Papers by Lyudmila S Mihaylova
Harish Bhaskar graduated from University of Madras, India with a Bachelors degree in Information ... more Harish Bhaskar graduated from University of Madras, India with a Bachelors degree in Information Technology. Harish then joined University of Exeter in the year 2002 for a Master of Science degree in Autonomous Systems. During this degree he specialized in different ...

Atmosphere
Recently, analyses of the air quality in Pakistan have received significant interest, especially ... more Recently, analyses of the air quality in Pakistan have received significant interest, especially regarding the impact of air pollutant concentrations on human health. The Atlas of Baseline Environmental Profiling along the China-Pakistan Economic Corridor (CPEC) at five locations in Gilgit-Baltistan (GB) is a major landmark in this regard due to the presence of massive glaciers in the region, which are considered as water reserves for the country. Using various statistical measurements, the air quality was analyzed at the studied geographic locations. Further, air quality was evaluated based on air pollutant data acquired from ambient air monitoring laboratories. For example, 24 h concentrations of particulate matter (PM2.5) were found to range from 25.4 to 60.1 µg/m3, with peaks in the winter season at Gilgit. It was found that PM2.5 values were 1.7 and 1.3 times greater than National Environmental Quality Standards (NEQS) standards only at Gilgit and Chilas, respectively, and 1.5 ...

2020 42nd Annual International Conference of the IEEE Engineering in Medicine & Biology Society (EMBC), 2020
A large amount of calibration data is typically needed to train an electroencephalogram (EEG)-bas... more A large amount of calibration data is typically needed to train an electroencephalogram (EEG)-based braincomputer interfaces (BCI) due to the non-stationary nature of EEG data. This paper proposes a novel weighted transfer learning algorithm using a dynamic time warping (DTW) based alignment method to alleviate this need by using data from other subjects. DTW-based alignment is first applied to reduce the temporal variations between a specific subject data and the transfer learning data from other subjects. Next, similarity is measured using Kullback Leibler divergence (KL) between the DTW aligned data and the specific subject data. The other subjects' data are then weighted based on their KL similarity to each trials of the specific subject data. This weighted data from other subjects are then used to train the BCI model of the specific subject. An experiment was performed on publicly available BCI Competition IV dataset 2a. The proposed algorithm yielded an average improvement of 9% compared to a subject-specific BCI model trained with 4 trials, and the results yielded an average improvement of 10% compared to naive transfer learning. Overall, the proposed DTW-aligned KL weighted transfer learning algorithm show promise to alleviate the need of large amount of calibration data by using only a short calibration data.
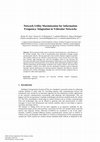
Proceedings of 2nd International Multi-Disciplinary Conference Theme: Integrated Sciences and Technologies, IMDC-IST 2021, 7-9 September 2021, Sakarya, Turkey, 2022
The increment in traffic jams in vehicular environments has a vital influence on the wireless cha... more The increment in traffic jams in vehicular environments has a vital influence on the wireless channel. This is due to broadcasting many application messages among communicated vehicles. This has generated a data wireless channel congestion problem. This problem influences the efficiency and performance of the network due to the large data collision, high messages loss, and low throughput which in turn decreases the awareness and safety in the vehicular environments. In this paper, a new efficient broadcast approach has been developed based on the network utility maximization method to adjust vehicle broadcasting rate and mitigate overhead on the wireless channel. The results from the simulation scenario have shown that the developed broadcast protocol enhances vehicular network performance by reducing channel congestion and improves network attributes such as delay, throughput, messages loss, and total channel occupation time as compared to other tested approaches.

