Peer-Reviewed Article by Noemi Vergopolan

Today, the most popular approaches in agricultural forecasting leverage process-based crop models... more Today, the most popular approaches in agricultural forecasting leverage process-based crop models, crop monitoring data, and/or remote sensing imagery. Individually, each of these tools has its own unique advantages but is, nonetheless, limited in prediction accuracy, precision, or both. In this study we integrate in situ and remote sensing (RS) soil moisture observations with APSIM model through sequential data assimilation to evaluate the improvement in model predictions of downstream state variables across 5 experimental sites in the U.S Midwest. Four RS data products and in-situ observations spanning 19 site-years were used through two data assimilation approaches namely Ensemble Kalman Filter (EnKF) and Generalized Ensemble Filter (GEF) to constrain model states at observed time steps and estimate joint background and observation error matrices. Then, the assimilation's impact on estimates of soil moisture, yield, NDVI, tile drainage, and nitrate leaching was assessed across all site-years. When assimilating in situ observations, the accuracy of soil moisture forecasts in the assimilation layers was improved by reducing RMSE by an average of 17% for 10cm and ~28% for 20 cm depth soil layer across all site-years. These changes also led to improved simulation of soil moisture in deeper soil layers by an average of 12%. Although crop yield was improved by an average of 23%, the greatest improvement in yield accuracy was demonstrated in site-years with higher water stress, where assimilation served to increase available soil water for crop uptake. Alternatively, estimates of annual tile drainage and nitrate leaching were not well constrained across the study sites. Trends in drainage constraint suggest the importance of including additional data constraint such as evapotranspiration. The assimilation of RS soil moisture showed weaker constraint of downstream model state variables when compared to the assimilation of in situ soil moisture. The median reduction in soil moisture RMSE for observed soil layers was lower, on average, by a factor of 5. However, crop yield estimates were still improved overall with a median RMSE reduction of 17.2%. Crop yield prediction was improved when assimilating both in-situ and remote sensing soil moisture observations and there is strong evidence that yield improvement was higher when under water-stressed conditions. Comparisons of system performance across different combinations of remote sensing data products indicated the importance of high temporal resolution and accurate observation uncertainty estimates when assimilating surface soil moisture observations.

Science of The Total Environment
Land use and cover change (LUCC) in Brazil encompass a complex interplay of diverse factors acros... more Land use and cover change (LUCC) in Brazil encompass a complex interplay of diverse factors across different biomes. Understanding these dynamics is crucial for informed decision-making and sustainable land management. In this study, we comprehensively analyzed LUCC patterns and drivers using 30 m resolution MapBiomas Collection 6.0 data (1985-2020). By mapping deforestation of primary and secondary natural vegetation, natural vegetation regeneration, and transitions between pasture, soybean, agriculture, and irrigation, we shed light on the intricate nature of LUCC in Brazil. Our findings highlight significant and increasing trends of deforestation in primary vegetation in the country. Simultaneously, the Atlantic Forest, Caatinga, Pampa, and other regions of the Cerrado have experienced intensification processes. Notably, the pasture area in Brazil reached its peak in 2006 and has since witnessed a gradual replacement by soybean and other crops. While pasture-driven deforestation persists in most biomes, the net pasture area has only increased in the Amazon and Pantanal, decreasing in other biomes due to the conversion of pasturelands to intensive cropping in other regions. Our analysis further reveals that primary and secondary vegetation deforestation accounts for a substantial portion of overall forest loss, with
Water Resources Research
Land Surface Models (LSMs) are physically based numerical models that simulate the coupled fluxes... more Land Surface Models (LSMs) are physically based numerical models that simulate the coupled fluxes of water, energy, and carbon cycles on the land-atmosphere interphase (Fisher & Koven, 2020; Vergopolan et al., 2020). Traditionally, LSMs are used to serve as boundary conditions to large-scale models (e.g., Earth System Models (ESMs)) by representing the influence of the land on meteorological processes in terms of energy partitioning, water fluxes, surface roughness, albedo, among others (Fisher & Koven, 2020). As uncoupled models, LSMs also provide spatially distributed, physically based modeling tools, making them critical scientific assets in predicting hydrological variability at various scales. Thus, LSMs allow addressing societal needs for information about water and energy over continental and global domains (
Water Resources Research
The LSM approach is especially appealing given that it does not require observations of soil mois... more The LSM approach is especially appealing given that it does not require observations of soil moisture and instead it is modeled using more readily available topographic, meteorological, soils, and vegetation data (Chaney et al., 2015); however, its reliability is strongly tied to the uncertainties of these data (

