Papers by Farhana Zulkernine
IEEE Access
This work involved human subjects in its research. Approval of all ethical and experimental proce... more This work involved human subjects in its research. Approval of all ethical and experimental procedures and protocols was granted by Queen'
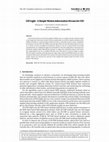
Proceedings of the Canadian Conference on Artificial Intelligence
Vision-based Human Activity Recognition (HAR) aims to recognize human activities based on the ana... more Vision-based Human Activity Recognition (HAR) aims to recognize human activities based on the analysis of video data, and has extensive applications in modern industry and human life. Inflated 3D (I3D) is a deep learning architecture that is commonly used for action recognition by using two-stream video data: RGB stream and optical flow stream. I3D achieved great success on a variety of action recognition benchmarks. However, the use of optical flow incurs high computational cost, making the approach unsuitable for real-time applications. We propose an alternative simple motion information extractor to replace the optical flow branch and reduce the computational cost. It is a modified I3D that uses 128 frames of 112x112 images as input. The low spatial resolution and long temporal range of the proposed I3D RGB stream can reduce the spatial information and enhance the motion information. Experiments show that this simple motion stream can increase the accuracy of the original I3D spatial stream by 4.09% on the Kinetics 400 dataset.
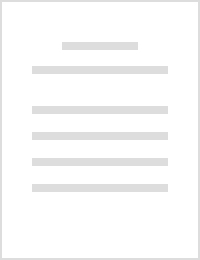
Family Practice
Background Posttraumatic stress disorder (PTSD) has significant morbidity and economic costs. Thi... more Background Posttraumatic stress disorder (PTSD) has significant morbidity and economic costs. This study describes the prevalence and characteristics of patients with PTSD using primary care electronic medical record (EMR) data. Methods This retrospective cross-sectional study used EMR data from the Canadian Primary Care Sentinel Surveillance Network (CPCSSN). This study included 1,574 primary care providers located in 7 Canadian provinces. There were 689,301 patients that visited a CPCSSN provider between 1 January 2017 and 31 December 2019. We describe associations between PTSD and patient characteristics using descriptive statistics, chi-square, and multiple logistic regression models. Results Among the 689,301 patients included, 8,817 (1.3%, 95% CI 1.2–1.3) had a diagnosis of PTSD. On multiple logistic regression analysis, patients with depression (OR 4.4, 95% CI 4.2–4.7, P < 0.001), alcohol abuse/dependence (OR 1.7, 95% CI 1.6–1.9, P < 0.001), and/or drug abuse/dependence...
JCO clinical cancer informatics, Sep 1, 2022
IEEE Transactions on Network and Service Management, Jun 1, 2023
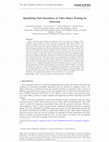
Object detection and tracking are important areas of research in computer vision. Computer vision... more Object detection and tracking are important areas of research in computer vision. Computer vision solutions to object detection are typically single-frame solutions. To perform tracking by detection, these solutions typically do object detection on a perframe basis, thus losing any temporal information from previous frames. Many multiobject tracking solutions report the average precision performance on video datasets, but they do not evaluate the temporal qualities of these solutions. In video, not only the detection of objects is important but the temporal motion attributes of an object's path, such as its velocity, acceleration, and jerk, are important as well. Many implementations of Object Tracking by Detection systems have run into the problem of motion smoothing for bounding box paths. This paper focuses on quantifying the smoothness of detected object paths within some temporal window. We propose using two smoothness metrics from the field of biokinematics and adapt them for use with detections. Finally, using these metrics, we evaluate the ground truth and two popular object detectors, at the time of experimentation (YOLOv3 and Retinanet), on the entire MOT17 dataset. The results show that the metrics are useful in determining object smoothness, and provide us with an additional approach to evaluate an algorithm's performance in object tracking. The experiments also demonstrate that YOLOv3 produces smoother bounding boxes than Retinanet. All supplemental graphs and data are shown in our appendix 1 .
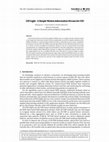
Vision-based Human Activity Recognition (HAR) aims to recognize human activities based on the ana... more Vision-based Human Activity Recognition (HAR) aims to recognize human activities based on the analysis of video data, and has extensive applications in modern industry and human life. Inflated 3D (I3D) is a deep learning architecture that is commonly used for action recognition by using two-stream video data: RGB stream and optical flow stream. I3D achieved great success on a variety of action recognition benchmarks. However, the use of optical flow incurs high computational cost, making the approach unsuitable for real-time applications. We propose an alternative simple motion information extractor to replace the optical flow branch and reduce the computational cost. It is a modified I3D that uses 128 frames of 112x112 images as input. The low spatial resolution and long temporal range of the proposed I3D RGB stream can reduce the spatial information and enhance the motion information. Experiments show that this simple motion stream can increase the accuracy of the original I3D spatial stream by 4.09% on the Kinetics 400 dataset.
