Papers by Joel Quintanilla

Scientia Iranica, 2011
Breast cancer is one of the leading causes of female mortality in the world, and early detection ... more Breast cancer is one of the leading causes of female mortality in the world, and early detection is an important means of reducing the mortality rate. The presence of microcalcification clusters has been considered as a very important indicator of malignant types of breast cancer, and its detection is important to prevent and treat the disease. This paper presents an effective approach, in order to detect microcalcification clusters in digitized mammograms, based on the synergy of image processing and partitional (hard and fuzzy) clustering techniques. Mathematical morphology has been used for image processing, and is used in this work as a first step, with the purpose of enhancing the contrast of microcalcifications. Image segmentation is an important task in the field of image processing, in order to identify regions with the same features. In the second step, we use image segmentation, using three partitional, hard and fuzzy clustering algorithms, such as k-Means, Fuzzy c-Means and Possibilistic Fuzzy c-Means, in order to make a comparison of the advantages and drawbacks offered by these algorithms, and which should help to improve the detection of microcalcification clusters in digitized mammograms.
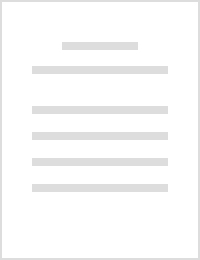
International Journal of Intelligent Computing in Medical Sciences & Image Processing, 2013
ABSTRACT This paper presents a novel method for the automatic detection of microcalcifications in... more ABSTRACT This paper presents a novel method for the automatic detection of microcalcifications in regions of interest images. Automatic detection method is implemented by feature extraction and sub-segmentation steps. The feature extraction step is improved using a top-hat transform such that microcalcifications can be highlighted. In a second step a sub-segmentation method based on the possibilistic fuzzy c-means clustering algorithm is applied in order to segment the images and as a way to identify the atypical pixels inside the regions of interest as the pixels representing microcalcifications. Once the pixels representing these objects have been identified, an artificial neural network model is used to learn the relations between atypical pixels and microcalcifications, such that the model can be used for aid diagnosis, and a medical could determine if these regions of interest are benign or malignant. So, as the results show, the proposed approach is a good alternative for the detection of suspicious regions, which could be of great help for medical diagnosis.
La serie del libro ofrecerá los volúmenes de contribuciones seleccionadas de investigadores que c... more La serie del libro ofrecerá los volúmenes de contribuciones seleccionadas de investigadores que contribuyan a la actividad de difusión científica de ECORFAN en su área de investigación en Ingeniería. Además de tener una evaluación total, en las manos de los editores de la Universidad Mayor, Real y Pontificia de San Francisco Xavier de Chuquisaca y Universidad Tecnologia del Sureste de Guanajuato que colaboraron con calidad y puntualidad en sus capítulos, cada contribución individual fue arbitrada a estándares internacionales (LATINDEX-DIALNET-ResearchGate-DULCINEA-HISPANA-Sudoc-SHERPA-UNIVERSIA-e-Revistas), la serie propone así a la comunidad académica , los informes recientes sobre los nuevos progresos en las áreas más interesantes y prometedoras de investigación en Ingeniería.
Journal of Scientific and Technical Applications, Jun 30, 2019
Procesamiento de imagen, Mejora de contraste, Mamografías
EURASIP journal on advances in signal processing, Dec 1, 2011
A new method for detecting microcalcifications in regions of interest (ROIs) extracted from digit... more A new method for detecting microcalcifications in regions of interest (ROIs) extracted from digitized mammograms is proposed. The top-hat transform is a technique based on mathematical morphology operations and, in this paper, is used to perform contrast enhancement of the mi-crocalcifications. To improve microcalcification detection, a novel image sub-segmentation approach based on the possibilistic fuzzy c-means algorithm is used.
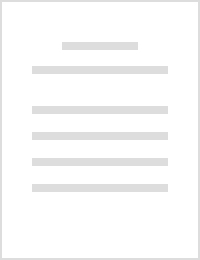
