Papers by Praveen Sankaran
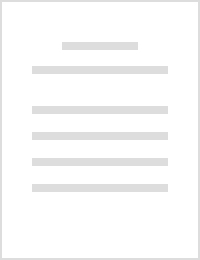
Smart Environment for Smart Cities, 2019
Smart Water is an essential component of the smart environment since life, sustenance, growth and... more Smart Water is an essential component of the smart environment since life, sustenance, growth and death of the environment depends on water. The concept of smart water is derived from the concept of smart cities having one to one relationship in their building blocks. Smart Water Management is a very high responsive, intelligent digital system operated by IOTs and ICTs, clouds, related computer-based models along with humans to identify water-related issues and even automatically using artificial intelligence solving it in real time without human interventions. The chapter presents an attempt to develop a Smart Water Management for Kozhikode Metropolitan area. Water in the study area is studied in conjunction with the spatial distribution of community in a watershed. The issues arising out of the present and potential usage pattern for households for community wellbeing and economic development is the basis of water resources management in Kozhikode Metropolitan Area (KMA). KMA enjoys substantial precipitation a year and is bound by two major rivers on both sides and are lined by many streams within its jurisdiction. However, KMA faces many water-related problems. A SWOT analysis was performed to identify and consolidate the capabilities of various watersheds and its communities in the study area. The issues faced by various communities were grouped into few categories and solutions were proposed for each community. Integrated Smart Water Management is proposed as a solution to the problems faced by the communities. It is footed on the principles of Water democracy, which is implemented through a system of ICT, IoT and decision support systems. The Integrated Smart Water Management System enables the community to be aware of the issues well in advance and find and implement solutions proactively. A spatial decision support system (SDSS) is proposed to help the community to take decisions related to water management. The SDSS takes many of the decisions as per the set procedure and alerts the community only cases where a systematic solution is not available. The integrated water management system implements the decisions taken by the SDSS, through its automatic sensors and actuators managing the water resources.
Theoretical Issues in Ergonomics Science, 2022
2017 IEEE International Conference on Signal Processing, Informatics, Communication and Energy Systems (SPICES)
The number of deaths due to the motor vehicle accidents has increased drastically during the last... more The number of deaths due to the motor vehicle accidents has increased drastically during the last few years. It is estimated that nearly 40–50 % of the accidents are due to the distractions of the driver. One of the methods to assess driver distraction is using head pose estimation. The unavailability of a standard driving database hampers the study and testing of driver distraction algorithms. In this paper we present the development and bench-marking of the Distracted Car Driver (DCD) database. The database contains real time driving videos of 12 different individuals, in varying environmental conditions. To bench-mark the database we use standard linear and nonlinear manifold techniques for data embedding.
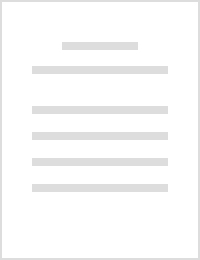
2018 International Conference on Data Science and Engineering (ICDSE)
Driver distraction has remained a significant factor in a large number of motor-vehicle accidents... more Driver distraction has remained a significant factor in a large number of motor-vehicle accidents. Detection of driver inattention is considered a major potential area of research so as to prevent a huge number of road accidents. This paper presents a methodology that utilizes deep neural networks to analyze driver distraction. A tuned convolutional neural network for the vehicle driver image set is developed and used to classify face pose and this information is used to estimate driver distraction. The final proposed network consists of five convolutional layers, few of them followed by pooling layers, ReLU layers and finally three fully connected layers. The network is trained and tested using the vehicle driver database developed during real time vehicle operation by 14 individuals in multiple scenarios. The proposed approach resulted in better accuracy when compared to standard linear and nonlinear embedding based algorithms.
