Papers by Jose M. Ferrandez
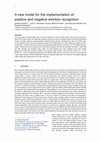
The large range of potential applications, not only for patients but also for healthy people, tha... more The large range of potential applications, not only for patients but also for healthy people, that could be achieved by affective BCI (aBCI) makes more latent the necessity of finding a commonly accepted protocol for real-time EEG-based emotion recognition. Based on wavelet package for spectral feature extraction, attending to the nature of the EEG signal, we have specified some of the main parameters needed for the implementation of robust positive and negative emotion classification. 12 seconds has resulted as the most appropriate sliding window size; from that, a set of 20 target frequency-location variables have been proposed as the most relevant features that carry the emotional information. Lastly, QDA and KNN classifiers and population rating criterion for stimuli labeling have been suggested as the most suitable approaches for EEG-base emotion recognition. The proposed model reached a mean accuracy of 98% (s.d. 1.4) and 98.96% (s.d. 1.28) in a subject-dependent approach for ...
The construction of a system for aiding some kind of blindness is proposed. It consists in a bioi... more The construction of a system for aiding some kind of blindness is proposed. It consists in a bioinspired retina model into a reconfigurable architecture which is connected to an augmented reality device based on see-through technology. This device will permit the integration of real world images to the ones obtained by the neuromorphic retina. This system will provide a local gain control, in addition of a bioinspired preprocessing, FPGAs plasticity, and the miniaturization of the components, including low power devices. These characteristics are highly desirable in some low vision supporting systems.
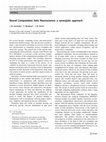
For several decades, computing science and neuroscience benefit from fruitful synergy. This speci... more For several decades, computing science and neuroscience benefit from fruitful synergy. This special issue searches to make a step forward by providing an overview of how the new advancements in computing sciences strengthen and enrich this synergy. The general aim of this special issue, continuing the neurocybernetic concepts from Wiener and W.S. McCulloch, is to present a wider and more comprehensive relation of the computational paradigm (CP), with emerging neuroscience studies. The secondary objectives are also (1) to help neuroscience and cognitive science, by explaining the latter as a result of the former, (2) to establish an interaction framework between neural computation and neuroscience by posing a series of appropriate questions in both directions of the interaction, from artificial systems to neural systems, and from neural systems to artificial systems. Nowadays, machine learning holds great promise in the development of new models and theories in the field of neuroscie...
There are differences between healthy subjects that present a normal aging and subjects that even... more There are differences between healthy subjects that present a normal aging and subjects that even without showing symptoms of neurodegenerative diseases are already in the previous stages of the disease, when it comes to process emotions. In this way, it would be possible to use the recognition of emotions as a method of aid for the diagnosis of neurodegenerative diseases in early stages. However, apathy, mobility difficulties, and changes in emotional regulation associated with normal aging make it difficult to detect emotional disturbances. Therefore, in this article we propose the development of experimentation protocols that allow the evaluation of the emotional state of subjects through based-home affective computing technologies.
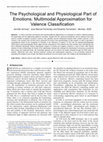
In order to develop more precise and functional affective applications, it is necessary to achiev... more In order to develop more precise and functional affective applications, it is necessary to achieve a balance between the psychology and the engineering applied to emotions. Signals from the central and peripheral nervous systems have been used for emotion recognition purposes, however, their operation and the relationship between them remains unknown. In this context, in the present work we have tried to approach the study of the psychobiology of both systems in order to generate a computational model for the recognition of emotions in the dimension of valence. To this end, the electroencephalography (EEG) signal, electrocardiography (ECG) signal and skin temperature of 24 subjects have been studied. Each methodology has been evaluated individually, finding characteristic patterns of positive and negative emotions in each of them. After feature selection of each methodology, the results of the classification showed that, although the classification of emotions is possible at both ce...

ABSTRACTThe large range of potential applications, not only for patients but also for healthy peo... more ABSTRACTThe large range of potential applications, not only for patients but also for healthy people, that could be achieved by affective BCI (aBCI) makes more latent the necessity of finding a commonly accepted protocol for real-time EEG-based emotion recognition. Based on wavelet package for spectral feature extraction, attending to the nature of the EEG signal, we have specified some of the main parameters needed for the implementation of robust positive and negative emotion classification. 12 seconds has resulted as the most appropriate sliding window size; from that, a set of 20 target frequency-location variables have been proposed as the most relevant features that carry the emotional information. Lastly, QDA and KNN classifiers and population rating criterion for stimuli labeling have been suggested as the most suitable approaches for EEG-base emotion recognition. The proposed model reached a mean accuracy of 98% (s.d. 1.4) and 98.96% (s.d. 1.28) in a subject-dependent appro...
Lecture Notes in Computer Science, 2015
As the population increases in the world, the ratio of health carers is rapidly decreasing. There... more As the population increases in the world, the ratio of health carers is rapidly decreasing. Therefore, there is an urgent need to create new technologies to monitor the physical and mental health of people during their daily life. In particular, negative mental states like depression and anxiety are big problems in modern societies, usually due to stressful situations during everyday activities including work. This paper presents a machine learning approach for stress detection on people using wearable physiological sensors with the final aim of improving their quality of life. The presented technique can monitor the state of the subject continuously and classify it into "stressful" or "non-stressful" situations. Our classification results show that this method is a good starting point towards real-time stress detection.

