Papers by Ralph Vincent Regalado
2018 International Conference on Asian Language Processing (IALP), 2018
Language identification is a text classification task for identifying the language of a given tex... more Language identification is a text classification task for identifying the language of a given text. Several works use this as a preprocessing technique prior to sentiment analysis, mood analysis, and named entity recognition among others. Thus, building an accurate language identification engine is important given that the Philippines is home to more than 170 languages, and is scarce of language documents and resources. We compare machine learning algorithms such as Naive Bayes, Linear Support Vector Machines (SVM), and Random Forest for classification of Philippine languages. Results show that the Linear SVM model had the best performance with 0.97 Fl-score.
Information & Communications Technology in the Philippines: Contemporary Perspectives, 2014
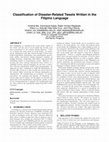
Proceeding of the 12th National Natural Language Processing Research Symposium, Apr 22, 2014
The Philippines is considered the social media capital of the world, and the role of social media... more The Philippines is considered the social media capital of the world, and the role of social media has become even more pronounced in the country during disasters. Twit-ter is among the many forms of social media. As experienced , information and data shared through Twitter have helped individuals, institutions, and organizations (government , public, and private) during emergency response, in making decisions, conducting relief efforts, and practically mobilizing people to humanitarian causes. However, extracting the most relevant information from Twitter is a challenge because natural languages do not have a particular structure immediately useful when programming. An approach we have considered to build the information extraction (IE) system is to first classify the tweets based on the content before extracting the relevant information per classification. The classifications are as follows: (1) caution and advice, (2) casualties and damage, (3) donations, (4) call for help, and (5) others. In this paper, we describe our approaches in the classification, experiments, results, as well as our recommendations to improve the classification module. Our results in the experiments ranges from 84% to 98% f-measure.
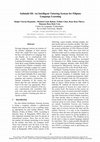
Proceedings of The 2nd Workshop on Natural Language Processing Techniques for Educational Applications
Heritage language learners are learners of the primary language of their parents which they might... more Heritage language learners are learners of the primary language of their parents which they might have been exposed to but have not learned it as a language they can fluently use to communicate with other people. Salinlahi, an Interactive Learning Environment, was developed to teach these young Filipino heritage learners about basic Filipino vocabulary while Salinlahi II included a support for collab-orative learning. With the aim of teaching learners with basic knowledge in Filipino we developed Salinlahi III to teach higher level lessons focusing on Filipino grammar and sentence construction. An internal evaluation of the system has shown that the user interface and feedback of the tutor was appropriate. Moreover , in an external evaluation of the system , experimental and controlled field tests were done and results showed that there is a positive learning gain after using the system.
Subjectivity classification classifies a given
document if it contains subjective information or ... more Subjectivity classification classifies a given
document if it contains subjective information or not, or
identifies which portions of the document are subjective.
This research reports a machine learning approach on
document-level and sentence-level subjectivity classification
of Filipino texts using existing machine learning algorithms
such as C4.5, Naïve Bayes, k-Nearest Neighbor, and Support
Vector Machine. For the document-level classification, result
shows that Support Vector Machines gave the best result
with 95.06% accuracy. While for the sentence-level
classification, Naïve Baves gave the best result with 58.75%
accuracy.
Proceedings of the Workshop on Language Processing and Crisis Information 2013, Oct 14, 2013
The Philippines is considered to be one of the
world’s most disaster prone countries. Since
the u... more The Philippines is considered to be one of the
world’s most disaster prone countries. Since
the use of mobile devices continues to grow,
many generated applications for mobile devices
that will aid during disaster. While most of
the people residing in the urban areas are
mostly smartphone users, people who are living
in the rural areas are still using low-cost
phones. In order to equally provide information
that will be needed on or during disaster,
we created BahaBa a SMS-based route
generation system targeted for mobile devices.
The system accepts a SMS and generates a
template-based response to the sender, containing
instructions on the shortest path to the
nearest safe place in a community.
2014 International Conference on Advanced Computer Science and Information System, 2014
Due to the emigration of millions of Filipinos, the Filipino language is now recognized as one of... more Due to the emigration of millions of Filipinos, the Filipino language is now recognized as one of the major heritage languages. Children of Filipino migrants are exposed to the Filipino language but they did not learn it completely due to emigration and/or formal education in language of country they now live in. Filipino is currently being taught in several States
2012 International Conference on Asian Language Processing, 2012
Subjectivity classification classifies whether a text expresses an opinion or not. Though there a... more Subjectivity classification classifies whether a text expresses an opinion or not. Though there are already existing works in this field especially for the English Language, no reports have been made if these approaches are indeed effective when adapted to the Filipino language. This research reports a feature-based approach for subjectivity classification using existing classifiers such as Naïve Bayes, Bagging, Multilayer perceptron and Random Forest Tree. Result shows that the Bagging classifier gave the best results with 64.7% accuracy.
2013 International Conference on Asian Language Processing, 2013
Uploads
Papers by Ralph Vincent Regalado
document if it contains subjective information or not, or
identifies which portions of the document are subjective.
This research reports a machine learning approach on
document-level and sentence-level subjectivity classification
of Filipino texts using existing machine learning algorithms
such as C4.5, Naïve Bayes, k-Nearest Neighbor, and Support
Vector Machine. For the document-level classification, result
shows that Support Vector Machines gave the best result
with 95.06% accuracy. While for the sentence-level
classification, Naïve Baves gave the best result with 58.75%
accuracy.
world’s most disaster prone countries. Since
the use of mobile devices continues to grow,
many generated applications for mobile devices
that will aid during disaster. While most of
the people residing in the urban areas are
mostly smartphone users, people who are living
in the rural areas are still using low-cost
phones. In order to equally provide information
that will be needed on or during disaster,
we created BahaBa a SMS-based route
generation system targeted for mobile devices.
The system accepts a SMS and generates a
template-based response to the sender, containing
instructions on the shortest path to the
nearest safe place in a community.
document if it contains subjective information or not, or
identifies which portions of the document are subjective.
This research reports a machine learning approach on
document-level and sentence-level subjectivity classification
of Filipino texts using existing machine learning algorithms
such as C4.5, Naïve Bayes, k-Nearest Neighbor, and Support
Vector Machine. For the document-level classification, result
shows that Support Vector Machines gave the best result
with 95.06% accuracy. While for the sentence-level
classification, Naïve Baves gave the best result with 58.75%
accuracy.
world’s most disaster prone countries. Since
the use of mobile devices continues to grow,
many generated applications for mobile devices
that will aid during disaster. While most of
the people residing in the urban areas are
mostly smartphone users, people who are living
in the rural areas are still using low-cost
phones. In order to equally provide information
that will be needed on or during disaster,
we created BahaBa a SMS-based route
generation system targeted for mobile devices.
The system accepts a SMS and generates a
template-based response to the sender, containing
instructions on the shortest path to the
nearest safe place in a community.