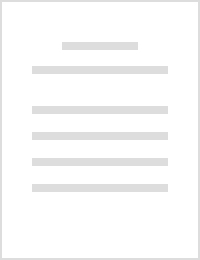
2018 International Conference on Data Science and Engineering (ICDSE)
Haze is a common atmospheric degradation which reduces the visibility of outdoor images by creati... more Haze is a common atmospheric degradation which reduces the visibility of outdoor images by creating a thin veil over the scene. Removal of haze from a natural scene is a complex process because of the difficulty in getting any prior information other than the hazy image. In natural images, the assumption of global atmospheric light may not be true because of the non uniform nature of haze. Most of the state-of-the-art methods use a patch based algorithm for the estimation of global atmospheric light and transmission map. In this work, we propose a patch based dehazing technique which estimates localized atmospheric light by clustering the minimum channel of the image using Self Organizing Map (SOM). This local atmospheric light is used in haze-line based dehazing method for the estimation of transmission map. It gives a better estimate of haze content compared to the existing methods and results in better dehazing. The pixel based haze-line model has reduced complexity when compared to dark channel prior based methods. This excludes the requirement of any transmission map refinement operations which makes the algorithm simpler and faster.
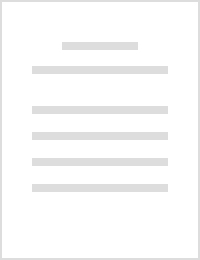
Expert Systems with Applications
Abstract Vehicle driver distraction is one of the major reasons for road accidents. Involvement w... more Abstract Vehicle driver distraction is one of the major reasons for road accidents. Involvement with a co-passenger, use of in-vehicle devices or phone leads to a situation where the driver head pose varies and the eye is off the road. A low cost early warning system should reduce the distracted driving instances, thus making our roads safer. Face pose information forms an important cue to determine driver distraction. The main objective of this work is to analyse the distractions of the driver based on his/her face pose cues. A straight pose or slight variation would indicate a non-distracted driver, while a large pose variation from the center would indicate a high probability for a distracted driver. Face pose database of vehicle drivers is developed and is bench-marked. A clustered two layer approach on Gabor features is proposed. A five layer convolutional network with three fully connected layers is also used to bench-mark the data. The proposed clustered two-layer approach with Gabor features and SVM classifier provides better results in driver distraction analysis when compared to the deep learning approach and other manifold approaches. The improved accuracy could be attributed to the improved modeling of manifold in our approach, better class discrimination of the Gabor features together with better classification provided by the SVM classifier.
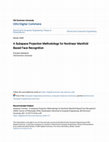
A novel feature extraction method that utilizes nonlinear mapping from the original data space to... more A novel feature extraction method that utilizes nonlinear mapping from the original data space to the feature space is presented in this dissertation. Feature extraction methods aim to find compact representations of data that are easy to classify. Measurements with similar values are grouped to same category, while those with differing values are deemed to be of separate categories. For most practical systems, the meaningful features of a pattern class lie in a low dimensional nonlinear constraint region (manifold) within the high dimensional data space. A learning algorithm to model this nonlinear region and to project patterns to this feature space is developed. Least squares estimation approach that utilizes interdependency between points in training patterns is used to form the nonlinear region. The proposed feature extraction strategy is employed to improve face recognition accuracy under varying illumination conditions and facial expressions. Though the face features show var...
2015 IEEE Recent Advances in Intelligent Computational Systems (RAICS), 2015
This paper proposes a clustering landmark selection technique for Landmark Isomap (L-Isomap). L-I... more This paper proposes a clustering landmark selection technique for Landmark Isomap (L-Isomap). L-Isomap randomly selects a set of points called landmark points from the data set, for computing the distance from the selected landmark points to all other non landmark points. Selection of the landmark points is crucial in proper representation of the data. The number of landmark points selected and the location of these points will be dependent on the data properties. The proposed method when compared with random L-Isomap and Isomap, performs well for different landmark points for different databases.
Signal Processing: Image Communication
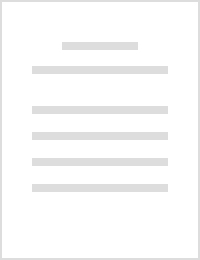
TENCON 2019 - 2019 IEEE Region 10 Conference (TENCON), 2019
Despite the significant progress in diagnosis and treatment, breast cancer still continues to be ... more Despite the significant progress in diagnosis and treatment, breast cancer still continues to be the most common deadly disease occurring among women in the world. Histopathological analysis is the standard method for breast cancer diagnosis. Manual inspection of histological samples is a highly specialized and time consuming task. Computer-Aided Diagnosis Systems (CAD) could overcome the limitations of manual analysis and assist pathologists to diagnose cancer more accurately and efficiently. Hence, we propose a novel approach that uses deep learned nucleus feature classifier fusion framework for breast cancer detection from histopathological images. Recently, convolutional neural networks (CNNs) have produced high recognition rates compared to hand crafted features but results in much higher complexity in network design. Moreover, designing CNN from scratch requires large amount of training data. So, we use pretrained CNNs for feature extraction. Extracted features are fused using...
