Papers by Yousif Al-Dunainawi
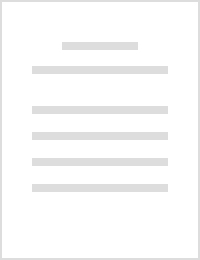
Spe Journal, 2020
In this work, the artificial neural network (ANN) is implemented for prediction slug frequency FS... more In this work, the artificial neural network (ANN) is implemented for prediction slug frequency FS for low viscosity μL flow (μL ≤ 1.6 mPa·s) in vertical, horizontal, and inclined pipes. To the authors' knowledge, no ANN model in the literature exists for predicting FS. The input parameters for the suggested ANN are superficial liquid velocity VSL, pipe diameter D, superficial gas velocity VSG, and pipe inclination ϕ. Measured data (450 data points) are gathered from five different resources for developing the ANN model. The ranges of FS, VSL, D, VSG, and ϕ covered by the data set, are (0.03 to 3.167 1/s), (0.05 to 2.073 m/s), (0.01905 to 0.0779 m), (0.133 to 11.84 m/s), and (0 to 90°), respectively. The most popular transfer functions of tangent sigmoid and linear are used in the hidden and output layers, respectively, whereas the Levenberg and Marquardt back propagation algorithm is conducted to train ANN. The experimental data set is divided into 70% for training, 15% for vali...
Journal of Laser Applications
International Journal of Oil, Gas and Coal Technology

Advances in Science, Technology and Engineering Systems Journal
This paper proposes a novel intelligent technique that has been designed to optimise the performa... more This paper proposes a novel intelligent technique that has been designed to optimise the performance of Software Defined Networks (SDN). The proposed hybrid intelligent system has employed integration of intelligence-based optimisation approaches with the artificial neural network. These heuristic optimisation methods include Genetic Algorithms (GA) and Particle Swarm Optimisation (PSO). These methods were utilised separately in order to select the best inputs to maximise SDN performance. In order to identify SDN behaviour, the neural network model is trained and applied. The maximal optimisation approach has been identified using an analytical approach that considered SDN performance and the computational time as objective functions. Initially, the general model of the neural network was tested with unseen data before implementing the model using GA and PSO to determine the optimal performance of SDN. The results showed that the SDN represented by Artificial Neural Network ANN, and optmised by PSO, generated a better configuration with regards to computational efficiency and performance index.

IEEE Access
Fairness, low latency, and high throughput with low energy consumption are desired attributes for... more Fairness, low latency, and high throughput with low energy consumption are desired attributes for medium access control (MAC) protocols. The IEEE 802.15.4 standard defines the MAC and physical layers standard for IPv6 over low power personal area network (6LoWPAN). When a non-appropriate parameter setting is used, the default MAC parameters generate excessive collisions, packet losses, and high latency under high traffic when a large number of 6LoWPAN nodes being deployed. A search of the literature revealed few studies that investigate the impact of optimizing these parameters to achieve high throughput with minimum latency. This paper proposes a new intelligent approach to selecting the optimal 6LoWPAN MAC layer parameters set; the introduced mechanism depends on artificial neural networks, genetic algorithm, or particles swarm optimization to select and validate the optimized MAC parameters. The obtained simulation results showed that utilizing the optimal MAC parameters improved 6LoWPAN network throughput by 52-63% and reduced the end-to-end delay by 54-65% in which the enhancement percentage depends on the number of deployed sensor nodes in the network. INDEX TERMS 6LoWPAN, artificial neural network, genetic algorithm, particle swarm optimization, MAC parameters.
2016 6th International Conference on Information Communication and Management (ICICM), 2016
2016 SAI Computing Conference (SAI), 2016

IEEE Internet of Things Journal
This paper introduces a proof-of-concept 6LoWPAN testbed to study the integration of programmable... more This paper introduces a proof-of-concept 6LoWPAN testbed to study the integration of programmable network technologies in relaxed throughput and low-power IoT devices. Open source software and hardware platforms are used in the implemented testbed to increase the possibility of future network extension. The proposed architecture offers end-to-end connectivity via the 6LoWPAN gateway to integrate IPv6 hosts and the low data rate devices directly. Nowadays, Software-Defined Networking (SDN) and Network Function Virtualization (NFV) are the most promising technologies for dealing with the massive increase in M2M devices and achieving agile traffic. The developed approach in this paper is entitled tailored Software Defined-Network Function Virtualization (SD-NFV), which is aimed at reducing the end-to-end delay and improving the energy depletion in sensor nodes. Experimental scenarios of the implemented testbed are conducted using a simple sensing application and the obtained results indicate that the introduced approach is appropriate for constrained IoT devices. By utilizing SD-NFV scheme in 6LoWPAN network, the data delivery ratio increased by 5-14%, the node operational time prolonged by 70%, the end-to-end latency for gathering sensor data minimized by ≈160%, and the latency for transmitting control messages to a specified node diminished by ≈63% when compared to a traditional (non SDN-enabled) 6LoWPAN network.