Heliyon, 2021
Control systems need to be able to operate under uncertainty and especially under attacks. To add... more Control systems need to be able to operate under uncertainty and especially under attacks. To address such challenges, this paper formulates the solution of robust control for uncertain systems under time-varying and unknown time-delay attacks in cyber-physical systems (CPSs). A novel control method able to deal with thwart time-delay attacks on closed-loop control systems is proposed. Using a descriptor model and an appropriate Lyapunov functional, sufficient conditions for closed-loop stability are derived based on linear matrix inequalities (LMIs). A design procedure is proposed to obtain an optimal state feedback control gain such that the uncertain system can be resistant under an injection time-delay attack with variable delay. Furthermore, various fault detection frameworks are proposed by following the dynamics of the measured data at the system's input and output using statistical analysis such as correlation analysis and K-L (Kullback-Leibler) divergence criteria to detect attack's existence and to prevent possible instability. Finally, an example is provided to evaluate the proposed design method's effectiveness.
2020 IEEE 23rd International Conference on Information Fusion (FUSION), 2020
This is a repository copy of Machine learning approaches for cancer bone segmentation from micro ... more This is a repository copy of Machine learning approaches for cancer bone segmentation from micro computed tomography images.

2020 13th International Conference on Developments in eSystems Engineering (DeSE), 2020
This paper presents a non-random weight initialisation scheme for convolutional neural network la... more This paper presents a non-random weight initialisation scheme for convolutional neural network layers. It builds upon previous work that was limited to perceptron layers, but in that work repeatable determinism was achieved with equality in categorisation accuracy between the established random scheme and a linear ramp non-random scheme. This work however, is in Convolutional layers and are the layers that have been responsible for better than human performance in image recognition. The previous perceptron work found that number range was more important rather than the gradient. However, that was due to the fully connected nature of dense layers. Although, in convolutional layers by contrast, there is an order direction implied, and the weights relate to filters rather than image pixel positions, so the weight initialisation is more complex. However, the paper demonstrates a better performance, over the currently established random schemes with convolutional layers. The proposed met...
2016 19th International Conference on Information Fusion (FUSION), 2016
An efficient recognition framework requires both good feature representation and effective classi... more An efficient recognition framework requires both good feature representation and effective classification methods. This paper proposes such a framework based on a spatial Scale Invariant Feature Transform (SIFT) combined with a logistic regression classifier. The performance of the proposed framework is compared to that of state-of-the-art methods based on the Histogram of Orientation Gradients, SIFT features, Support Vector Machine and K-Nearest Neighbours classifiers. By testing with the largest vehicle logo data-set, it is shown that the proposed framework can achieve a classification accuracy of 99:93%, the best among all studied methods. Moreover, the proposed framework shows robustness when noise is added in both training and testing images.

IET Wireless Sensor Systems, 2021
Communications between the Internet of Vehicles in smart cities helps increase the awareness and ... more Communications between the Internet of Vehicles in smart cities helps increase the awareness and safety among drivers. However, the channel congestion problem is considered as a key challenge for the communication networks due to continuing collection and exchange of traffic information in dense environments. The channel congestion problem degrades the efficiency and reliability of the ad hoc network. Therefore, the adaptation of the data rate and power control is considered as one of the effective solutions to mitigate channel congestion. A new hybrid game transmission rate and power channel congestion control approach on the Internet of Vehicle networks where the nodes play as greedy opponents demanding high information rates with the maximum power level are developed. Furthermore, the existence of a Nash equilibrium, which is the optimal information rate and power transmission for every vehicle, is established. Simulation results demonstrate that the proposed approach enhances the network performance by an overall percentage of 42.27%, 43.94% and 47.66% regarding channel busy time, messages loss and data collision as compared with others. This increases the awareness and performance of the vehicular communication network. This is an open access article under the terms of the Creative Commons Attribution License, which permits use, distribution and reproduction in any medium, provided the original work is properly cited.