Geophysical Research Letters
Soil moisture (SM) spatiotemporal variability critically influences water resources, agriculture,... more Soil moisture (SM) spatiotemporal variability critically influences water resources, agriculture, and climate. However, besides site-specific studies, little is known about how SM varies locally (1–100-m scale). Consequently, quantifying the SM variability and its impact on the Earth system remains a long-standing challenge in hydrology. We reveal the striking variability of local-scale SM across the United States using SMAP-HydroBlocks — a novel satellite-based surface SM data set at 30-m resolution. Results show how the complex interplay of SM with landscape characteristics and hydroclimate is primarily driven by local variations in soil properties. This local-scale complexity yields a remarkable and unique multi-scale behavior at each location. However, very little of this complexity persists across spatial scales. Experiments reveal that on average 48% and up to 80% of the SM spatial information is lost at the 1-km resolution, with complete loss expected at the scale of current state-of-the-art SM monitoring and modeling systems (1–25 km resolution).

Soil moisture plays a key role in controlling land-atmosphere interactions, with implications for... more Soil moisture plays a key role in controlling land-atmosphere interactions, with implications for water resources, agriculture, climate, and ecosystem dynamics. Although soil moisture varies strongly across the landscape, current monitoring capabilities are limited to coarse-scale satellite retrievals and a few regional in-situ networks. Here, we introduce SMAP-HydroBlocks (SMAP-HB), a high-resolution satellite-based surface soil moisture dataset at an unprecedented 30-m resolution (2015--2019) across the conterminous United States. SMAP-HB was produced by using a scalable cluster-based merging scheme that combines high-resolution land surface modeling, radiative transfer modeling, machine learning, SMAP satellite microwave data, and in-situ observations. We evaluated the resulting dataset over 1,192 observational sites. SMAP-HB performed substantially better than the current state-of-the-art SMAP products, showing a median temporal correlation of 0.73±0.13 and a median Kling-Gupta Efficiency of 0.52±0.20. The largest benefit of SMAP-HB is, however, the high spatial detail and improved representation of the soil moisture spatial variability and spatial accuracy with respect to SMAP products. The SMAP-HB dataset is available via https://doi.org/10.5281/zenodo.5206725 and at https://waterai.earth/smaphb

We introduce the Precipitation Probability DISTribution (PPDIST) dataset, a collection of global ... more We introduce the Precipitation Probability DISTribution (PPDIST) dataset, a collection of global high resolution (0.1°) observation-based climatologies (1979-2018) of the occurrence and peak intensity of precipitation (P) at daily and 3-hourly timescales. The climatologies were produced using neural networks trained with daily P observations from 93,138 gauges and hourly P observations (resampled to 3-hourly) from 11,881 gauges worldwide. Mean validation coefficient of determination (R 2) values ranged from 0.76 to 0.80 for the daily P occurrence indices, and from 0.44 to 0.84 for the daily peak P intensity indices. The neural networks performed significantly better than current state-of-the-art reanalysis (ERA5) and satellite (IMERG) products for all P indices. Using a 0.1 mm 3 h −1 threshold, P was estimated to occur 12.2%, 7.4%, and 14.3% of the time, on average, over the global, land, and ocean domains, respectively. The highest P intensities were found over parts of Central America, India, and Southeast Asia, along the western equatorial coast of Africa, and in the intertropical convergence zone. The PPDIST dataset is available via www.gloh2o.org/ppdist.

Remote Sensing of Environment, 2020
Accurate and detailed soil moisture information is essential for, among other things, irrigation,... more Accurate and detailed soil moisture information is essential for, among other things, irrigation, drought and flood prediction, water resources management, and field-scale (i.e., tens of m) decision making. Recent satellite missions measuring soil moisture from space continue to improve the availability of soil moisture information. However, the utility of these satellite products is limited by the large footprint of the microwave sensors. This study presents a merging framework that combines a hyper-resolution land surface model (LSM), a radiative transfer model (RTM), and a Bayesian scheme to merge and downscale coarse resolution remotely sensed hydrological variables to a 30-m spatial resolution. The framework is based on HydroBlocks, an LSM that solves the field-scale spatial heterogeneity of land surface processes through interacting hydrologic response units (HRUs). The framework was demonstrated for soil moisture by coupling HydroBlocks with the Tau-Omega RTM used in the Soil Moisture Active Passive (SMAP) mission. The brightness temperature from the HydroBlocks-RTM and SMAP L3 were merged to obtain updated 30-m soil moisture. We validated the downscaled soil moisture estimates at four experimental watersheds with dense in-situ soil moisture networks in the United States and obtained overall high correlations (> 0.81) and good mean KGE score (0.56). The downscaled product captures the spatial and temporal soil moisture dynamics better than SMAP L3 and L4 product alone at both field and watershed scales. Our results highlight the value of hyper-resolution modeling to bridge the gap between coarsescale satellite retrievals and field-scale hydrological applications.

