Papers by Delmiro Fernandez-Reyes
Scientific Reports, May 11, 2022
arXiv (Cornell University), Nov 21, 2013
arXiv (Cornell University), Dec 20, 2013
Optics Express, Nov 9, 2020
medRxiv (Cold Spring Harbor Laboratory), May 26, 2021
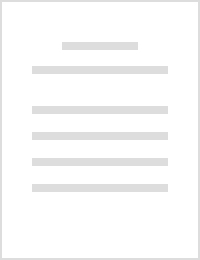
International Conference on Learning Representations, Dec 23, 2013
ABSTRACT Recent non-linear feature selection approaches employing greedy optimisation of Centred ... more ABSTRACT Recent non-linear feature selection approaches employing greedy optimisation of Centred Kernel Target Alignment(KTA) exhibit strong results in terms of generalisation accuracy and sparsity. However, they are computationally prohibitive for large datasets. We propose randSel, a randomised feature selection algorithm, with attractive scaling properties. Our theoretical analysis of randSel provides strong probabilistic guarantees for correct identification of relevant features. RandSel's characteristics make it an ideal candidate for identifying informative learned representations. We've conducted experimentation to establish the performance of this approach, and present encouraging results, including a 3rd position result in the recent ICML black box learning challenge as well as competitive results for signal peptide prediction, an important problem in bioinformatics.
arXiv (Cornell University), Aug 13, 2020
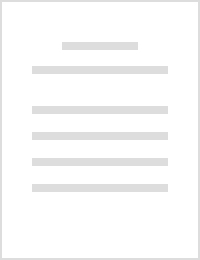
ABSTRACT Commencing with the Fisher discriminant, the Otsu algorithm for image segmentation is re... more ABSTRACT Commencing with the Fisher discriminant, the Otsu algorithm for image segmentation is reviewed and a multidimensional extension proposed. Identification of the pixels belonging to red-blood cells in 1300times1030 24-bit colour images of thin-film microscope slides of laboratory samples of malarial infected blood is used to illustrate the Otsu algorithm and the proposed extension. The Otsu algorithm is applied to the image intensity and independently to each of the colour channels in a set of 142 images and the best combination of the colour channel outputs that maximizes the Fisher discriminant selected. ROC and MRROC curves and error rates are used to evaluate performance. The multidimensional extension of the Otsu algorithm is similarly assessed. Preliminary results obtained from sequential application of the multi-dimensional extension of the Otsu algorithm are presented and directions for further research discussed
Human Molecular Genetics, Aug 1, 1997
Medical Image Analysis, Jul 1, 2023
Lecture Notes in Computer Science, 2019
npj digital medicine, Nov 4, 2022
Transfusion Clinique Et Biologique, Feb 1, 1999

Frontiers in Public Health
Malaria is a common and serious disease that primarily affects developing countries and its sprea... more Malaria is a common and serious disease that primarily affects developing countries and its spread is influenced by a variety of environmental and human behavioral factors; therefore, accurate prevalence prediction has been identified as a critical component of the Global Technical Strategy for Malaria from 2016 to 2030. While traditional differential equation models can perform basic forecasting, supervised machine learning algorithms provide more accurate predictions, as demonstrated by a recent study using an elastic net model (REMPS). Nevertheless, current short-term prediction systems do not achieve the required accuracy levels for routine clinical practice. To improve in this direction, stacked hybrid models have been proposed, in which the outputs of several machine learning models are aggregated by using a meta-learner predictive model. In this paper, we propose an alternative specialist hybrid approach that combines a linear predictive model that specializes in the linear c...
<p>A) HAT dataset (wKIERA settings: <i>poolsize</i> = 10, <i>maxiter</... more <p>A) HAT dataset (wKIERA settings: <i>poolsize</i> = 10, <i>maxiter</i> = 400, <i>rep</i> = 2000, wkRBF ρ = 0.01); B) TB dataset (<i>poolsize</i> = 10, <i>maxiter</i> = 400, <i>rep</i> = 2000, wkRBF ρ = 1). C) MALARIA dataset (<i>poolsize</i> = 10, <i>maxiter</i> = 400, <i>rep</i> = 2000, wkRBF ρ = 1). <u>Top</u>, <u>Middle</u> and <u>Bottom</u>: See legend on <a href="http://www.plosone.org/article/info:doi/10.1371/journal.pone.0001806#pone-0001806-g002" target="_blank">Figure 2</a>.</p
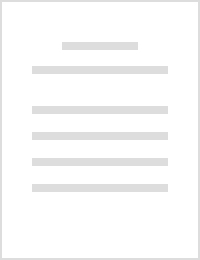
<p>Proteins with differential profiles between the malaria groups were classified into phys... more <p>Proteins with differential profiles between the malaria groups were classified into physiological pathways. This included proteins identified common to all malaria groups (blue bars), common to both defined severe malaria syndromes (purple bars), proteins with levels elevated in SMA (light green bars), proteins with levels elevated in CM (light orange bars) and proteins specific to CM (dark orange bars). For each panel, columns were stacked by number of proteins identified in each category. Grey dotted connectors represent either a regulation link or a common protein component between to physiological pathways. UM: uncomplicated malaria; SMA: severe malaria anemia; CM: cerebral malaria. Proteins are represented by their gene names (Refer to <a href="http://www.plospathogens.org/article/info:doi/10.1371/journal.ppat.1004038#ppat-1004038-t002" target="_blank">Table 2</a>, Fig. S3 and Table S2 in <a href="http://www.plospathogens.org/article/info:doi/10.1371/journal.ppat.1004038#ppat.1004038.s001" target="_blank">Text S1</a> for full names).</p
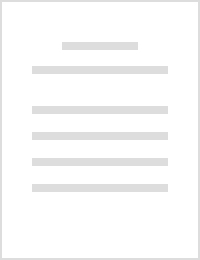
<p>(<b>A</b>) Applying a non-parametric test, 29 human proteins were identified... more <p>(<b>A</b>) Applying a non-parametric test, 29 human proteins were identified showing significant (adjusted p<0.001) differences between any of the four groups. Cluster analysis using self-organizing tree algorithm (SOTA) revealed four different clusters with distinct protein profiles designated as ‘malaria decreased’, ‘malaria increased’, ‘severe malaria’ and ‘cerebral malaria’ (see also Fig. S3 in <a href="http://www.plospathogens.org/article/info:doi/10.1371/journal.ppat.1004038#ppat.1004038.s001" target="_blank">Text S1</a>). (<b>B</b>) Heatmap visualizing protein profiles in individual patients. Samples were organized according to group affiliation and proteins were sorted following SOTA clusters. Displayed are scaled relative intensities of each protein in each group (CC = grey, UM = green, SMA = red, CM = blue).</p
Uploads
Papers by Delmiro Fernandez-Reyes