Frontiers in Bioengineering and Biotechnology, 2021
Most mental disorders, such as addictive diseases or schizophrenia, are characterized by impaired... more Most mental disorders, such as addictive diseases or schizophrenia, are characterized by impaired cognitive function and behavior control originating from disturbances within prefrontal neural networks. Their often chronic reoccurring nature and the lack of efficient therapies necessitate the development of new treatment strategies. Brain-computer interfaces, equipped with multiple sensing and stimulation abilities, offer a new toolbox whose suitability for diagnosis and therapy of mental disorders has not yet been explored. This study, therefore, aimed to develop a biocompatible and multimodal neuroprosthesis to measure and modulate prefrontal neurophysiological features of neuropsychiatric symptoms. We used a 3D-printing technology to rapidly prototype customized bioelectronic implants through robot-controlled deposition of soft silicones and a conductive platinum ink. We implanted the device epidurally above the medial prefrontal cortex of rats and obtained auditory event-related...
2019 10th International Conference on Dependable Systems, Services and Technologies (DESSERT), 2019
This is a repository copy of Non-random weight initialisation in deep learning networks for repea... more This is a repository copy of Non-random weight initialisation in deep learning networks for repeatable determinism.
Towards Autonomous Robotic Systems, 2021
Visual odometry can be used to estimate the pose of a robot from current and recent video frames.... more Visual odometry can be used to estimate the pose of a robot from current and recent video frames. A problem with these methods is that they drift over time due to the accumulation of estimation errors at each time-step. In this short paper we propose and briefly demonstrate the potential benefit of using prior 2D, top-down map information combined with multiple hypothesis particle filtering to correct visual odometry estimates. The results demonstrate a substantial improvement in robustness and accuracy over the sole use of visual odometry.

Journal of Neural Engineering, 2020
Objective. Common spatial patterns (CSP) is a prominent feature extraction algorithm in motor ima... more Objective. Common spatial patterns (CSP) is a prominent feature extraction algorithm in motor imagery (MI)-based brain-computer interfaces (BCIs). However, CSP is computed using sample-based covariance-matrix estimation. Hence, its performance deteriorates if the number of training trials is small. To address this problem, this paper proposes a novel regularized covariance matrix estimation framework for CSP (i.e. DTW-RCSP) based on dynamic time warping (DTW) and transfer learning.Approach. The proposed framework combines the subject-specific covariance matrix (Σ ss) estimated using the few available trials from the new subject, with a novel DTW-based transferred covariance matrix (Σ DTW) estimated using previous subjects' trials. In the proposed Σ DTW , the available labelled trials from the previous subjects are temporally aligned to the average of the few available trials of the new subject from the same class using DTW. This alignment aims to reduce temporal variations and non-stationarities between previous subjects trials and the available few trials from the new subjects. Moreover, to tackle the problem of regularization parameter selection when only few trials are available for training, an online method is proposed, where the best regularization parameter is selected based on the confidence scores of the trained classifier on upcoming first few labelled testing trials. Main results. The proposed framework is evaluated on two datasets against two baseline algorithms. The obtained results reveal that DTW-RCSP significantly outperformed the baseline algorithms at various testing scenarios, particularly, when only a few trials are available for training. Significance. Impressively, our results show that successful BCI interactions could be achieved with a calibration session as small as only one trial per class.

Journal of Reliable Intelligent Environments, 2020
Modern Smart City applications draw on the need for requirements that are safe, reliable and sust... more Modern Smart City applications draw on the need for requirements that are safe, reliable and sustainable, as such these applications have a need to utilise machine learning mechanisms such that they are consistent with public liability. Machine and Deep Learning networks therefore are required to be in a form that are safe and deterministic in their development and also in their deployment. The viability of non-random weight initialisation schemes in neural networks make the network more deterministic in learning sessions which is a desirable property in safety critical systems where deep learning is applied to smart city applications and where public liability is a concern. The paper uses a variety of schemes over number ranges and gradients and achieved a 98.09% accuracy figure, +0.126% higher than the original random number scheme at 97.964%. The paper highlights that in this case it is the number range and not the gradient that is affecting the achieved accuracy most dominantly, although there can be a coupling of number range with activation functions used. Unexpectedly in this paper, an effect of numerical instability was discovered from run to run when run on a multi-core CPU. The paper also has shown the enforcement of consistent deterministic results on an multicore CPU by defining atomic critical code regions, and that aids repeatable Information Assurance (IA) in model fitting (or learning sessions). That enforcement of consistent repeatable determinism has also a benefit to accuracy even for the random schemes, and a highest score of 98.29%, +0.326% higher than the baseline was achieved. However, also the non-random initialisation scheme causes weight arrangements after learning to be more structured which has benefits for validation in safety critical applications.