SWI) smoothing filter resulted in improved performance for all satellite products. The best-to-wo... more SWI) smoothing filter resulted in improved performance for all satellite products. The best-to-worst performance ranking of the four single-sensor satellite products was SMAPL3E SWI , SMOS SWI , AMSR2 SWI , and ASCAT SWI , with the L-band-based SMAPL3E SWI (median R of 0.72) outperforming the others at 50 % of the sites. Among the two multi-sensor satellite products (MeMo and ESA-CCI SWI), MeMo performed better on average (median R of 0.72 versus 0.67), probably due to the inclusion of SMAPL3E SWI. The best-to-worst performance ranking of the six openloop models was HBV-MSWEP, HBV-ERA5, ERA5-Land, HBV-IMERG, VIC-PGF, and GLDAS-Noah. This ranking largely reflects the quality of the precipitation forcing. HBV-MSWEP (median R of 0.78) performed best not just among the open-loop models but among all products. The calibration of HBV improved the median R by +0.12 on average compared to random parameters, highlighting the importance of model calibration. The best-to-worst performance ranking of the six models with satellite data assimilation was HBV-MSWEP+SMAPL3E, HBV-ERA5+SMAPL3E, GLEAM, SMAPL4, HBV-IMERG+SMAPL3E, and ERA5.

Given the varying manifestations of climate change over time and the influence of climate percept... more Given the varying manifestations of climate change over time and the influence of climate perceptions on adaptation, it is important to understand whether farmer perceptions match patterns of environmental change from observational data. We use a combination of social and environmental data to understand farmer perceptions related to rainy season onset. Household surveys were conducted with 1171 farmers across Zambia at the end of the 2015/16 growing season eliciting their perceptions of historic changes in rainy season onset and their heuristics about when rain onset occurs. We compare farmers’ perceptions with satellite-gauge-derived rainfall data from the Climate Hazards Group Infrared Precipitation with Station dataset and hyper-resolution soil moisture estimates from the HydroBlocks land surface model. We find evidence of a cognitive bias, where farmers perceive the rains to be arriving later, although the physical data do not wholly support this. We also find that farmers’ heuristics about rainy season onset influence maize planting dates, a key determinant of maize yield and food security in sub-Saharan Africa. Our findings suggest that policy makers should focus more on current climate variability than future climate change.

