Papers by Patrick Groenen
This paper puts forward kernel ridge regression as an approach for forecasting with many predicto... more This paper puts forward kernel ridge regression as an approach for forecasting with many predictors that are related nonlinearly to the target variable. In kernel ridge regression, the observed predictor variables are mapped nonlinearly into a high-dimensional space, where estimation of the predictive regression model is based on a shrinkage estimator to avoid overfitting. We extend the kernel ridge regression
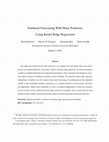
SSRN Electronic Journal, 2000
This paper puts forward kernel ridge regression as an approach for forecasting with many predicto... more This paper puts forward kernel ridge regression as an approach for forecasting with many predictors that are related nonlinearly to the target variable. In kernel ridge regression, the observed predictor variables are mapped nonlinearly into a high-dimensional space, where estimation of the predictive regression model is based on a shrinkage estimator to avoid overfitting. We extend the kernel ridge regression methodology to enable its use for economic time-series forecasting, by including lags of the dependent variable or other individual variables as predictors, as is typically desired in macroeconomic and financial applications. Monte Carlo simulations as well as an empirical application to various key measures of real economic activity confirm that kernel ridge regression can produce more accurate forecasts than traditional linear methods for dealing with many predictors based on principal component regression.
Consider the classification task of assigning a test object toone of two or more possible groups,... more Consider the classification task of assigning a test object toone of two or more possible groups, or classes. An intuitive way to proceedis to assign the object to that class, to which the distance is minimal. Asa distance measure to a class, we propose here to use the distance to theconvex hull of that class. Hence the name Nearest Convex
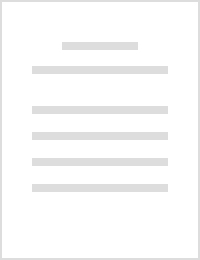
BioMed Research International, 2015
In recent years, there has been a considerable amount of research on the use of regularization me... more In recent years, there has been a considerable amount of research on the use of regularization methods for inference and prediction in quantitative genetics. Such research mostly focuses on selection of markers and shrinkage of their effects. In this review paper, the use of ridge regression for prediction in quantitative genetics using single-nucleotide polymorphism data is discussed. In particular, we consider (i) the theoretical foundations of ridge regression, (ii) its link to commonly used methods in animal breeding, (iii) the computational feasibility, and (iv) the scope for constructing prediction models with nonlinear effects (e.g., dominance and epistasis). Based on a simulation study we gauge the current and future potential of ridge regression for prediction of human traits using genome-wide SNP data. We conclude that, for outcomes with a relatively simple genetic architecture, given current sample sizes in most cohorts (i.e., N < 10,000) the predictive accuracy of ridge regression is slightly higher than the classical genome-wide association study approach of repeated simple regression (i.e., one regression per SNP). However, both capture only a small proportion of the heritability. Nevertheless, we find evidence that for large-scale initiatives, such as biobanks, sample sizes can be achieved where ridge regression compared to the classical approach improves predictive accuracy substantially.
Reliable Knowledge Discovery, 2012
Chapman & Hall/CRC Statistics in the Social and Behavioral Sciences, 2006
In analysis of variance, there is usually little attention for interpreting the terms of the effe... more In analysis of variance, there is usually little attention for interpreting the terms of the effects themselves, especially for interaction effects. One of the reasons is that the number of interaction-effect terms increases rapidly with the number of predictor variables and the number of categories. In this paper, we propose a new model, called the interaction decomposition model, that allows to visualize the interactions. We argue that with the help of the visualization, the interaction-effect terms are much easier to interpret. We apply our method to predict holiday spending 1 using seven categorical predictor variables.
Springer Series in Statistics, 1997
Springer Series in Statistics, 1997
Springer Series in Statistics, 1997
Springer Series in Statistics, 1997
Springer Series in Statistics, 1997
Springer Series in Statistics, 1997
Springer Series in Statistics, 1997
Springer Series in Statistics, 1997
Springer Series in Statistics, 1997
Springer Series in Statistics, 1997
Springer Series in Statistics, 1997
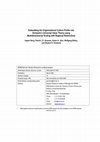
Journal of Personnel Psychology, 2011
Person-organization fit is often measured by the congruence of a person's values and the values t... more Person-organization fit is often measured by the congruence of a person's values and the values that he or she ascribes to the organization. A popular instrument used in this context is the Organizational Culture Profile . The OCP scales its 54 items on eight factors, derived by exploratory factor analysis. We investigate the extent to which the OCP can be embedded into Schwartz's Theory of Universals in Values (TUV) that is formulated in terms of a circumplex in MDS space. To address this question, we develop a nonstandard MDS method that enforces a TUV-based axial regionality onto the solution space together with a permutation test that assesses the consistency of the side constraints with the MDS representation. We find that the OCP can indeed be largely embedded into the TUV. The practical implication is that P-O fit can at least be approximated by the congruence of the person's and the organization's positions on two value dimensions, risk vs. rules and results vs.
Report Econometric Institute Erasmus University Rotterdam, Mar 10, 2004
In analysis of variance, there is usually little attention for interpreting the terms of the effe... more In analysis of variance, there is usually little attention for interpreting the terms of the effects themselves, especially for interaction effects. One of the reasons is that the number of interaction-effect terms increases rapidly with the number of predictor variables and the number of categories. In this paper, we propose a new model, called the interaction decomposition model, that allows to visualize the interactions. We argue that with the help of the visualization, the interaction-effect terms are much easier to interpret. We apply our method to predict holiday spending 1 using seven categorical predictor variables.
Uploads
Papers by Patrick Groenen