Papers by Judith Rousseau

Scandinavian Journal of Statistics, 2005
We are interested in estimating level sets using a Bayesian non-parametric approach, from an inde... more We are interested in estimating level sets using a Bayesian non-parametric approach, from an independent and identically distributed sample drawn from an unknown distribution. Under fairly general conditions on the prior, we provide an upper bound on the rate of convergence of the Bayesian level set estimate, via the rate at which the posterior distribution concentrates around the true level set. We then consider, as an application, the log-spline prior in the two-dimensional unit cube. Assuming that the true distribution belongs to a class of Ho¨lder, we provide an upper bound on the rate of convergence of the Bayesian level set estimates. We compare our results with existing rates of convergence in the frequentist non-parametric literature: the Bayesian level set estimator proves to be competitive and is also easy to compute, which is of no small importance. A simulation study is given as an illustration.

Risk Analysis, 2008
A novel approach to the quantitative assessment of food-borne risks is proposed. The basic idea i... more A novel approach to the quantitative assessment of food-borne risks is proposed. The basic idea is to use Bayesian techniques in two distinct steps: first by constructing a stochastic core model via a Bayesian network based on expert knowledge, and second, using the data available to improve this knowledge. Unlike the Monte Carlo simulation approach as commonly used in quantitative assessment of food-borne risks where data sets are used independently in each module, our consistent procedure incorporates information conveyed by data throughout the chain. It allows “back-calculation” in the food chain model, together with the use of data obtained “downstream” in the food chain. Moreover, the expert knowledge is introduced more simply and consistently than with classical statistical methods. Other advantages of this approach include the clear framework of an iterative learning process, considerable flexibility enabling the use of heterogeneous data, and a justified method to explore the effects of variability and uncertainty. As an illustration, we present an estimation of the probability of contracting a campylobacteriosis as a result of broiler contamination, from the standpoint of quantitative risk assessment. Although the model thus constructed is oversimplified, it clarifies the principles and properties of the method proposed, which demonstrates its ability to deal with quite complex situations and provides a useful basis for further discussions with different experts in the food chain.

Computational Statistics & Data Analysis, 2009
The focus of this paper is on the sensitivity to the specification of the prior in a hidden Marko... more The focus of this paper is on the sensitivity to the specification of the prior in a hidden Markov model describing homogeneous segments of DNA sequences. An intron from the chimpanzee α-fetoprotein gene, which plays an important role in embryonic development in mammals is analysed. Three main aims are considered : (i) to assess the sensitivity to prior specification in Bayesian hidden Markov models for DNA sequence segmentation; (ii) to examine the impact of replacing the standard Dirichlet prior with a mixture Dirichlet prior; and (iii) to propose and illustrate a more comprehensive approach to sensitivity analysis, using importance sampling. It is obtained that (i) the posterior estimates obtained under a Bayesian hidden Markov model are indeed sensitive to the specification of the prior distributions; (ii) compared with the standard Dirichlet prior, the mixture Dirichlet prior is more flexible, less sensitive to the choice of hyperparameters and less constraining in the analysis, thus improving posterior estimates; and (iii) importance sampling was computationally feasible, fast and effective in allowing a richer sensitivity analysis.

Electronic Journal of Statistics, 2010
The Importance Sampling method is used as an alternative approach to MCMC in repeated Bayesian es... more The Importance Sampling method is used as an alternative approach to MCMC in repeated Bayesian estimations. In the particular context of numerous data sets, MCMC algorithms have to be called on several times which may become computationally expensive. Since Importance Sampling requires a sample from a posterior distribution, our idea is to use MCMC to generate only a certain number of Markov chains and use them later in the subsequent IS estimations. For each Importance Sampling procedure, the suitable chain is selected by one of three criteria we present here. The first and second criteria are based on the L 1 norm of the difference between two posterior distributions and their Kullback-Leibler divergence respectively. The third criterion results from minimizing the variance of IS estimate. A supplementary automatic selection procedure is also proposed to choose those posterior for which Markov chains will be generated and to avoid arbitrary choice of importance functions. The featured methods are illustrated in simulation studies on three types of Poisson model: simple Poisson model, Poisson regression model and Poisson regression model with extra Poisson variability. Different parameter settings are considered. * Corresponding author. D. Gajda et al./IS for repeated MCMC for Poisson models 362 AMS 2000 subject classifications: Primary 62F15, 65C05; secondary 65C60.

