Papers by Mostafa H Tawfeek
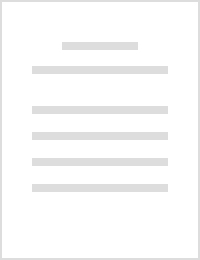
Transportation Research Part C: Emerging Technologies
Abstract This study proposes an addition to the architecture of the context-aware Driver Assistan... more Abstract This study proposes an addition to the architecture of the context-aware Driver Assistance Systems (DASs) by introducing a context identification layer to the reasoning subsystem. The proposed layer contains two algorithms that work in sequence: the infrastructure detection algorithm and the driver classification algorithm, respectively. The infrastructure detection algorithm aims to identify intersection-related driving when the driver adjusts his or her behavior due to the presence of an intersection ahead. Then, the driver classification algorithm categorizes drivers into cautious, normal, and aggressive at both locations. Data from 64 drivers in a Naturalistic Driving Study was used to prove the concept of the proposed layer. Several behavioral measures were extracted, including following distance, relative speed, headway, acceleration, time-to-collision, and jerk. These behavioral measures were then used to train the algorithms in the context identification layer. The results of both algorithms supported the concept of the proposed layer. These results have implications related to driver behaviors including i) the intersection-related driving behavior can be detected, ii) the drivers tend to be relatively aggressive at intersections when compared to segments, and iii) the driver classification, which ignores the driver’s relative location to intersections, were more likely to misclassify drivers as aggressive when they were in high intersection density areas such as downtown cores. The findings of this study emphasized the importance of context-aware DAS architecture that acknowledges and integrates the variation in driver behavior due to both a change in the surrounding environment and drivers’ individual needs.
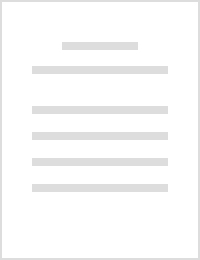
Journal of Transportation Safety & Security
This study aims at modelling unassisted drivers’ speed at the yellow onset to enhance Connected a... more This study aims at modelling unassisted drivers’ speed at the yellow onset to enhance Connected and Autonomous Vehicles applications at signalised intersections and maximise drivers’ comfort. For this purpose, a total of 2442 real-life vehicle trajectories were analysed to extract driver behavioural measures (i.e. speed, acceleration, and distance to intersection) at different times before the yellow onset. These behavioural measures were used to integrate drivers’ perceptual ability into modelling drivers’ speed at the yellow onset. To develop these models, three machine learning techniques; namely, linear regression, Support Vector Machine, and Neural Networks have been adopted. The best model was a neural network model and was selected based on the goodness-of-fit of the test dataset which has an R-squared value of 0.97. The results indicate that the speed at the yellow onset can be estimated based on behavioural measures while accounting for drivers’ perceptual ability. Also, the model can contribute to a V2I application by assisting the driver in a partially autonomous vehicle to avoid trapping in the dilemma zone and stop safely at signalised intersections. Also, the model can be used to recommend a comfortable riding speed, from a rider’s perspective to a fully autonomous vehicle.

Canadian Journal of Civil Engineering
Rear-end collisions represent a quarter to one-third of the total number of collisions occurring ... more Rear-end collisions represent a quarter to one-third of the total number of collisions occurring on North American roads. While there are several methods to mitigate rear-end collision effects, one way is to warn drivers about impending events using forward collision warning (FCW) systems. At the core of any FCW algorithm is a trigger distance at which a message is relayed to the driver to avoid rear-end collisions. The main goal of this paper is to propose a warning distance model based on naturalistic driver following behavior. This was achieved by investigating car-following events within a critical time-to-collision range. A total of 5785 candidate car-following events were identified for the model development from 2 months of naturalistic driving study data of 63 drivers. Using regression analysis, the minimum warning distance was linked to several performance measures. It was found that the relative speed, the host vehicle speed, and the host vehicle acceleration can significa...

Canadian Journal of Civil Engineering
This study investigates the car-following behavior during braking at intersections and segments. ... more This study investigates the car-following behavior during braking at intersections and segments. Car-following events were extracted from a naturalistic driving dataset, mapped using ArcGIS, and analyzed to differentiate between the intersection- and segment-related events. The intersection-related events were identified according to an intersection influence area, which was estimated based on the stopping sight distance and the speed limit. Five behavioral measures were quantified based on exploring the Probability Density Functions (PDF) for intersection- and segment-related events. The results showed that there were significant differences between the PDFs of the measures for both cases. Moreover, it was indicated that drivers tend to be more aggressive at intersections when compared to segments. Thus, it is crucial to consider the driver’s location when investigating driver behavior. The quantified behavioral measures are a rich data source that can be used for car-following mic...
