Papers by Benjamin Glicksberg
Nature Medicine
T he scientific, academic, medical and data science communities have come together in the face of... more T he scientific, academic, medical and data science communities have come together in the face of the COVID-19 pandemic crisis to rapidly assess novel paradigms in artificial intelligence (AI) that are rapid and secure, and potentially incentivize data sharing and model training and testing without the usual privacy and data ownership hurdles of conventional collaborations 1,2. Healthcare providers, researchers and industry have pivoted their focus to address unmet and critical clinical needs created by the crisis, with remarkable results 3-9. Clinical trial recruitment has been expedited and facilitated by national regulatory bodies and an international cooperative spirit 10-12. The data analytics and AI disciplines have always fostered open
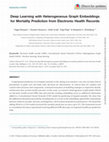
Data Intelligence
Computational prediction of in-hospital mortality in the setting of an intensive care unit can he... more Computational prediction of in-hospital mortality in the setting of an intensive care unit can help clinical practitioners to guide care and make early decisions for interventions. As clinical data are complex and varied in their structure and components, continued innovation of modelling strategies is required to identify architectures that can best model outcomes. In this work, we trained a Heterogeneous Graph Model (HGM) on electronic health record (EHR) data and used the resulting embedding vector as additional information added to a Convolutional Neural Network (CNN) model for predicting in-hospital mortality. We show that the additional information provided by including time as a vector in the embedding captured the relationships between medical concepts, lab tests, and diagnoses, which enhanced predictive performance. We found that adding HGM to a CNN model increased the mortality prediction accuracy up to 4%. This framework served as a foundation for future experiments invol...
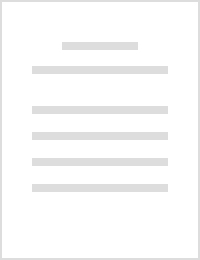
Background. Current lipid-lowering drugs often leave significant residual risk for adverse outcom... more Background. Current lipid-lowering drugs often leave significant residual risk for adverse outcomes. Identification of previously approved drugs for new indications, drug repurposing, may provide a cost effective alternative to de novo drug developing.Objectives. We combined clinical, transcriptomic, computational, and experimental strategies to explore lipid-lowering and plaque-stabilizing effects of atypical antidepressant trazodone.Methods. First, a connectivity mapping strategy was used to match rosuvastatin gene expression signature derived from a clinical trial of 85 patients with to the expression patterns of 1,309 different small molecules to discover a similarity between the rosuvastatin and trazodone gene expression signatures. Then, we assessed the lipid-lowering ability of trazodone in vitro using HepG2 cells and in vivo using molecular imaging of rabbit atherosclerotic lesions. In addition, we analyzed electronic medical records of patients from three large medical cent...
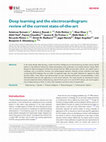
EP Europace
In the recent decade, deep learning, a subset of artificial intelligence and machine learning, ha... more In the recent decade, deep learning, a subset of artificial intelligence and machine learning, has been used to identify patterns in big healthcare datasets for disease phenotyping, event predictions, and complex decision making. Public datasets for electrocardiograms (ECGs) have existed since the 1980s and have been used for very specific tasks in cardiology, such as arrhythmia, ischemia, and cardiomyopathy detection. Recently, private institutions have begun curating large ECG databases that are orders of magnitude larger than the public databases for ingestion by deep learning models. These efforts have demonstrated not only improved performance and generalizability in these aforementioned tasks but also application to novel clinical scenarios. This review focuses on orienting the clinician towards fundamental tenets of deep learning, state-of-the-art prior to its use for ECG analysis, and current applications of deep learning on ECGs, as well as their limitations and future area...
