferenced SAR images are compared against the ground truth provided by corresponding optical images. The accuracy yielded by PCNN is quantitatively evaluated and critically discussed, also in comparison with commonly used feature extraction techniques.
Uploads
Papers by Giorgio Licciardi