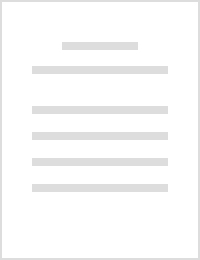
SIMULATION
Rear-end collisions represent a quarter to one-third of the total number of collisions occurring ... more Rear-end collisions represent a quarter to one-third of the total number of collisions occurring on North American roads. Consequently, Forward Collision Warning (FCW) algorithms have been developed to mitigate this type of critical collision by warning drivers about an impending rear-end event. The algorithms are typically tested to ensure their effectiveness in reducing specific events, such as rear-end conflicts and/or collisions, or by assessing the change in the frequency and severity of braking maneuvers. Such assessments are usually microscopic in nature and deal with isolated (independent) situations. This paper aims at assessing six FCW algorithms at a network level with varying market penetration rates using a calibrated micro-simulation model. The algorithms were assessed in terms of their safety (rear-end conflicts frequency), mobility (travel times), and environmental impacts (emissions and fuel consumption). Based on the results of this study, most of the FCW algorithm...
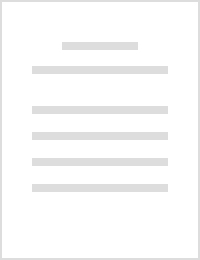
Transportation Research Record: Journal of the Transportation Research Board
Safety Performance Functions (SPFs) are regression models used to predict the expected number of ... more Safety Performance Functions (SPFs) are regression models used to predict the expected number of collisions as a function of various traffic and geometric characteristics. One of the integral components in developing SPFs is the availability of accurate exposure factors, that is, annual average daily traffic (AADT). However, AADTs are not often available for minor roads at rural intersections. This study aims to develop a robust AADT estimation model using a deep neural network. A total of 1,350 rural four-legged, stop-controlled intersections from the Province of Alberta, Canada, were used to train the neural network. The results of the deep neural network model were compared with the traditional estimation method, which uses linear regression. The results indicated that the deep neural network model improved the estimation of minor roads’ AADT by 35% when compared with the traditional method. Furthermore, SPFs developed using linear regression resulted in models with statistically...
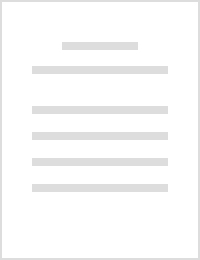
Canadian Journal of Civil Engineering, 2021
This study aims at modelling drivers’ speed in car-following during braking situations at interse... more This study aims at modelling drivers’ speed in car-following during braking situations at intersections to estimate a safe comfortable human-like speed at the minimum car-following distance for Autonomous Vehicles (AV). Several car-following behavioral measures at different times before reaching the minimum following distance and the intersection control type (signalized or unsignalized) were extracted to train the model using three machine learning techniques. The results showed that the XGBoost model is superior when compared to other techniques with R-squared values of 0.99 and 0.97 for training and testing datasets. The results also indicated that the control type impact driver speed at the minimum following distance. The modelled speed will provide more comfortable speed to the AV riders and will not violate the expectations of the surrounding traditional vehicle drivers. Also, the proposed model can be adopted to enhance current car-following models by considering the effect o...
Canadian Journal of Civil Engineering, 2021
This study proposes a framework that accounts for mental workload and available sight distances t... more This study proposes a framework that accounts for mental workload and available sight distances to estimate advisory speed limits on horizontal curves. To achieve this goal, automated scripts were ...
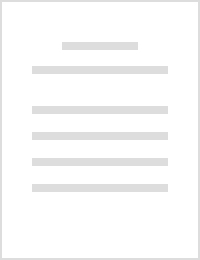
Accident; analysis and prevention, 2018
An important element of highway design is ensuring that the available sight distance (ASD) on a h... more An important element of highway design is ensuring that the available sight distance (ASD) on a highway meets driver needs. For instance, if the ASD at any point on a highway is less than the distance required to come to a complete stop after seeing a hazard (i.e. Stopping Sight Distance (SSD)), the driver will not be able to stop in time to avoid a collision. SSD is function of a number of variables which vary depending on the driver, the vehicle driven and surface conditions; examples of such variables include a driver's perception reaction time or PRT (i.e. the time required by the driver to perceive and react to a hazard) and the deceleration rate of the vehicle. Most design guides recommend deterministic values for PRT and deceleration rates. Although these values may serve the needs of the average driver, they may not satisfy the needs of drivers with limited abilities. In other words, even if the ASD exceeds required SSD defined in the design guide, it might not always sa...