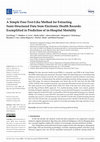
Big Data and Cognitive Computing
The Epic electronic health record (EHR) is a commonly used EHR in the United States. This EHR con... more The Epic electronic health record (EHR) is a commonly used EHR in the United States. This EHR contain large semi-structured “flowsheet” fields. Flowsheet fields lack a well-defined data dictionary and are unique to each site. We evaluated a simple free-text-like method to extract these data. As a use case, we demonstrate this method in predicting mortality during emergency department (ED) triage. We retrieved demographic and clinical data for ED visits from the Epic EHR (1/2014–12/2018). Data included structured, semi-structured flowsheet records and free-text notes. The study outcome was in-hospital death within 48 h. Most of the data were coded using a free-text-like Bag-of-Words (BoW) approach. Two machine-learning models were trained: gradient boosting and logistic regression. Term frequency-inverse document frequency was employed in the logistic regression model (LR-tf-idf). An ensemble of LR-tf-idf and gradient boosting was evaluated. Models were trained on years 2014–2017 and...
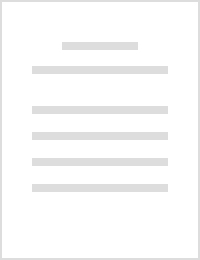
Background: Alzheimer’s disease (AD) is a progressive neurodegenerative disorder and the most com... more Background: Alzheimer’s disease (AD) is a progressive neurodegenerative disorder and the most common cause of dementia in the United States. In spite of evidence of females having a greater lifetime risk of developing Alzheimer’s Disease (AD) and greater apolipoprotein E4-related (apoE4) AD risk compared to males, molecular signatures underlying these findings remain elusive. Methods: We took a meta-analysis approach to study gene expression in the brains of 1,084 AD patients and age-matched controls and whole blood from 645 AD patients and age-matched controls in seven independent datasets. Sex-specific gene expression patterns were investigated through use of gene-based, pathway-based and network-based approaches. The ability of a sex-specific AD gene expression signature to distinguish Alzheimer’s disease from healthy controls was assessed using a linear support vector machine model. Cell type deconvolution from whole blood gene expression data was performed to identify different...
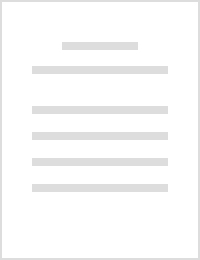
ABSTRACTBackgroundChanges in autonomic nervous system function, characterized by heart rate varia... more ABSTRACTBackgroundChanges in autonomic nervous system function, characterized by heart rate variability (HRV), have been associated with and observed prior to the clinical identification of infection. We performed an evaluation of this metric collected by wearable devices, to identify and predict Coronavirus disease 2019 (COVID-19) and its related symptoms.MethodsHealth care workers in the Mount Sinai Health System were prospectively followed in an ongoing observational study using the custom Warrior Watch Study App which was downloaded to their smartphones. Participants wore an Apple Watch for the duration of the study measuring HRV throughout the follow up period. Survey’s assessing infection and symptom related questions were obtained daily.FindingsUsing a mixed-effect COSINOR model the mean amplitude of the circadian pattern of the standard deviation of the interbeat interval of normal sinus beats (SDNN), a HRV metric, differed between subjects with and without COVID-19 (p=0.006...
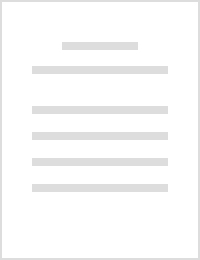
Clinical Journal of the American Society of Nephrology
Background and objectivesSepsis-associated AKI is a heterogeneous clinical entity. We aimed to ag... more Background and objectivesSepsis-associated AKI is a heterogeneous clinical entity. We aimed to agnostically identify sepsis-associated AKI subphenotypes using deep learning on routinely collected data in electronic health records.Design, setting, participants, & measurementsWe used the Medical Information Mart for Intensive Care III database, which consists of electronic health record data from intensive care units in a tertiary care hospital in the United States. We included patients ≥18 years with sepsis who developed AKI within 48 hours of intensive care unit admission. We then used deep learning to utilize all available vital signs, laboratory measurements, and comorbidities to identify subphenotypes. Outcomes were mortality 28 days after AKI and dialysis requirement.ResultsWe identified 4001 patients with sepsis-associated AKI. We utilized 2546 combined features for K-means clustering, identifying three subphenotypes. Subphenotype 1 had 1443 patients, and subphenotype 2 had 189...