IEEE Access
Generally, it has been taken for granted that green technology provides clean and cheap energy, b... more Generally, it has been taken for granted that green technology provides clean and cheap energy, but often without consideration of the costs. In fact, there are many trade offs concurrent with enabling such technology. Accordingly, this paper evaluates and compares the green energy oriented mobile networks with their traditional counterparts. It presents a mathematical model that helps in understanding the different variables which are necessary to advocate the green/renewable method over the traditional form or vice versa. This research shows that the cost efficiency (CE) of green networks can be relatively high, about twice that of the traditional, which is represented by cloud radio access network. Based on experimental data, this research shows that green technology requires more operational control than the traditional form to produce the same amount of power. With variant sites, cities, countries, geographical areas and equipment manufacturing characteristics, the proposed model can predict the futuristic total green network's trade-offs. By doing so, the service providers, investors or network vendors will be able to decide upon an appropriate balance between both types of networks.

Engineering Applications of Artificial Intelligence
The proposal of this study is a new nonlinear autoregressive moving average, NARMA-L2 controller,... more The proposal of this study is a new nonlinear autoregressive moving average, NARMA-L2 controller, which is based on an adaptive neuro-fuzzy inference system, ANFIS architecture. The new control configuration employs Sugeno-type fuzzy inference system FIS submodels to map input characteristics to the output of a dynamic and nonlinear system. The default hybrid learning algorithm (Backpropagation and Least Square Error) has been carried out as well as particle swarm optimisation (PSO) approach, in order to select the optimal parameters of the ANFIS submodels. Once the system has been modelled efficiently and accurately, the proposed controller is designed by rearranging the generalised FIS submodels. The controller performance is evaluated by simulations conducted on a binary distillation column, which is characterised by a nonlinear and dynamic behaviour. The obtained results show that the PSO-ANFIS based NARMA-L2 achieved more efficient modelling and control performances when compared with other controllers. These controllers include ANN-based NARMA-L2, (PD, PI and PID like) fuzzy-tuned by GA and PSO and traditional PID, which are also implemented to the column for comparison. Stability and robustness of the proposed controller regarding system inputs variance have also been tested by applying asynchronous setpoints of both inputs of the process.
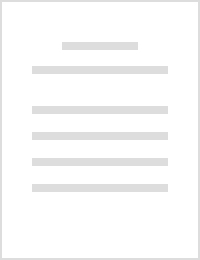
Since carbonate reservoirs are a heterogeneous in nature, therefore the behaviour of petrophysica... more Since carbonate reservoirs are a heterogeneous in nature, therefore the behaviour of petrophysical properties of these reservoirs is a highly nonlinear. There is no close conventional statistical model can describe the behaviour of the relation between cementation factor and rock properties. Artificial Neural Network technique is used in many applications to predict variable that usually cannot be measured in linear modelling. Depending on well logs data, the Interactive Petrophysics software had been used to calculate the petrophysical properties of studied oilfield. In this study, the data sets used for training and testing neural network are provided from well number three of Nasiriya oilfield in the south of Iraq. The neural network model was trained using two different training algorithms; Gradient Descent with Momentum and Levenberg - Marquardt. Porosity, permeability and resistivity formation factor relationships to cementation factor are proposed using artificial neural network model. An efficient performance of excellent prediction of cementation factor has been obtained with less than (1*10-4) mean square error (MSE).
In this paper, PD-fuzzy logic control has been designed to control product compositions of distil... more In this paper, PD-fuzzy logic control has been designed to control product compositions of distillation column. Manual and Particle Swarm Optimisation (PSO) procedures have been applied to tune scaling factors of the controller. Simulation is carried out to minimize the Integral of Square Error (ISE). System behaviour analysis shows very good performance and fast response.
—Genetic Algorithms (GA), Simulated Annealing (SA) and Particle Swarm Optimisation (PSO) are popu... more —Genetic Algorithms (GA), Simulated Annealing (SA) and Particle Swarm Optimisation (PSO) are population-based stochastic search algorithms that categorised into the taxonomy of evolutionary optimisation. These methods have been employed independently to tune a fuzzy controller for maintaining the product compositions of a binary distillation column. An analytical investigation has been conducted to distinguish the optimal tuning approach of the controller among these techniques. Based on simulation results, particle swarm optimisation approach combined with the fuzzy logic controller is identified as a comparatively better configuration regarding to its performance index as well as computational efficiency.