Journal of Hydrometeorology, 2017
Dry conditions in 2013–16 in much of the western United States were responsible for severe drough... more Dry conditions in 2013–16 in much of the western United States were responsible for severe drought and led to an exceptional fire season in the Pacific Northwest in 2015. Winter 2015/16 was forecasted to relieve drought in the southern portion of the region as a result of increased precipitation due to a very strong El Niño signal. A student forecasting challenge is summarized in which forecasts of winter hydroclimate across the western United States were made on 1 January 2016 for the winter hydroclimate using several dynamical and statistical forecast methods. They show that the precipitation forecasts had a large spread and none were skillful, while anomalously high observed temperatures were forecasted with a higher skill and precision. The poor forecast performance, particularly for precipitation, is traceable to high uncertainty in the North American Multi-Model Ensemble (NMME) forecast, which appears to be related to the inability of the models to predict an atmospheric block...
Earth's Future
Studies often talk of "diagnosing" drought, a term inspired by medical science that is suggestive... more Studies often talk of "diagnosing" drought, a term inspired by medical science that is suggestive of analysis of symptoms and causes leading to a thorough understanding of an illness or disorder to enable treatment. After all, drought is akin to a chronic and complex disorder for which we wish to lessen symptoms and ideally prevent altogether. However, we show that there is no consensus as to what a "drought diagnosis" entails, neither across nor within disciplines. Yet the medical science literature provides us with the steps of the diagnostic process,
Geoscientific Model Development
tween the land surface and the river network leads to appreciable differences in the simulated sp... more tween the land surface and the river network leads to appreciable differences in the simulated spatial heterogeneity of the surface energy balance, (2) a limited number of HRUs (∼ 300 per 0.25 • cell) are required to approximate the fully distributed simulation adequately, and (3) the surface energy balance partitioning is sensitive to the river routing model parameters. The resulting routing scheme provides an effective and efficient path forward to enable a two-way coupling between the high-resolution river networks and state-of-the-art tiling schemes in ESMs.
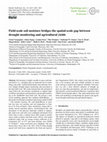
Hydrology and Earth System Sciences, 2021
Soil moisture is highly variable in space and time, and deficits (i.e., droughts) play an importa... more Soil moisture is highly variable in space and time, and deficits (i.e., droughts) play an important role in modulating crop yields. Limited hydroclimate and yield data, however, hamper drought impact monitoring and assessment at the farm field scale. This study demonstrates the potential of using field-scale soil moisture simulations to support high-resolution agricultural yield prediction and drought monitoring at the smallholder farm field scale. We present a multiscale modeling approach that combines HydroBlocks – a physically based hyper-resolution land surface model (LSM) – with machine learning. We used HydroBlocks to simulate root zone soil moisture and soil temperature in Zambia at 3 h 30 m resolution. These simulations, along with remotely sensed vegetation indices, meteorological data, and descriptors of the physical landscape (related to topography, land cover, and soils) were combined with district-level maize data to train a random forest (RF) model to predict maize yields at district and field scales (250 m). Our model predicted yields with an average testing coefficient of determination (R2) of 0.57 and mean absolute error (MAE) of 310 kg ha−1 using year-based cross-validation. Our predicted maize losses due to the 2015–2016 El Niño drought agreed well with losses reported by the Food and Agriculture Organization (FAO). Our results reveal that soil moisture is the strongest and most reliable predictor of maize yield, driving its spatial and temporal variability. Soil moisture was also a more effective indicator of drought impacts on crops than precipitation, soil and air temperatures, and remotely sensed normalized difference vegetation index (NDVI)-based drought indices. This study demonstrates how field-scale modeling can help bridge the spatial-scale gap between drought monitoring and agricultural impacts.

. Soil moisture is highly variable in space, and its deficits (i.e. droughts) plays an important ... more . Soil moisture is highly variable in space, and its deficits (i.e. droughts) plays an important role in modulating crop yields and its variability across landscapes. Limited hydroclimate and yield data, however, hampers drought impact monitoring and assessment at the farmer field-scale. This study demonstrates the potential of field-scale soil moisture simulations to advance high-resolution agricultural yield prediction and drought monitoring at the smallholder farm field-scale. We present a multi-scale modeling approach that combines HydroBlocks, a physically-based hyper-resolution Land Surface Model (LSM), and machine learning. We applied HydroBlocks to simulate root zone soil moisture and soil temperature in Zambia at 3-hourly 30-m resolution. These simulations along with remotely sensed vegetation indices, meteorological conditions, and data describing the physical properties of the landscape (topography, land cover, soil properties) were combined with district-level maize data to train a random forest model (RF) to predict maize yields at the district- and field-scale (250-m) levels. Our model predicted yields with a coefficient of variation (R2) of 0.61, Mean Absolute Error (MAE) of 349 kg ha−1, and mean normalized error of 22 %. We captured maize losses due to the 2015/2016 El Niño drought at similar levels to losses reported by the Food and Agriculture Organization (FAO). Our results revealed that soil moisture is the strongest and most reliable predictor of maize yield, driving its spatial and temporal variability. Consequently, soil moisture was also the most effective indicator of drought impacts in crops when compared with precipitation, soil and air temperatures, and remotely-sensed NDVI-based drought indices. By combining field-scale root zone soil moisture estimates with observed maize yield data, this research demonstrates how field-scale modeling can help bridge the spatial scale discontinuity gap between drought monitoring and agricultural impacts.

The International Journal of High Performance Computing Applications
Helicopters can experience brownout when flying close to a dusty surface. The uplifting of dust i... more Helicopters can experience brownout when flying close to a dusty surface. The uplifting of dust in the air can remarkably restrict the pilot’s visibility area. Consequently, a brownout can disorient the pilot and lead to the helicopter collision against the ground. Given its risks, brownout has become a high-priority problem for civil and military operations. Proper helicopter design is thus critical, as it has a strong influence over the shape and density of the cloud of dust that forms when brownout occurs. A way forward to improve aircraft design against brownout is the use of particle simulations. For simulations to be accurate and comparable to the real phenomenon, billions of particles are required. However, using a large number of particles, serial simulations can be slow and too computationally expensive to be performed. In this work, we investigate an message passing interface (MPI) + graphics processing unit (multi-GPU) approach to simulate brownout. In specific, we use a ...

