Papers by Masoud Asgharian
arXiv (Cornell University), May 23, 2024
Research Square (Research Square), Apr 23, 2024
Annals of Operations Research, Jan 14, 2024
IEEE Transactions on Computer-Aided Design of Integrated Circuits and Systems, Dec 31, 2022
Findings of the Association for Computational Linguistics: EACL 2023

Bernoulli, Nov 1, 2017
Length-biased survival data commonly arise in cross-sectional surveys and prevalent cohort studie... more Length-biased survival data commonly arise in cross-sectional surveys and prevalent cohort studies on disease duration. Ignoring biased sampling leads to bias in estimating the hazard-of-failure and the survivaltime in the population. We address estimating the location of a possible change-point of an otherwise smooth hazard function when the collected data form a biased sample from the target population and the data are subject to informative censoring. We provide two estimation methodologies, for the location and size of the change-point, adapted to two scenarios of the truncation distribution: known and unknown. While the estimators in the first case show gain in efficiency as compared to those in the second case, the latter is more robust to the form of the truncation distribution. In both cases, the change-point estimators can achieve the rate O p (1/n). We study the asymptotic properties of the estimates and devise interval-estimators for the location and size of the change, paving the way towards making statistical inference about whether or not a change-point exists. Several simulated examples are discussed to assess the finite sample behavior of the estimators. The proposed methods are then applied to analyze a set of survival data collected on elderly Canadian citizen (aged 65+) suffering from dementia.
SN computer science, Jun 29, 2023

medRxiv (Cold Spring Harbor Laboratory), Jul 29, 2021
Traffic is one of the major contributors to PM2.5 in cities worldwide. Quantifying the role of tr... more Traffic is one of the major contributors to PM2.5 in cities worldwide. Quantifying the role of traffic is an important step towards understanding the impact of transport policies on the possibilities to achieve cleaner air and accompanying health benefits. We carried out a meta-analysis using the World Health Organisation (WHO) database of source apportionment studies of PM2.5 concentrations. Specifically, we used a Bayesian meta-regression approach, modelling both overall and traffic-related (tailpipe and non-tailpipe) concentrations simultaneously. We obtained the distributions of expected PM2.5 concentrations (posterior densities) of different types for 117 cities worldwide. For each city, we calculated the probabilities of exceeding the WHO-recommended concentration of PM2.5 if all of traffic emissions were removed. Using the non-linear Integrated Exposure Response (IER) function of PM2.5, we estimated percent reduction in different disease endpoints for a scenario with complete removal of traffic emissions. We found that this results in achieving the WHO-recommended concentration of PM2.5 only for a handful of cities that already have low concentrations of pollution. The percentage reduction in prevented mortality for cardiovascular and respiratory diseases increases up to a point (30-40 ug/m 3), and above this concentration, it flattens off. For Diabetesrelated mortality, the percentage reduction in mortality decreases with increasing concentrations-a trend that is opposite to other outcomes. For cities with high concentrations of pollution, the results highlight the need for multisectoral strategies to reduce pollution. The IER functions of PM2.5 result in diminishing returns of health benefits at high concentrations, and in case of Diabetes, there are even negative returns. The results show the significant effect of the shape of IER functions on health benefits. Overall, despite the diminishing results, a significant burden of deaths can be prevented by policies that aim to reduce traffic emissions even at high concentrations of pollution.

Shape is an important phenotype of living species that contain different environmental and geneti... more Shape is an important phenotype of living species that contain different environmental and genetic information. Clustering living cells using their shape information can provide a preliminary guide to their functionality and evolution. Hierarchical clustering and dendrograms, as a visualization tool for hierarchical clustering, are commonly used by practitioners for classification and clustering. The existing hierarchical shape clustering methods are distance based. Such methods often lack a proper statistical foundation to allow for making inference on important parameters such as the number of clusters, often of prime interest to practitioners. We take a model selection perspective to clustering and propose a shape clustering method through linear models defined on Spherical Harmonics expansions of shapes. We introduce a BIC-type criterion, called CLUSBIC, and study consistency of the criterion. Special attention is paid to the notions of over-and under-specified models, important in studying model selection criteria and naturally defined in model selection literature. These notions do not automatically extend to shape clustering when a model selection perspective is adopted for clustering. To this end we take a novel approach using hypothesis testing. We apply our proposed criterion to cluster a set of real 3D images from HeLa cell line.
Les Cahiers du GERAD, Apr 1, 2020
La collection Les Cahiers du GERAD est constituée des travaux de recherche menés par nos membres.... more La collection Les Cahiers du GERAD est constituée des travaux de recherche menés par nos membres. La plupart de ces documents de travail aété soumisà des revues avec comité de révision. Lorsqu'un document est accepté et publié, le pdf original est retiré si c'est nécessaire et un lien vers l'article publié est ajouté.