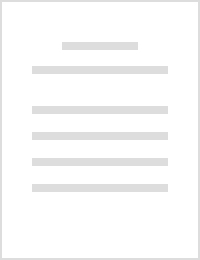
Communications in Computer and Information Science
Classification is a machine learning technique that is used to find the membership of an object t... more Classification is a machine learning technique that is used to find the membership of an object to a given set of classes or groups. Neural network (NN) classifier always suffers some issues while examining outliers and data points from nearby classes. In this paper, we present a new hyper-credal neural network classifier based on belief theory. This method is based on credal classification technique introduced in Dempster-Shafer Theory (DST). It allows a data point to belong not only to a specific class but also to a meta-class or an ignorant class based on its mass. The sample which lies in an overlapping region is accurately classified in this method to a meta-class which corresponds to the union of the overlapping classes. Therefore this approach reduces the classification error at the price of precision. But this decrease in precision is acceptable in applications such as medical, defence related applications where a wrong decision would cost more than avoiding some correct decisions. The results and analysis of different databases are given to illustrate the potential of this approach. This idea of hyper-credal classification is extended to convolutional neural network (CNN) classifiers also.
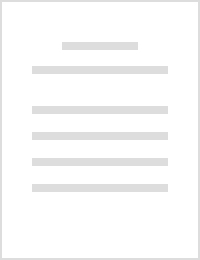
2019 International Conference on Information Technology (ICIT), 2019
Human errors are considered to be one of the most common reasons for road accidents. Autonomous d... more Human errors are considered to be one of the most common reasons for road accidents. Autonomous driving technology has the potential to reduce the number of accidents to a large extent by eliminating this error. Estimating the speed of vehicles is a capability of utmost importance for a self driving vehicle for accident free autonomous navigation. Hence speed estimation is an area which has been extensively researched in the recent years. This paper presents a novel, stereo vision based algorithm for the speed estimation problem. This algorithm involves detecting various obstacles on the road for two consecutive frames using YOLO followed by matching the features of the detected objects using Scale Invariant Feature Transform (SIFT). This algorithm also leverages the MC CNN architecture for finding the disparity map of the frames. The algorithm developed is applied on the KITTI dataset. Once calculated, the position and speed of the obstacles can then be fed to the path planning and...
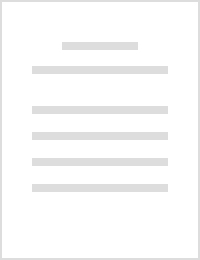
2018 International Conference on Data Science and Engineering (ICDSE), 2018
Driver distraction has remained a significant factor in a large number of motor-vehicle accidents... more Driver distraction has remained a significant factor in a large number of motor-vehicle accidents. Detection of driver inattention is considered a major potential area of research so as to prevent a huge number of road accidents. This paper presents a methodology that utilizes deep neural networks to analyze driver distraction. A tuned convolutional neural network for the vehicle driver image set is developed and used to classify face pose and this information is used to estimate driver distraction. The final proposed network consists of five convolutional layers, few of them followed by pooling layers, ReLU layers and finally three fully connected layers. The network is trained and tested using the vehicle driver database developed during real time vehicle operation by 14 individuals in multiple scenarios. The proposed approach resulted in better accuracy when compared to standard linear and nonlinear embedding based algorithms.
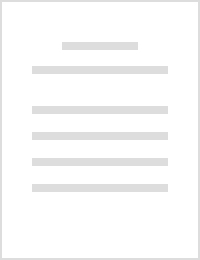
In information processing domains, high dimensional data poses several challenges in terms of sto... more In information processing domains, high dimensional data poses several challenges in terms of storage, visualization, and retrieval. There are several instances where, even though the data points are of high dimension, the data actually resides on a lower dimensional space. Dimensionality reduction methods attempt to find meaningful representation of data which is present in the lower dimension leading to better visualization, removal of noisy features and redundant information. Traditional linear dimensionality reduction techniques are incapable of dealing with nonlinear datasets. Nonlinear dimensionality reduction methods like Isomap work well for such datasets, but suffer from the issue of out-of-sample extension. In this paper, a solution for out-of-sample problem of Isomap method and extended Isomap method is put forward by employing neural networks. Out-of-sample extensions of Isomap and extended Isomap using deep neural network (DNN) are proposed. The proposed method is teste...