Clinical Journal of the American Society of Nephrology
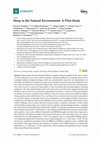
Sleep quality has been directly linked to cognitive function, quality of life, and a variety of s... more Sleep quality has been directly linked to cognitive function, quality of life, and a variety of serious diseases across many clinical domains such as psychiatry and cardiology. Standard methods for assessing sleep involve overnight studies in hospital settings, which are uncomfortable, expensive, not representative of real sleep, and difficult to conduct on a large scale. Recently, a number of commercial digital devices have been developed that record physiological data which can act as a proxy for sleep quality in lieu of standard electroencephalogram recording equipment. Each device company makes different claims of accuracy and measures different features of sleep quality, and it is still unknown how well these devices correlate with one another and perform in a research setting. In this pilot study of 21 participants, we investigated whether outputs from four sensors, specifically FitBit, Withings Aura, Hexoskin, and Oura Ring, were related to known cognitive and psychological m...
Bioinformatics, Convergence Science, and Systems Biology
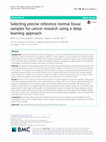
BMC Medical Genomics
Background: Normal tissue samples are often employed as a control for understanding disease mecha... more Background: Normal tissue samples are often employed as a control for understanding disease mechanisms, however, collecting matched normal tissues from patients is difficult in many instances. In cancer research, for example, the open cancer resources such as TCGA and TARGET do not provide matched tissue samples for every cancer or cancer subtype. The recent GTEx project has profiled samples from healthy individuals, providing an excellent resource for this field, yet the feasibility of using GTEx samples as the reference remains unanswered. Methods: We analyze RNA-Seq data processed from the same computational pipeline and systematically evaluate GTEx as a potential reference resource. We use those cancers that have adjacent normal tissues in TCGA as a benchmark for the evaluation. To correlate tumor samples and normal samples, we explore top varying genes, reduced features from principal component analysis, and encoded features from an autoencoder neural network. We first evaluate whether these methods can identify the correct tissue of origin from GTEx for a given cancer and then seek to answer whether disease expression signatures are consistent between those derived from TCGA and from GTEx. Results: Among 32 TCGA cancers, 18 cancers have less than 10 matched adjacent normal tissue samples. Among three methods, autoencoder performed the best in predicting tissue of origin, with 12 of 14 cancers correctly predicted. The reason for misclassification of two cancers is that none of normal samples from GTEx correlate well with any tumor samples in these cancers. This suggests that GTEx has matched tissues for the majority cancers, but not all. While using autoencoder to select proper normal samples for disease signature creation, we found that disease signatures derived from normal samples selected via an autoencoder from GTEx are consistent with those derived from adjacent samples from TCGA in many cases. Interestingly, choosing top 50 mostly correlated samples regardless of tissue type performed reasonably well or even better in some cancers. Conclusions: Our findings demonstrate that samples from GTEx can serve as reference normal samples for cancers, especially those do not have available adjacent tissue samples. A deep-learning based approach holds promise to select proper normal samples.
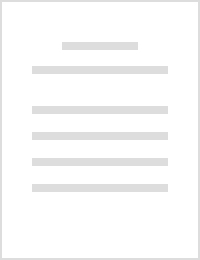
JAMIA Open
Objectives Electronic health record (EHR) data are increasingly used for biomedical discoveries. ... more Objectives Electronic health record (EHR) data are increasingly used for biomedical discoveries. The nature of the data, however, requires expertise in both data science and EHR structure. The Observational Medical Outcomes Partnership (OMOP) common data model (CDM) standardizes the language and structure of EHR data to promote interoperability of EHR data for research. While the OMOP CDM is valuable and more attuned to research purposes, it still requires extensive domain knowledge to utilize effectively, potentially limiting more widespread adoption of EHR data for research and quality improvement. Materials and methods We have created ROMOP: an R package for direct interfacing with EHR data in the OMOP CDM format. Results ROMOP streamlines typical EHR-related data processes. Its functions include exploration of data types, extraction and summarization of patient clinical and demographic data, and patient searches using any CDM vocabulary concept. Conclusion ROMOP is freely availa...