The feature selection has been widely used to reduce the data dimensionality. Data reduction impr... more The feature selection has been widely used to reduce the data dimensionality. Data reduction improve the classification performance, the approximation function, and pattern recognition systems in terms of speed, accuracy and simplicity. A strategy to reduce the number of features in local search are the sequential search algorithms. In this work is presented a feature selection method based on Sequential Forward Selection (SFS) and Feed Forward Neural Network (FFNN) to estimate the prediction error as a selection criterion. Three well-known database have been used to test the SFS-FFNN with Artificial Metaplasticity on Perceptron Multilayer (AMMLP). The AMMLP is a new method applied for classification of patterns. The results obtained by SFS-FFNN with AMMLP in classification accuracy are superior than obtained by conventional BP algorithm and other recent feature selection algorithms applied to the same database. By these reasons the proposed method SFS-FFNN with AMMLP is an interesting alternative to reduce the data dimensionality and provide a high accuracy.
Breast cancer is one of the leading causes to women mortality in the world. Cluster of Microcalci... more Breast cancer is one of the leading causes to women mortality in the world. Cluster of Microcalcifications (MCC) in mammograms can be an important early sign of breast cancer, the detection is important to prevent and treat the disease. In this paper, we present a novel method for the detection of MCC in mammograms which consists of image enhancement by histogram adaptive equalization technique, MCC edge detection by Coordinate Logic Filters (CLF), generation, clustering and labelling of suboptimal features vectors by means of Self Organizing Map (SOM) Neural Network. Like comparison we applied an unsupervised clustering K-means in the stage of labelling of our method. In the labelling stage, we obtain better results with the proposed SOM Neural Network compared with the k-means algorithm. Then, we show that the proposed method can locate MCCs in an efficient way.
In this paper, Ant Colony System (ACS) algorithm is applied for edge detection in grayscale image... more In this paper, Ant Colony System (ACS) algorithm is applied for edge detection in grayscale images. The novelty of the proposed method is to extract a set of images from the original grayscale image using Multiscale Adaptive Gain for image contrast enhancement and then apply the ACS algorithm to detect the edges on each of the extracted images. The resulting set of images represents the pheromone trails matrices which are summed to produce the output image. The image contrast enhancement makes ACS algorithm more effective when accumulating pheromone trails on the true edge pixels. The results of the experiments are presented to confirm the effectiveness of the proposed method.
... In Section IV we introduce the NNs computational model with embedded neuronal plasticity prop... more ... In Section IV we introduce the NNs computational model with embedded neuronal plasticity properties, the AMP algorithm and network structure ... The features can be calculated from the knot characteristics such as the size, shape, density, and smoothness of borders, etc. ...
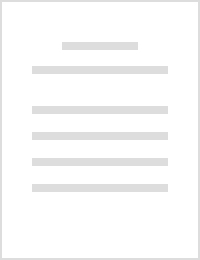
Breast cancer is one of the leading causes to women mortality in the world. Clusters of Microcalc... more Breast cancer is one of the leading causes to women mortality in the world. Clusters of Microcalcifications (MCCs) in mammograms can be an important early sign of breast cancer, the detection is important to prevent and treat the disease. Coordinate Logic Filters (CLF), are very efficient in digital signal processing applications, such as noise removal, magnification, opening, closing, skeletonization, and coding, as well as in edge detection, feature extraction, and fractal modelling. This paper presents an edge detector of MCCs in Regions of Interest (ROI) from mammograms using a novel combination. The edge detector consist in the combination of image enhancement by histogram adaptive technique, a Self Organizing Map (SOM) Neural Network and CLF. The experiment results show that the proposed method can locate MCCs edges. Moreover, the proposed method is quantitatively evaluated by Pratt’s figure of merit together with two widely used edge detectors and visually compared, achieving the best results.