Remote Sensing of Environment
Soil Moisture (SM) is a direct measure of agricultural drought. While there are several global SM... more Soil Moisture (SM) is a direct measure of agricultural drought. While there are several global SM indices, none of them directly use SM observations in a near-real-time capacity and as an operational tool. This paper presents a near-real-time global SM index monitor based on integrated SMAP (Soil Moisture Active Passive) and SMOS (Soil Moisture and Ocean Salinity) remote sensing data. We make use of the short period (2015-2018) of SMAP datasets in combination with two approaches-Cumulative Distribution Function Mapping (CDFM) and Bayesian conditional process-and integrate them with SMOS data in a way that SMOS data is consistent with SMAP. The integrated SMOS and SMAP (SMOS/SMAP) has an increased global revisit frequency and a period of record from 2010 to the present. A four-parameter Beta distribution was fitted to the SMOS/SMAP dataset for each calendar month of each grid cell at~36 km resolution for the period from 2010 to 2018. We used an asymptotic method that guarantees the values of the bounding parameters of the Beta distribution will envelop both the smallest and largest observed values. The Kolmogorov-Smirnov (KS) test showed that more grids globally will pass if the integrated dataset is from the Bayesian conditional approach. A daily global SM index map is generated and posted online based on translating each grid's integrated SM value for that day to a corresponding probability percentile relevant to the particular calendar month from 2010 to 2018. For validation, we use the Canadian Prairies Ecozone (CPE). We compare the integrated SM with the SMAP core validation and RISMA sites from ISMN, compare our indices with other models (VIC, ESA's CCI SM v04.4 integrated satellite data, and SPI-1), and make a two-by-two comparison of candidate indices using heat maps and summary CDF statistics. Furthermore, we visually compare our global SM-based index maps with those produced by other organizations. Our Global SM Index Monitor (GSMIM) performed, in many tests, similarly to the CCI's product SM index but with the advantage of being a near-real-time tool, which has applications for identifying evolving drought for food security conditions, insurance, policymaking, and crop planning especially for the remote parts of the globe.

Soil Moisture (SM) is a direct measure of agricultural drought. While there are several global SM... more Soil Moisture (SM) is a direct measure of agricultural drought. While there are several global SM indices, none of them directly use SM observations in a near-real-time capacity and as an operational tool. This paper presents a near-real-time global SM index monitor based on integrated SMAP (Soil Moisture Active Passive) and SMOS (Soil Moisture and Ocean Salinity) remote sensing data. We make use of the short period (2015-2018) of SMAP datasets in combination with two approaches-Cumulative Distribution Function Mapping (CDFM) and Bayesian conditional process-and integrate them with SMOS data in a way that SMOS data is consistent with SMAP. The integrated SMOS and SMAP (SMOS/SMAP) has an increased global revisit frequency and a period of record from 2010 to the present. A four-parameter Beta distribution was fitted to the SMOS/SMAP dataset for each calendar month of each grid cell at~36 km resolution for the period from 2010 to 2018. We used an asymptotic method that guarantees the values of the bounding parameters of the Beta distribution will envelop both the smallest and largest observed values. The Kolmogorov-Smirnov (KS) test showed that more grids globally will pass if the integrated dataset is from the Bayesian conditional approach. A daily global SM index map is generated and posted online based on translating each grid's integrated SM value for that day to a corresponding probability percentile relevant to the particular calendar month from 2010 to 2018. For validation , we use the Canadian Prairies Ecozone (CPE). We compare the integrated SM with the SMAP core validation and RISMA sites from ISMN, compare our indices with other models (VIC, ESA's CCI SM v04.4 integrated satellite data, and SPI-1), and make a two-by-two comparison of candidate indices using heat maps and summary CDF statistics. Furthermore, we visually compare our global SM-based index maps with those produced by other organizations. Our Global SM Index Monitor (GSMIM) performed, in many tests, similarly to the CCI's product SM index but with the advantage of being a near-real-time tool, which has applications for identifying evolving drought for food security conditions, insurance, policymaking, and crop planning especially for the remote parts of the globe.