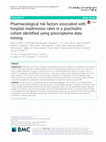
BMC medical informatics and decision making, Jan 14, 2018
Worldwide, over 14% of individuals hospitalized for psychiatric reasons have readmissions to hosp... more Worldwide, over 14% of individuals hospitalized for psychiatric reasons have readmissions to hospitals within 30 days after discharge. Predicting patients at risk and leveraging accelerated interventions can reduce the rates of early readmission, a negative clinical outcome (i.e., a treatment failure) that affects the quality of life of patient. To implement individualized interventions, it is necessary to predict those individuals at highest risk for 30-day readmission. In this study, our aim was to conduct a data-driven investigation to find the pharmacological factors influencing 30-day all-cause, intra- and interdepartmental readmissions after an index psychiatric admission, using the compendium of prescription data (prescriptome) from electronic medical records (EMR). The data scientists in the project received a deidentified database from the Mount Sinai Data Warehouse, which was used to perform all analyses. Data was stored in a secured MySQL database, normalized and indexed ...
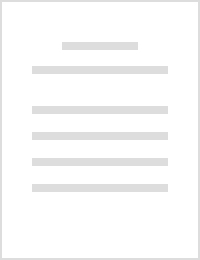
Journal of the American College of Cardiology, Jan 12, 2018
Artificial intelligence and machine learning are poised to influence nearly every aspect of the h... more Artificial intelligence and machine learning are poised to influence nearly every aspect of the human condition, and cardiology is not an exception to this trend. This paper provides a guide for clinicians on relevant aspects of artificial intelligence and machine learning, reviews selected applications of these methods in cardiology to date, and identifies how cardiovascular medicine could incorporate artificial intelligence in the future. In particular, the paper first reviews predictive modeling concepts relevant to cardiology such as feature selection and frequent pitfalls such as improper dichotomization. Second, it discusses common algorithms used in supervised learning and reviews selected applications in cardiology and related disciplines. Third, it describes the advent of deep learning and related methods collectively called unsupervised learning, provides contextual examples both in general medicine and in cardiovascular medicine, and then explains how these methods could ...
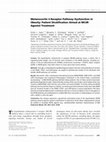
The Journal of clinical endocrinology and metabolism, Jan 2, 2018
The hypothalamic melanocortin 4 receptor (MC4R)-pathway serves a critical role in regulating body... more The hypothalamic melanocortin 4 receptor (MC4R)-pathway serves a critical role in regulating bodyweight. Loss of function (LoF) mutations in the MC4R pathway including mutations in the POMC (1), PCSK1, LEPR (2) or the MC4R genes (3) have been shown to be causative of early-onset severe obesity. Through a comprehensive epidemiological analysis of known and predicted LoF variants in the POMC, PCSK1 and LEPR genes, we sought to estimate the number of US individuals with bi-allelic MC4R pathway LoF variants. We predict approximately 650 α-MSH/POMC, 8,500 PCSK1 and 3,600 LEPR homozygous and compound heterozygous individuals in the US, cumulatively enumerating over 12,800 MC4R pathway deficient obese patients. Very few of these have been genetically diagnosed to date. These estimates increase when we include a small subset of less rare variants: β-MSH/POMC, PCSK1 N221D, and a novel PCSK1 LoF variant (T640A). To further define the MC4R pathway and its potential impact on obesity we tested ...
Uploads
Papers by Benjamin Glicksberg