IOP Conference Series: Materials Science and Engineering, 2019
The advancement of threats is focusing commanders to consider how to combat these complex threats... more The advancement of threats is focusing commanders to consider how to combat these complex threats throughout the kill chain including prior to launch and Anti-Access Area Denial (A2AD). To match this technology race, modern platforms have been designed with integrated command and control systems and automated Defensive Aids Suites built on modular open system architectures incorporating less diverse but more complex software driven systems. The successful operation of these combat systems is reliant upon the availability of accurate, configured, harmonised and "time sensitive" mission data without which the systems may be ineffective. This paper explores the use of threat analysis diagramming techniques, and open architectures tightly integrated with a EWOS life-cycle; to develop countermeasures with a measured response beyond the traditional self-protection kill chain stages of self-protection. It introduces how threat analysis prepares understanding for simulation and how a countermeasure description language can be used to store and exchange countermeasures in a structured form. This level of intelligence data support and analysis coordinated and synchronised across multiple platforms thereby facilitating the complexities of force protection higher up the kill chain into an onion of protection mapped to a Venn diagram of countermeasure types (design intentions) with differing data needs.
IEEE Sensors Journal, 2017
This paper presents a shrinkage-based particle filter method for tracking a mobile user in wirele... more This paper presents a shrinkage-based particle filter method for tracking a mobile user in wireless networks. The proposed method estimates the shadowing noise covariance matrix using the shrinkage technique. The particle filter is designed with the estimated covariance matrix to improve the tracking performance. The shrinkage-based particle filter can be applied in a number of applications for navigation, tracking, and localization when the available sensor measurements are correlated and sparse. The performance of the shrinkage-based particle filter is compared with the posterior Cramer-Rao lower bound, which is also derived in this paper. The advantages of the proposed shrinkage-based particle filter approach are demonstrated via simulation and experimental results.
2021 IEEE 24th International Conference on Information Fusion (FUSION), 2021
This is a repository copy of Real-time activation pattern monitoring and uncertainty characterisa... more This is a repository copy of Real-time activation pattern monitoring and uncertainty characterisation in image classification.
arXiv (Cornell University), Nov 10, 2021
Learning in uncertain, noisy, or adversarial environments is a challenging task for deep neural n... more Learning in uncertain, noisy, or adversarial environments is a challenging task for deep neural networks (DNNs). We propose a new theoretically grounded and efficient approach for robust learning that builds upon Bayesian estimation and Variational Inference. We formulate the problem of density propagation through layers of a DNN and solve it using an Ensemble Density Propagation (EnDP) scheme. The EnDP approach allows us to propagate moments of the variational probability distribution across the layers of a Bayesian DNN, enabling the estimation of the mean and covariance of the predictive distribution at the output of the model. Our experiments using MNIST and CIFAR-10 datasets show a significant improvement in the robustness of the trained models to random noise and adversarial attacks.

arXiv (Cornell University), Nov 2, 2016
Semi-supervised and unsupervised systems provide operators with invaluable support and can tremen... more Semi-supervised and unsupervised systems provide operators with invaluable support and can tremendously reduce the operators' load. In the light of the necessity to process large volumes of video data and provide autonomous decisions, this work proposes new learning algorithms for activity analysis in video. The activities and behaviours are described by a dynamic topic model. Two novel learning algorithms based on the expectation maximisation approach and variational Bayes inference are proposed. Theoretical derivations of the posterior estimates of model parameters are given. The designed learning algorithms are compared with the Gibbs sampling inference scheme introduced earlier in the literature. A detailed comparison of the learning algorithms is presented on real video data. We also propose an anomaly localisation procedure, elegantly embedded in the topic modeling framework. It is shown that the developed learning algorithms can achieve 95% success rate. The proposed framework can be applied to a number of areas, including transportation systems, security and surveillance.
Uploads
Papers by Lyudmila S Mihaylova