This work presents a method to detect Microcalcifications in Regions of Interest from digitized m... more This work presents a method to detect Microcalcifications in Regions of Interest from digitized mammograms. The method is based mainly on the combination of Image Processing, Pattern Recognition and Artificial Intelligence. The Top-Hat transform is a technique based on mathematical morphology operations that, in this work is used to perform contrast enhancement of microcalcifications in the region of interest. In order to find more or less homogeneous regions in the image, we apply a novel image sub-segmentation technique based on Possibilistic Fuzzy c-Means clustering algorithm. From the original region of interest we extract two window-based features, Mean and Deviation Standard, which will be used in a classifier based on a Artificial Neural Network in order to identify microcalcifications. Our results show that the proposed method is a good alternative in the stage of microcalcifications detection, because this stage is an important part of the early Breast Cancer detection.

Breast cancer is one of the leading causes to women mortality in the world and early detection is... more Breast cancer is one of the leading causes to women mortality in the world and early detection is an important means to reduce the mortality rate. The presence of microcalcifications clusters has been considered as a very important indicator of malignant types of breast cancer and its detection is important to prevent and treat the disease. This paper presents an alternative and effective approach in order to detect micro calcifications clusters in digitized mammograms based on the synergy of the image processing, pattern recognition and artificial intelligence. The mathematical morphology is an image processing technique used for the purpose of image enhancement. A k means algorithm is used to cluster the data based on the features vectors and finally an artificial neural network-based classifier is applied and the classification performance is evaluated by a ROC curve. Experimental results indicate that the percentage of correct classification was 99.72%, obtaining 100% true positive (sensitivity) and 99.67% false positive (specificity), with the best classifier proposed. In case of the best classifier, we obtained a performance evaluation of classification of Az = 0.9875.
In this paper a method to extract and build patterns to model microcalcifications from digitized ... more In this paper a method to extract and build patterns to model microcalcifications from digitized mammography is presented. The proposed method consist in a combination of two steps, in the first one, a feature extraction method is applied using multiscale wavelet image processing, and is combined with a Self Organizing Neural Network to solve the segmentation image problem. In the second one, a feature selection method is considered applying a Generalized Regression Neural Networks (GRNN) in order to obtain an optimal and a short input vector for classifier design proposes. Optimal results were obtained and compared with others.

Neurocomputing, 2011
A novel improvement in neural network training for pattern classification is presented in this pa... more A novel improvement in neural network training for pattern classification is presented in this paper. The proposed training algorithm is inspired by the biological metaplasticity property of neurons and Shannon's information theory. This algorithm is applicable to artificial neural networks (ANNs) in general, although here it is applied to a multilayer perceptron (MLP). During the training phase, the artificial metaplasticity multilayer perceptron (AMMLP) algorithm assigns higher values for updating the weights in the less frequent activations than in the more frequent ones. AMMLP achieves a more efficient training and improves MLP performance. The well-known and readily available Wisconsin Breast Cancer Database (WBCD) has been used to test the algorithm. Performance of the AMMLP was tested through classification accuracy, sensitivity and specificity analysis, and confusion matrix analysis. The results obtained by AMMLP are compared with the backpropagation algorithm (BPA) and other recent classification techniques applied to the same database. The best result obtained so far with the AMMLP algorithm is 99.63%. .es (A. Marcano-Cedeñ o), [email protected] (J. Quintanilla-Domínguez), [email protected] (D. Andina). Please cite this article as: A. Marcano-Cedeñ o, et al., Breast cancer classification applying artificial metaplasticity algorithm, Neurocomputing (2010),

Over the last ten years, Salamanca has been considered among the most polluted cities in México.... more Over the last ten years, Salamanca has been considered among the most polluted cities in México. Nowadays, there is an Automatic Environmental Monitoring Network (AEMN) which measures air pollutants (Sulphur Dioxide (SO2), Particular Matter (PM10), Ozone (O3), etc.), as well as environmental variables (wind speed, wind direction, temperature, and relative humidity), and it takes a sample of the variables every minute. The AEM Network is mainly based on three monitoring stations located at Cruz Roja, DIF, and Nativitas. In this work, we use the PFCM (Possibilistic Fuzzy c Means) clustering algorithm as a mean to get a combined measure, from the three stations, looking to provide a tool for better management of contingencies in the city, such that local or general action can be taken in the city according to the pollution level given by each station and the combined measure. Besides, we also performed an analysis of correlation between pollution and environmental variables. The results show a significative correlation between pollutant concentrations and some environmental variables. So, the combined measure and the correlations can be used for the establishment of general contingency thresholds.