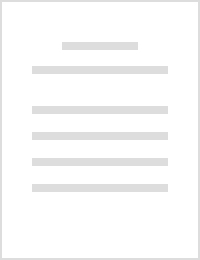
The electrocardiogram (ECG) signal provides a useful non-interventional method for identifying ca... more The electrocardiogram (ECG) signal provides a useful non-interventional method for identifying cardiac arrhythmia. In this paper, we look at automatic ECG beat classification into 2 categories-Normal and Premature ventricular contraction using Dempster Shafer Theory (DST). In biomedical signal classification problems, the cost of making an erroneous decision can be high. Deferring a decision rather than taking a wrong decision might be beneficial. This is done by using the evidential k nearest neighbours (EKNN) approach which is based on Dempster Shafer Theory for classifying the ECG beats. RR interval features are used. Analysis is done on the MIT-BIH database. Performance evaluation is done by considering error rates. Performance of EKNN is compared with the traditional k nearest neighbours (maximum voting) approach. Effect of training data size is assessed by using training sets of varying sizes. Effect of using different distance measures on KNN and EKNN performance is studied. ...
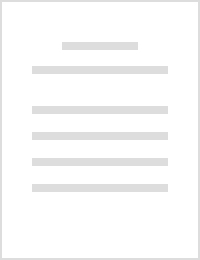
2018 International Conference on Data Science and Engineering (ICDSE), 2018
Haze is a common atmospheric degradation which reduces the visibility of outdoor images by creati... more Haze is a common atmospheric degradation which reduces the visibility of outdoor images by creating a thin veil over the scene. Removal of haze from a natural scene is a complex process because of the difficulty in getting any prior information other than the hazy image. In natural images, the assumption of global atmospheric light may not be true because of the non uniform nature of haze. Most of the state-of-the-art methods use a patch based algorithm for the estimation of global atmospheric light and transmission map. In this work, we propose a patch based dehazing technique which estimates localized atmospheric light by clustering the minimum channel of the image using Self Organizing Map (SOM). This local atmospheric light is used in haze-line based dehazing method for the estimation of transmission map. It gives a better estimate of haze content compared to the existing methods and results in better dehazing. The pixel based haze-line model has reduced complexity when compared...
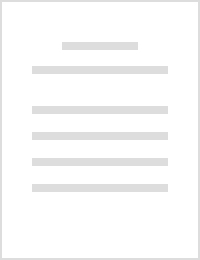
Classification is a machine learning technique that is used to find the membership of an object t... more Classification is a machine learning technique that is used to find the membership of an object to a given set of classes or groups. Neural network (NN) classifier always suffers some issues while examining outliers and data points from nearby classes. In this paper, we present a new hyper-credal neural network classifier based on belief theory. This method is based on credal classification technique introduced in Dempster-Shafer Theory (DST). It allows a data point to belong not only to a specific class but also to a meta-class or an ignorant class based on its mass. The sample which lies in an overlapping region is accurately classified in this method to a meta-class which corresponds to the union of the overlapping classes. Therefore this approach reduces the classification error at the price of precision. But this decrease in precision is acceptable in applications such as medical, defence related applications where a wrong decision would cost more than avoiding some correct dec...
Distracted driving is the act of driving when engaged in other activities which takes the driver’... more Distracted driving is the act of driving when engaged in other activities which takes the driver’s attention away from the road. It is one among the main causes of road accidents. Majority of these accidents occur because of momentary negligence, thus a driver monitoring system which executes and analyzes in real time is necessary. The required dataset will be prepared and this data will be utilised to train the neural network. The major areas of focus are extreme head poses, yawning detection, facial expressions, head nodding and hand movements that are indicators of distraction. Emphasis will also be given in exploring different ensemble methods for combining various existing models.
Uploads
Papers by Praveen Sankaran