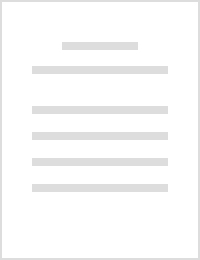
Canadian Journal of Civil Engineering, 2018
Rear-end collisions represent a quarter to one-third of the total number of collisions occurring ... more Rear-end collisions represent a quarter to one-third of the total number of collisions occurring on North American roads. While there are several methods to mitigate rear-end collision effects, one way is to warn drivers about impending events using forward collision warning (FCW) systems. At the core of any FCW algorithm is a trigger distance at which a message is relayed to the driver to avoid rear-end collisions. The main goal of this paper is to propose a warning distance model based on naturalistic driver following behavior. This was achieved by investigating car-following events within a critical time-to-collision range. A total of 5785 candidate car-following events were identified for the model development from 2 months of naturalistic driving study data of 63 drivers. Using regression analysis, the minimum warning distance was linked to several performance measures. It was found that the relative speed, the host vehicle speed, and the host vehicle acceleration can significantly affect the minimum warning distance. To assess the performance of the developed algorithm, it was compared to six of the existing FCW algorithms in terms of warning distances. The results of the developed algorithm were consistent with the other perceptual FCW algorithms. However, the warning distances of the proposed algorithm were less than the distances produced by the kinematic algorithms. The proposed algorithm could be used as a minimum threshold to trigger an alert for an FCW algorithm. Since the proposed algorithm is developed based on actual driving data, it is expected to be more acceptable by drivers. However, the algorithm needs further testing in real-life to validate this expectation.
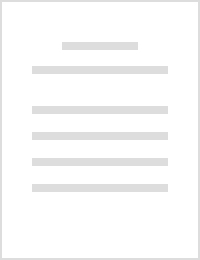
SIMULATION, 2019
Rear-end collisions represent a quarter to one-third of the total number of collisions occurring ... more Rear-end collisions represent a quarter to one-third of the total number of collisions occurring on North American roads. Consequently, Forward Collision Warning (FCW) algorithms have been developed to mitigate this type of critical collision by warning drivers about an impending rear-end event. The algorithms are typically tested to ensure their effectiveness in reducing specific events, such as rear-end conflicts and/or collisions, or by assessing the change in the frequency and severity of braking maneuvers. Such assessments are usually microscopic in nature and deal with isolated (independent) situations. This paper aims at assessing six FCW algorithms at a network level with varying market penetration rates using a calibrated micro-simulation model. The algorithms were assessed in terms of their safety (rear-end conflicts frequency), mobility (travel times), and environmental impacts (emissions and fuel consumption). Based on the results of this study, most of the FCW algorithms did not have a significant effect on mobility nor environmental impacts at various market penetration rates. On the contrary, all the algorithms showed significant safety improvements, in terms of reducing rear-end conflicts, as the market penetration rates increased. The only exception was a single algorithm that tends to be more conservative in terms of braking distance. The results showed that situational improvements (on a driver level) caused by using FCW systems will generally translate into systematic improvements (on a network level). This is important due to the anticipated gradual increase in intelligent vehicles, which are expected to be equipped with FCW systems, on our roads soon.
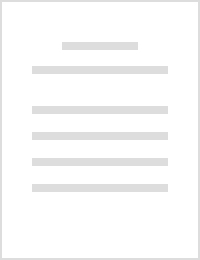
Transportation Research Record, 2019
Safety Performance Functions (SPFs) are regression models used to predict the expected number of ... more Safety Performance Functions (SPFs) are regression models used to predict the expected number of collisions as a function of various traffic and geometric characteristics. One of the integral components in developing SPFs is the availability of accurate exposure factors, that is, annual average daily traffic (AADT). However, AADTs are not often available for minor roads at rural intersections. This study aims to develop a robust AADT estimation model using a deep neural network. A total of 1,350 rural four-legged, stop-controlled intersections from the Province of Alberta, Canada, were used to train the neural network. The results of the deep neural network model were compared with the traditional estimation method, which uses linear regression. The results indicated that the deep neural network model improved the estimation of minor roads’ AADT by 35% when compared with the traditional method. Furthermore, SPFs developed using linear regression resulted in models with statistically insignificant AADTs on minor roads. Conversely, the SPF developed using the neural network provided a better fit to the data with both AADTs on minor and major roads being statistically significant variables. The findings indicated that the proposed model could enhance the predictive power of the SPF and therefore improve the decision-making process since SPFs are used in all parts of the safety management process.