Mass detection in mammography is a complex and challenge problem for digital image processing. Pa... more Mass detection in mammography is a complex and challenge problem for digital image processing. Partitional clustering algorithms are a good alternative for automatic detection of such elements, but have the disadvantage of having to segment an image into a number of regions, the number of which is unknown in advance, in addition to discrete approximations of the regions of interest. In this work we use a method of image sub-segmentation to identify possible masses in mammography. The advantage of this method is that the number of regions to segment the image is a known value so the algorithm is applied only once. Additionally, there is a parameter α that can change between 1 and 0 in a continuous way, offering the possibility of a continuous and more accurate approximation of the region of interest. Finally, since the identification of masses is based on the internal similarity of a group data, this method offers the possibility to identify such objects even from a small number of pixels in digital images. This paper presents an illustrative example using the traditional segmentation of images and the sub-segmentation method, which highlights the potential of the alternative we propose for such problems.
In this work we propose a method for subsegmentation of images using the PFCM clustering algorith... more In this work we propose a method for subsegmentation of images using the PFCM clustering algorithm. The sub-segmentation consists of ſnding, within the clusters found using the segmentation process, those data less representative, or atypical data, belonging to the clusters. These data represent, in many cases, the zones of interest during image analysis. Two different examples are used in order to show the results, and the advantages of identifying those elements of data forced to belong to a cluster, of which they are the less representative and, therefore may contain information of great interest in particular applications.
This paper presents the results of some partitional clustering algorithms applied to the segmenta... more This paper presents the results of some partitional clustering algorithms applied to the segmentation of color images in the RGB space. As more information is involved in the algorithm, and the distance measure is more flexible, the better the results. The selected algorithms for this work are the K-means, the FCM, the GK-B, and the GKPFCM. The GKPFCM gives the better results when all the algorithms are applied to the segmentation of two images, an image of bananas and the other one of tomates at different stages of ripeness in both cases. The results are interesting as it is possible to identify the objects, to determine the degree of ripeness, and to estimate the amount and proportion of ripe objects for a possible decision-making.

Expert Systems With Applications, 2011
The correct diagnosis of breast cancer is one of the major problems in the medical field. From th... more The correct diagnosis of breast cancer is one of the major problems in the medical field. From the literature it has been found that different pattern recognition techniques can help them to improve in this domain. These techniques can help doctors form a second opinion and make a better diagnosis. In this paper we present a novel improvement in neural network training for pattern classification. The proposed training algorithm is inspired by the biological metaplasticity property of neurons and Shannon's information theory. During the training phase the Artificial metaplasticity Multilayer Perceptron (AMMLP) algorithm gives priority to updating the weights for the less frequent activations over the more frequent ones. In this way metaplasticity is modeled artificially. AMMLP achieves a more effcient training, while maintaining MLP performance. To test the proposed algorithm we used the Wisconsin Breast Cancer Database (WBCD). AMMLP performance is tested using classification accuracy, sensitivity and specificity analysis, and confusion matrix. The obtained AMMLP classification accuracy of 99.26%, a very promising result compared to the Backpropagation Algorithm (BPA) and recent classification techniques applied to the same database.
Breast cancer is one of the leading causes to women mortality in the world. Cluster of Microcalci... more Breast cancer is one of the leading causes to women mortality in the world. Cluster of Microcalcifications (MCCs) in mammograms can be an important early sign of breast cancer, the detection is important to prevent and treat the disease. In this paper, we present a novel method for the ...
Uploads
Papers by Joel Quintanilla