Journal of Computational and Graphical Statistics, 2012
Since its introduction in the early 90's, the idea of using importance sampling (IS) with Markov ... more Since its introduction in the early 90's, the idea of using importance sampling (IS) with Markov chain Monte Carlo (MCMC) has found many applications. This paper examines problems associated with its application to repeated evaluation of related posterior distributions with a particular focus on Bayesian model validation. We demonstrate that, in certain applications, the curse of dimensionality can be reduced by a simple modification of IS. In addition to providing new theoretical insight into the behaviour of the IS approximation in a wide class of models, our result facilitates the implementation of computationally intensive Bayesian model checks. We illustrate the simplicity, computational savings and potential inferential advantages of the proposed approach through two substantive case studies, notably computation of Bayesian p-values for linear regression models and simulation-based model checking. Supplementary materials including appendices and the R code for Section 3.1.2 are available online.
Scandinavian Journal of Statistics, 2009
Abstract. We consider the consistency of the Bayes factor in goodness of fit testing for a param... more Abstract. We consider the consistency of the Bayes factor in goodness of fit testing for a parametric family of densities against a non-parametric alternative. Sufficient conditions for consistency of the Bayes factor are determined and demonstrated with priors using certain mixtures of triangular densities.

We consider the problem of combining opinions from different experts in an explicitly model-based... more We consider the problem of combining opinions from different experts in an explicitly model-based way to construct a valid subjective prior in a Bayesian statistical approach. We propose a generic approach by considering a hierarchical model accounting for various sources of variation as well as accounting for potential dependence between experts. We apply this approach to two problems. The first problem deals with a food risk assessment problem involving modelling dose-response for Listeria monocytogenes contamination of mice. Two hierarchical levels of variation are considered (between and within experts) with a complex mathematical situation due to the use of an indirect probit regression. The second concerns the time taken by PhD students to submit their thesis in a particular school. It illustrates a complex situation where three hierarchical levels of variation are modelled but with a simpler underlying probability distribution (log-Normal).
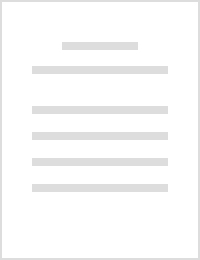
ABSTRACT L'Importance Sampling combiné avec les algorithmes MCMC est proposée ici dans le... more ABSTRACT L'Importance Sampling combiné avec les algorithmes MCMC est proposée ici dans le cas d'estimations Bayésiennes répétées. Dans le cas particulier de nombreux jeux de données simulés sous le même modèle, l'algorithme MCMC doit être utilisé pour chaque jeu de données ce qui peut devenir coûteux en temps calcul. Puisque l'IS nécessite le choix d'une fonction d'importance, nous proposons d'utiliser l'algorithme MCMC pour des jeux de données présélectionnés et ainsi d'obtenir des réalisations de chacune des lois a posteriori correspondantes. Les estimations des paramètres sous les autres jeux de données seront alors faites via IS en ayant préalablement choisi une des lois a posteriori présélectionnées. La fonction d'importance est donc ici la loi a posteriori choisie. Une amélioration de cette procédure consiste à choisir pour chaque jeu de données une fonction d'importance différente parmi des lois a posteriori présélectionnées. Deux critères sont proposés pour ce choix. Le premier critère est basé sur la minimisation de la norme L1 de la différence entre deux densités a posteriori et le deuxième minimise la variance de l'estimation MCMC. Pour éviter le choix arbitraire de l'ensemble de lois a posteriori présélectionnées, une procédure supplémentaire de sélection automatique a été établie. Les approches évoquées ici ont été étudiées via l'étude de simulations sur trois types de modèles Poissonniens : le modèle de Poisson et deux régressions de Poisson avec ou sans extravariabilité.
Uploads
Papers by Judith Rousseau