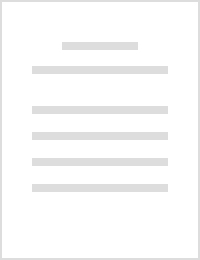
Canadian Journal of Civil Engineering, 2020
This study investigates the car-following behavior during braking at intersections and segments. ... more This study investigates the car-following behavior during braking at intersections and segments. Car-following events were extracted from a naturalistic driving dataset, mapped using ArcGIS, and analyzed to differentiate between the intersection- and segment-related events. The intersection-related events were identified according to an intersection influence area, which was estimated based on the stopping sight distance and the speed limit. Five behavioral measures were quantified based on exploring the probability density functions (PDF) for intersection- and segment-related events. The results showed that there were significant differences between the PDFs of the measures for both cases. Moreover, it was indicated that drivers tend to be more aggressive at intersections compared with segments. Thus, it is crucial to consider the driver’s location when investigating driver behavior. The quantified behavioral measures are a rich data source that can be used for car-following microscopic modeling, surrogate safety analysis, and driver assistance systems development.
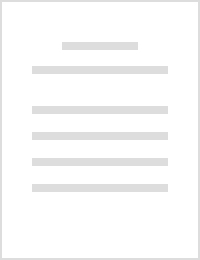
Journal of Transportation Safety & Security , 2020
This study aims at modelling unassisted drivers’ speed at the yellow onset to enhance Connected a... more This study aims at modelling unassisted drivers’ speed at the yellow onset to enhance Connected and Autonomous Vehicles applications at signalised intersections and maximise drivers’ comfort. For this purpose, a total of 2442 real-life vehicle trajectories were analysed to extract driver behavioural measures (i.e. speed, acceleration, and distance to intersection) at different times before the yellow onset. These behavioural measures were used to integrate drivers’ perceptual ability into modelling drivers’ speed at the yellow onset. To develop these models, three machine learning techniques; namely, linear regression, Support Vector Machine, and Neural Networks have been adopted. The best model was a neural network model and was selected based on the goodness-of-fit of the test dataset which has an R-squared value of 0.97. The results indicate that the speed at the yellow onset can be estimated based on behavioural measures while accounting for drivers’ perceptual ability. Also, the model can contribute to a V2I application by assisting the driver in a partially autonomous vehicle to avoid trapping in the dilemma zone and stop safely at signalised intersections. Also, the model can be used to recommend a comfortable riding speed, from a rider’s perspective to a fully autonomous vehicle.
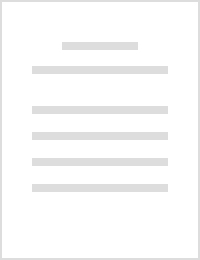
This study proposes an addition to the architecture of the context-aware Driver Assistance System... more This study proposes an addition to the architecture of the context-aware Driver Assistance Systems (DASs) by introducing a context identification layer to the reasoning subsystem. The proposed layer contains two algorithms that work in sequence: the infrastructure detection algorithm and the driver classification algorithm, respectively. The infrastructure detection algorithm aims to identify intersection-related driving when the driver adjusts his or her behavior due to the presence of an intersection ahead. Then, the driver classification algorithm categorizes drivers into cautious, normal, and aggressive at both locations. Data from 64 drivers in a Naturalistic Driving Study was used to prove the concept of the proposed layer. Several behavioral measures were extracted, including following distance, relative speed, headway, acceleration, time-to-collision, and jerk. These behavioral measures were then used to train the algorithms in the context identification layer. The results of both algorithms supported the concept of the proposed layer. These results have implications related to driver behaviors including i) the intersection-related driving behavior can be detected, ii) the drivers tend to be relatively aggressive at intersections when compared to segments, and iii) the driver classification, which ignores the driver’s relative location to intersections, were more likely to misclassify drivers as aggressive when they were in high intersection density areas such as downtown cores. The findings of this study emphasized the importance of context-aware DAS architecture that acknowledges and integrates the variation in driver behavior due to both a change in the surrounding environment and drivers’ individual needs.
Uploads
Papers by Mostafa H Tawfeek