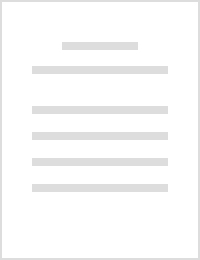
Data is encoded and stored in a wide variety of file types having different schema. As a result, ... more Data is encoded and stored in a wide variety of file types having different schema. As a result, File Type Identification (FTI) has become essential in data pre-processing and security. FTI traditionally involves interpretation of the key signature bytes found in the beginning of each file. However, when these signature bytes are corrupted, the contents of the file must be analysed to correctly identify the file type. Recently, studies leveraging machine learning algorithms have seen great success in content-based classification. In addition, when the signature bytes are not damaged, Commercial Off The Shelf (COTS) systems like Libmagic are preferred. We present an end-to-end framework combining signature and content-based file type classification. We utilize Libmagic in combination with machine learning algorithms, implemented in Spark, to accurately identify file types. The results show that our system can successfully identify the data schema and file types using a pool of workflow templates.
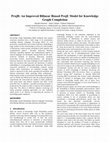
arXiv (Cornell University), Aug 15, 2022
Knowledge Graph Embedding (KGE) methods have gained enormous attention from a wide range of AI co... more Knowledge Graph Embedding (KGE) methods have gained enormous attention from a wide range of AI communities including Natural Language Processing (NLP) for text generation, classification and context induction. Embedding a huge number of interrelationships in terms of a small number of dimensions, require proper modeling in both cognitive and computational aspects. Recently, numerous objective functions regarding cognitive and computational aspects of natural languages are developed. Among which are the stateof-the-art methods of linearity, bilinearity, manifoldpreserving kernels, projection-subspace, and analogical inference. However, the major challenge of such models lies in their loss functions that associate the dimension of relation embeddings to corresponding entity dimension. This leads to inaccurate prediction of corresponding relations among entities when counterparts are estimated wrongly. ProjE model is improved in this work regarding all translative and bilinear interactions while capturing entity nonlinearity. Experimental results on benchmark Knowledge Graphs (KGs) such as FB15K and WN18 show that the proposed approach outperforms the state-of-the-art models in entity prediction task using linear and bilinear methods and other recent powerful ones. In addition, a parallel processing structure is proposed for the model in order to improve the scalability on large KGs. The effects of different adaptive clustering and newly proposed sampling approaches are also explained which prove to be effective in improving the accuracy of knowledge graph completion.
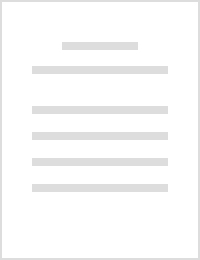
Family Practice, Dec 9, 2022
Background Posttraumatic stress disorder (PTSD) has significant morbidity and economic costs. Thi... more Background Posttraumatic stress disorder (PTSD) has significant morbidity and economic costs. This study describes the prevalence and characteristics of patients with PTSD using primary care electronic medical record (EMR) data. Methods This retrospective cross-sectional study used EMR data from the Canadian Primary Care Sentinel Surveillance Network (CPCSSN). This study included 1,574 primary care providers located in 7 Canadian provinces. There were 689,301 patients that visited a CPCSSN provider between 1 January 2017 and 31 December 2019. We describe associations between PTSD and patient characteristics using descriptive statistics, chi-square, and multiple logistic regression models. Results Among the 689,301 patients included, 8,817 (1.3%, 95% CI 1.2–1.3) had a diagnosis of PTSD. On multiple logistic regression analysis, patients with depression (OR 4.4, 95% CI 4.2–4.7, P &lt; 0.001), alcohol abuse/dependence (OR 1.7, 95% CI 1.6–1.9, P &lt; 0.001), and/or drug abuse/dependence (OR 2.6, 95% CI 2.5–2.8, P &lt; 0.001) had significantly higher odds of PTSD compared with patients without those conditions. Patients residing in community areas considered the most material deprived (OR 2.1, 95% CI 1.5–2.1, P &lt; 0.001) or the most socially deprived (OR 2.8, 95% CI 2.7–5.3, P &lt; 0.001) had higher odds of being diagnosed with PTSD compared with patients in the least deprived areas. Conclusions The prevalence of PTSD in Canadian primary care is 1.3% (95% CI 1.25–1.31). Using EMR records we confirmed the co-occurrence of PTSD with other mental health conditions within primary care settings suggesting benefit for improved screening and evidence-based resources to manage PTSD.