Hydrology and Earth System Sciences
We undertook a comprehensive evaluation of 22 gridded (quasi-)global (sub-)daily precipitation (P... more We undertook a comprehensive evaluation of 22 gridded (quasi-)global (sub-)daily precipitation (P ) datasets for the period 2000-2016. Thirteen non-gauge-corrected P datasets were evaluated using daily P gauge observations from 76 086 gauges worldwide. Another nine gaugecorrected datasets were evaluated using hydrological modeling, by calibrating the HBV conceptual model against streamflow records for each of 9053 small to mediumsized ( < 50 000 km 2 ) catchments worldwide, and comparing the resulting performance. Marked differences in spatiotemporal patterns and accuracy were found among the datasets. Among the uncorrected P datasets, the satellite-and reanalysis-based MSWEP-ng V1.2 and V2.0 datasets generally showed the best temporal correlations with the gauge observations, followed by the reanalyses (ERA-Interim, JRA-55, and NCEP-CFSR) and the satellite-and reanalysis-based CHIRP V2.0 dataset, the estimates based primarily on passive microwave remote sensing of rainfall (CMORPH V1.0, GSMaP V5/6, and TMPA 3B42RT V7) or near-surface soil moisture (SM2RAIN-ASCAT), and finally, estimates based primarily on thermal infrared imagery (GridSat V1.0, PER-SIANN, and PERSIANN-CCS). Two of the three reanalyses (ERA-Interim and JRA-55) unexpectedly obtained lower trend errors than the satellite datasets. Among the corrected P datasets, the ones directly incorporating daily gauge data (CPC Unified, and MSWEP V1.2 and V2.0) generally pro-vided the best calibration scores, although the good performance of the fully gauge-based CPC Unified is unlikely to translate to sparsely or ungauged regions. Next best results were obtained with P estimates directly incorporating temporally coarser gauge data (CHIRPS V2.0, GPCP-1DD V1.2, TMPA 3B42 V7, and WFDEI-CRU), which in turn outperformed the one indirectly incorporating gauge data through another multi-source dataset (PERSIANN-CDR V1R1). Our results highlight large differences in estimation accuracy, and hence the importance of P dataset selection in both research and operational applications. The good performance of MSWEP emphasizes that careful data merging can exploit the complementary strengths of gauge-, satellite-, and reanalysis-based P estimates.

Hydrology and Earth System Sciences
We undertook a comprehensive evaluation of 22 gridded (quasi-)global (sub-)daily precipitation (P... more We undertook a comprehensive evaluation of 22 gridded (quasi-)global (sub-)daily precipitation (P ) datasets for the period 2000-2016. Thirteen non-gauge-corrected P datasets were evaluated using daily P gauge observations from 76 086 gauges worldwide. Another nine gaugecorrected datasets were evaluated using hydrological modeling, by calibrating the HBV conceptual model against streamflow records for each of 9053 small to mediumsized ( < 50 000 km 2 ) catchments worldwide, and comparing the resulting performance. Marked differences in spatiotemporal patterns and accuracy were found among the datasets. Among the uncorrected P datasets, the satellite-and reanalysis-based MSWEP-ng V1.2 and V2.0 datasets generally showed the best temporal correlations with the gauge observations, followed by the reanalyses (ERA-Interim, JRA-55, and NCEP-CFSR) and the satellite-and reanalysis-based CHIRP V2.0 dataset, the estimates based primarily on passive microwave remote sensing of rainfall (CMORPH V1.0, GSMaP V5/6, and TMPA 3B42RT V7) or near-surface soil moisture (SM2RAIN-ASCAT), and finally, estimates based primarily on thermal infrared imagery (GridSat V1.0, PER-SIANN, and PERSIANN-CCS). Two of the three reanalyses (ERA-Interim and JRA-55) unexpectedly obtained lower trend errors than the satellite datasets. Among the corrected P datasets, the ones directly incorporating daily gauge data (CPC Unified, and MSWEP V1.2 and V2.0) generally pro-vided the best calibration scores, although the good performance of the fully gauge-based CPC Unified is unlikely to translate to sparsely or ungauged regions. Next best results were obtained with P estimates directly incorporating temporally coarser gauge data (CHIRPS V2.0, GPCP-1DD V1.2, TMPA 3B42 V7, and WFDEI-CRU), which in turn outperformed the one indirectly incorporating gauge data through another multi-source dataset (PERSIANN-CDR V1R1). Our results highlight large differences in estimation accuracy, and hence the importance of P dataset selection in both research and operational applications. The good performance of MSWEP emphasizes that careful data merging can exploit the complementary strengths of gauge-, satellite-, and reanalysis-based P estimates.
Uploads
Peer-Reviewed Article by Noemi Vergopolan