ompositions measurement is a vitally critical issue for the modelling and control of distillation... more ompositions measurement is a vitally critical issue for the modelling and control of distillation process. The product compositions of distillation columns are traditionally measured using indirect techniques via inferring tray compositions from its temperature or by using an online analyser. These techniques were reported as inefficient and relatively slow methods. In this paper, an alternative procedure is presented to predict the compositions of a binary distillation column. Particle swarm opti- misation based artificial neural network PSO-ANN is trained by different algorithms and tested by new unseen data to check the generality of the proposed method. Particle swarm optimisation is utilised, here, to choose the optimal topology of the network. The simulation results have indicated a reasonable accuracy of prediction with a minimal error between the predicted and simulated data of the column.

A cantilever beam is made from composite material which is consist of (matrix: polyester) and (pa... more A cantilever beam is made from composite material which is consist of (matrix: polyester) and (particles: Silicon-Carbide) with different volume fraction of particles. A force is applied at the free end of beam with different values. The experimental maximum deflection of beam which occurs at the point of the applied load is recorded. The deflection and slope of beam are analyzed by using FEM modeling. MATLAB paltform is built to assemble the equations, vector and matrix of FEM and solving the unknown variables (deflection and slope) at each node. Also ANSYS platform is used to modeling beam in finite element and solve the problem. The numerical methods are used to compare the results with the theoretical and experimental data. A good agreement is observed between the above methods. The Increase in volume fraction of particles results in increasing the modulus of elasticity and decreasing the deflection of beam. An equation is suggested for modulus of elasticity as functions of volume fraction.
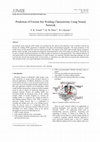
An artificial neural network (ANN) model was developed for the analysis and simulation of the cor... more An artificial neural network (ANN) model was developed for the analysis and simulation of the correlation between the friction stir welding (FSW) parameters of aluminum (Al) plates and mechanical properties. The input parameters of the model consist of weld speed (Ws) and tool rotation speed (Rs). The outputs of the ANN model include property parameters namely: tensile strength, yield strength and elongation. Good performance of the ANN model was achieved. The model can be used to calculate mechanical properties of welded Al plates as functions of weld speed and Rs. The combined influence of weld speed and Rs on the mechanical properties of welded Al plates was simulated. Simulated annealing technique was used to prevent the network from getting stuck in local minima. A comparison was made between measured and calculated data. The calculated results were in good agreement with measured data.

The present study develops an artificial neural network (ANN) to model an analysis and a simulati... more The present study develops an artificial neural network (ANN) to model an analysis and a simulation of the correlation between the average corrosion rate carbon steel and the effective parameter Reynolds number (Re), water concentration (Wc) % temperature (T o ) with constant of PH 7 . The water, produced fom oil in Kirkuk oil field in Iraq from well no. k184-Depth2200ft., has been used as a corrosive media and specimen area (400 mm 2 ) for the materials that were used as low carbon steel pipe. The pipes are supplied by Doura Refinery . The used flow system is all made of Q.V.F glass, and the circulation of the two -phase (liquid -liquid ) is affected using a Q.V.F pump .The input parameters of the model consists of Reynolds number , water concentration and temperature. The output is average corrosion rate .The performance of the two training algorithms, gradient descent with momentum and Levenberg-Marquardt, are compared to select the most suitable training algorithm for corrosion rate model. The model can be used to calculate the average corrosion rate properties of carbon steel alloy as functions of Reynolds number, water concentration and temperature. Accordingly, the combined influence of these effective parameters and the average corrosion rate is simulated. The results show that the corrosion rate increases with the increase of temperature, Reynolds number and the increase of water concentration.
Uploads
Papers by Yousif Al-Dunainawi