IEEE Access
Affective human-robot interaction is still an active area of research in part due to the great ad... more Affective human-robot interaction is still an active area of research in part due to the great advances in artificial intelligence. Now, the design of autonomous devices that work in real therapeutic environments has become a plausible reality. Affective human-robot interaction requires a robot to analyze the emotional state of the human interlocutor and interpret emotional responses that can be used, not merely in the interaction but, for example, to provoke desired therapeutic responses. It is, therefore, necessary to broaden experimental techniques into more realistic paradigms, where the capacity of emotion estimation can be completely explored. This exploratory paper proposes a realistic experimental paradigm in which the robot employs a dramatic story to evoke emotions in the users, and tests previously self-designed methodologies to be able to make estimates of the users' emotional state in real-time. Regardless of the multiple impediments and restrictions, and all the aspects that could still be improved, this paper can outline the feasibility of the proposed methodology in realistic scenarios.
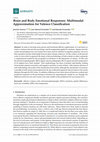
Sensors
In order to develop more precise and functional affective applications, it is necessary to achiev... more In order to develop more precise and functional affective applications, it is necessary to achieve a balance between the psychology and the engineering applied to emotions. Signals from the central and peripheral nervous systems have been used for emotion recognition purposes, however, their operation and the relationship between them remains unknown. In this context, in the present work, we have tried to approach the study of the psychobiology of both systems in order to generate a computational model for the recognition of emotions in the dimension of valence. To this end, the electroencephalography (EEG) signal, electrocardiography (ECG) signal and skin temperature of 24 subjects have been studied. Each methodology has been evaluated individually, finding characteristic patterns of positive and negative emotions in each of them. After feature selection of each methodology, the results of the classification showed that, although the classification of emotions is possible at both c...

Frontiers in neuroinformatics, 2018
The anticipatory recognition of braking is essential to prevent traffic accidents. For instance, ... more The anticipatory recognition of braking is essential to prevent traffic accidents. For instance, driving assistance systems can be useful to properly respond to emergency braking situations. Moreover, the response time to emergency braking situations can be affected and even increased by different driver's cognitive states caused by stress, fatigue, and extra workload. This work investigates the detection of emergency braking from driver's electroencephalographic (EEG) signals that precede the brake pedal actuation. Bioelectrical signals were recorded while participants were driving in a car simulator while avoiding potential collisions by performing emergency braking. In addition, participants were subjected to stress, workload, and fatigue. EEG signals were classified using support vector machines (SVM) and convolutional neural networks (CNN) in order to discriminate between braking intention and normal driving. Results showed significant recognition of emergency braking i...
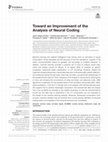
Frontiers in neuroinformatics, 2017
Machine learning and artificial intelligence have strong roots on principles of neural computatio... more Machine learning and artificial intelligence have strong roots on principles of neural computation. Some examples are the structure of the first perceptron, inspired in the retina, neuroprosthetics based on ganglion cell recordings or Hopfield networks. In addition, machine learning provides a powerful set of tools to analyze neural data, which has already proved its efficacy in so distant fields of research as speech recognition, behavioral states classification, or LFP recordings. However, despite the huge technological advances in neural data reduction of dimensionality, pattern selection, and clustering during the last years, there has not been a proportional development of the analytical tools used for Time-Frequency (T-F) analysis in neuroscience. Bearing this in mind, we introduce the convenience of using non-linear, non-stationary tools, EMD algorithms in particular, for the transformation of the oscillatory neural data (EEG, EMG, spike oscillations…) into the T-F domain pri...
Lecture Notes in Computer Science, 2007
An architecture for a cortical stimulator with visual neuroprosthetic purposes is presented. This... more An architecture for a cortical stimulator with visual neuroprosthetic purposes is presented. This device uses a 3D penetrating multielectrode array, which will be implanted in V1, offering different signal amplitude sets with the programable current source module. This electrode array has been proved for injecting current (charge) in a safety, secure and precise way during animal acute experimentation. The dynamic
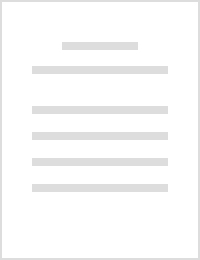
Lecture Notes in Computer Science, 2007
The issue of classification has long been a central topic in the analysis of multielectrode data,... more The issue of classification has long been a central topic in the analysis of multielectrode data, either for spike sorting or for getting insight into interactions among ensembles of neurons. Related to coding, many multivariate statistical techniques such as linear discriminant analysis (LDA) or artificial neural networks (ANN) have been used for dealing with the classification problem providing very similar performances. This is, there is no method that stands out from others and the right decision about which one to use is mainly depending on the particular cases demands. In this paper, we found groups of rabbit ganglion cells with distinguishable coding performances by means of a simple based on behaviour method. The method consisted of creating population subsets based on the autocorrelograms of the cells and grouping them according to a minimal Euclidian distance. These subpopulations shared functional properties and may be used for functional identification of the subgroups. Information theory (IT) has been used to quantify the coding capability of every subpopulation. It has been described that all cells that belonged to a certain subpopulation showed very small variances in the information they conveyed while these values were significantly different across subpopulations, suggesting that the functional separation worked around the capacity of each cell to code different stimuli. In addition, the overall informational ability of each of the generated subpopulations kept similar. This trend was present for an increasing number of classes until a critical value was reached, proposing a natural value for functional classes.
Lecture Notes in Computer Science, 1999
In this paper the coding capabilities of individual retinal ganglion cells are compared with resp... more In this paper the coding capabilities of individual retinal ganglion cells are compared with respect to the coding capabilities of small population of cells using different neural networks. This approach allows not only the identification of the most discriminating cells, but also detection of the parameters that are more important for the discrimination task. Our results show that the spike rate together with the exact timing of the first spike at light-ON were the most important parameters for encoding stimulus features. Furthermore we found that whereas single ganglion cells are poor classifiers of visual stimuli, a population of only 15 cells can distinguish stimulus color and intensity reasonable well. This demonstrates that visual information is coded as the overall set of activity levels across neurons rather than by single cells.
Uploads
Papers by Jose M. Ferrandez