Thesis Chapters by Juan María Herrera
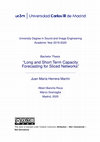
It has been widely established that the future 5G networks will make use of the network slicing c... more It has been widely established that the future 5G networks will make use of the network slicing concept, but this dynamic management of resources proves to be both a critical and a challenging mechanism to accommodate future time-varying service demands. The introduction of this thesis focuses on the recent use of artificial intelligence for networks and systems and overviews the changes it has brought to traditional capacity forecasting algorithms.
As a first approach, this thesis takes an existing short term capacity forecasting deep-learning system, DeepCog, and examines it thoroughly in order to determine optimal deterministic and design parameters and functions. This is done by first minimizing the validation loss, and then the custom cost metrics the original system was evaluated on. After this first section, an optimized system is proposed: DeepCogV2, capable of performing more accurate predictions with minimized cost and convergence time. The second part of this thesis is focused on building upon this short term capacity forecasting prediction system in order to design a long-term capacity forecasting system, capable of predicting network usage patterns for intervals longer than one hour. Two different approaches are taken in order to do so, with their respective strengths and limitations clearly discussed. After this section, a long-term capacity forecasting system (based on the previously proposed architecture of DeepCogV2) is proposed.
Uploads
Thesis Chapters by Juan María Herrera
As a first approach, this thesis takes an existing short term capacity forecasting deep-learning system, DeepCog, and examines it thoroughly in order to determine optimal deterministic and design parameters and functions. This is done by first minimizing the validation loss, and then the custom cost metrics the original system was evaluated on. After this first section, an optimized system is proposed: DeepCogV2, capable of performing more accurate predictions with minimized cost and convergence time. The second part of this thesis is focused on building upon this short term capacity forecasting prediction system in order to design a long-term capacity forecasting system, capable of predicting network usage patterns for intervals longer than one hour. Two different approaches are taken in order to do so, with their respective strengths and limitations clearly discussed. After this section, a long-term capacity forecasting system (based on the previously proposed architecture of DeepCogV2) is proposed.
As a first approach, this thesis takes an existing short term capacity forecasting deep-learning system, DeepCog, and examines it thoroughly in order to determine optimal deterministic and design parameters and functions. This is done by first minimizing the validation loss, and then the custom cost metrics the original system was evaluated on. After this first section, an optimized system is proposed: DeepCogV2, capable of performing more accurate predictions with minimized cost and convergence time. The second part of this thesis is focused on building upon this short term capacity forecasting prediction system in order to design a long-term capacity forecasting system, capable of predicting network usage patterns for intervals longer than one hour. Two different approaches are taken in order to do so, with their respective strengths and limitations clearly discussed. After this section, a long-term capacity forecasting system (based on the previously proposed architecture of DeepCogV2) is proposed.