IGI Global eBooks, Jan 18, 2011
IEEE Access, 2022
This work involved human subjects in its research. Approval of all ethical and experimental proce... more This work involved human subjects in its research. Approval of all ethical and experimental procedures and protocols was granted by Queen'
Lecture Notes in Computer Science, 2023
IEEE Transactions on Network and Service Management, Dec 1, 2022
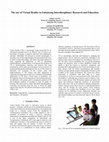
arXiv (Cornell University), Sep 23, 2018
Virtual Reality (VR) is increasingly being recognized for its educational potential and as an eff... more Virtual Reality (VR) is increasingly being recognized for its educational potential and as an effective way to convey new knowledge to people, it supports interactive and collaborative activities. Affordable VR powered by mobile technologies is opening a new world of opportunities that can transform the ways in which we learn and engage with others. This paper reports our study regarding the application of VR in stimulating interdisciplinary communication. It investigates the promises of VR in interdisciplinary education and research. The main contributions of this study are (i) literature review of theories of learning underlying the justification of the use of VR systems in education, (ii) taxonomy of the various types and implementations of VR systems and their application in supporting education and research (iii) evaluation of educational applications of VR from a broad range of disciplines, (iv) investigation of how the learning process and learning outcomes are affected by VR systems, and (v) comparative analysis of VR and traditional methods of teaching in terms of quality of learning. This study seeks to inspire and inform interdisciplinary researchers and learners about the ways in which VR might support them and also VR software developers to push the limits of their craft.
2023 IEEE 47th Annual Computers, Software, and Applications Conference (COMPSAC)
2023 IEEE 47th Annual Computers, Software, and Applications Conference (COMPSAC)
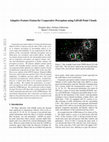
2023 IEEE/CVF Winter Conference on Applications of Computer Vision (WACV)
Cooperative perception allows a Connected Autonomous Vehicle (CAV) to interact with the other CAV... more Cooperative perception allows a Connected Autonomous Vehicle (CAV) to interact with the other CAVs in the vicinity to enhance perception of surrounding objects to increase safety and reliability. It can compensate for the limitations of the conventional vehicular perception such as blind spots, low resolution, and weather effects. An effective feature fusion model for the intermediate fusion methods of cooperative perception can improve feature selection and information aggregation to further enhance the perception accuracy. We propose adaptive feature fusion models with trainable feature selection modules. One of our proposed models Spatial-wise Adaptive feature Fusion (S-AdaFusion) outperforms all other State-of-the-Arts (SO-TAs) on two subsets of the OPV2V dataset: Default CARLA Towns for vehicle detection and the Culver City for domain adaptation. In addition, previous studies have only tested cooperative perception for vehicle detection. A pedestrian, however, is much more likely to be seriously injured in a traffic accident. We evaluate the performance of cooperative perception for both vehicle and pedestrian detection using the CODD dataset. Our architecture achieves higher Average Precision (AP) than other existing models for both vehicle and pedestrian detection on the CODD dataset. The experiments demonstrate that cooperative perception also improves the pedestrian detection accuracy compared to the conventional single vehicle perception process.
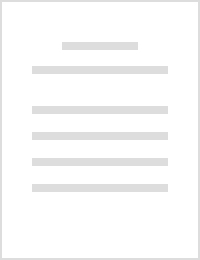
2020 IEEE Intl Conf on Dependable, Autonomic and Secure Computing, Intl Conf on Pervasive Intelligence and Computing, Intl Conf on Cloud and Big Data Computing, Intl Conf on Cyber Science and Technology Congress (DASC/PiCom/CBDCom/CyberSciTech)
This project addresses a classic employment law question in Canada and elsewhere using machine le... more This project addresses a classic employment law question in Canada and elsewhere using machine learning approach: how do we know whether a worker is an employee or an independent contractor? This is a central issue for self-represented litigants insofar as these two legal categories entail very different rights and employment protections. In this interdisciplinary research study, we collaborated with the Conflict Analytics Lab to develop machine learning models aimed at determining whether a worker is an employee or an independent contractor. We present a number of supervised learning models including a neural network model that we implemented using data labeled by law researchers and compared the accuracy of the models. Our neural network model achieved an accuracy rate of 91.5%. A critical discussion follows to identify the key features in the data that influence the accuracy of our models and provide insights about the case outcomes.
Uploads
Papers by Farhana Zulkernine