Wiley Interdisciplinary Reviews: Computational Statistics, Nov 10, 2020
In many applications of Bayesian clustering, posterior sampling on the discrete state space of cl... more In many applications of Bayesian clustering, posterior sampling on the discrete state space of cluster allocations is achieved via Markov chain Monte Carlo (MCMC) techniques. As it is typically challenging to design transition kernels to explore this state space efficiently, MCMC convergence diagnostics for clustering applications is especially important. For general MCMC problems, state-of-the-art convergence diagnostics involve comparisons across multiple chains. However, single-chain alternatives can be appealing for computationally intensive and slowly-mixing MCMC, as is typically the case for Bayesian clustering. Thus, we propose here a single-chain convergence diagnostic specifically tailored to discrete-space MCMC. Namely, we consider a Hotelling-type statistic on the highest probability states, and use regenerative sampling theory to derive its equilibrium distribution. By leveraging information from the unnormalized posterior, our diagnostic protects against seemingly convergent chains in which the relative frequency of visited states is incorrect. The methodology is illustrated with a Bayesian clustering analysis of genetic mutants of the flowering plant Arabidopsis thaliana.
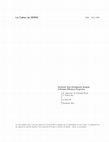
Les Cahiers du GERAD, Dec 1, 2013
Since its inception, stochastic Data Envelopment Analysis (DEA) has found many applications. The ... more Since its inception, stochastic Data Envelopment Analysis (DEA) has found many applications. The approach commonly taken in stochastic DEA is via chance constraint models. This approach cannot, however, capture the inherent random fluctuations of the efficiency score caused by the random nature of the input and output variables. Having taken a random efficiency perspective, one can introduce an alternative approach that provides ground for capturing these fluctuations. One aspect of this alternative approach, which seems to have been neglected, concerns the distribution of the random efficiency score. We show that the efficiency score does not have a continuous distribution even if the random input and output variables distributions are continuous. The efficiency score distribution has, in fact, a point mass decomposition at 1. This observation renders the non-parametric bootstrap of efficiency score impossible. We introduce several criteria for the ranking and classification of Decision Making Units (DMUs) using a random efficiency perspective, including an interactive ranking method that incorporates managers' knowledge and preferences. We then apply the point mass decomposition of efficiency score distributions of DMUs and show how these criteria can be implemented. We also discuss how one may estimate the efficiency score distributions of DMUs using both Bayesian and frequentist approaches. Our proposed methodology is illustrated using a real data set.

Stat, Mar 1, 2014
The pervasive use of prevalent cohort studies on disease duration increasingly calls for an appro... more The pervasive use of prevalent cohort studies on disease duration increasingly calls for an appropriate methodology to account for the biases that invariably accompany samples formed by such data. It is well known, for example, that subjects with shorter lifetime are less likely to be present in such studies. Moreover, certain covariate values could be preferentially selected into the sample, being linked to the long-term survivors. The existing methodology for estimating the propensity score using data collected on prevalent cases requires the correct conditional survival/hazard function given the treatment and covariates. This requirement can be alleviated if the disease under study has stationary incidence, the so-called stationarity assumption. We propose a non-parametric adjustment technique based on a weighted estimating equation for estimating the propensity score, which does not require modeling the conditional survival/hazard function when the stationarity assumption holds. The estimator's large-sample properties are established, and its small-sample behavior is studied via simulation. The estimated propensity score is utilized to estimate the survival curves.

Research Square (Research Square), Nov 28, 2022
Nesterov's accelerated gradient (AG) is a popular technique to optimize objective functions compr... more Nesterov's accelerated gradient (AG) is a popular technique to optimize objective functions comprising two components: a convex loss and a penalty function. While AG methods perform well for convex penalties, such as the LASSO, convergence issues may arise when it is applied to nonconvex penalties, such as SCAD. A recent proposal generalizes Nesterov's AG method to the nonconvex setting. The proposed algorithm requires specification of several hyperparameters for its practical application. Aside from some general conditions, there is no explicit rule for selecting the hyperparameters, and how different selection can affect convergence of the algorithm. In this article, we propose a hyperparameter setting based on the complexity upper bound to accelerate convergence, and consider the application of this nonconvex AG algorithm to high-dimensional linear and logistic sparse learning problems. We further establish the rate of convergence and present a simple and useful bound to characterize our proposed optimal damping sequence. Simulation studies show that convergence can be made, on average, considerably faster than that of the conventional proximal gradient algorithm. Our Accelerated Gradient Methods for Sparse Statistical Learning with Nonconvex Penaltie experiments also show that the proposed method generally outperforms the current state-of-the-art methods in terms of signal recovery.
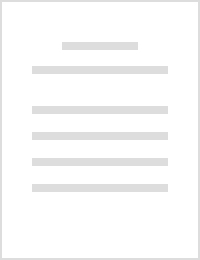
Theory of Probability and Its Applications, 2014
Nonsingularity of the information matrix plays a key role in model identification and the asympto... more Nonsingularity of the information matrix plays a key role in model identification and the asymptotic theory of statistics. For many statistical models, however, this condition seems virtually impossible to verify. An example of such models is a class of mixture models associated with multipath change-point problems (MCPs). The question then arises as to how often this assumption fails to hold. Using the subimmersion theorem and upper semicontinuity of the spectrum, we show that the set of singularities of the information matrix is a nowhere dense set, i.e., geometrically negligible, if the model is identifiable and some mild smoothness conditions hold. Under further smoothness conditions we show that the set is also of measure zero, i.e., both geometrically and analytically negligible. In view of our main results, we further study a flexible class of MCP models, thus paving the way for establishing asymptotic normality of the maximum likelihood estimates (MLEs) and statistical inference of the unknown par...
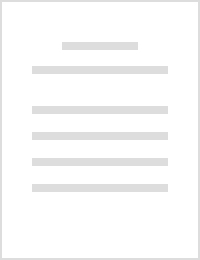
Expert Systems With Applications, Sep 1, 2021
Abstract The cross-efficiency (CE) evaluation method was introduced to improve the discriminatory... more Abstract The cross-efficiency (CE) evaluation method was introduced to improve the discriminatory power of DEA and eliminate unrealistic DEA weighting schemes. One important issue in CE evaluation is the non-uniqueness of the CE scores. Several secondary goal models based on different targets for cross-efficiencies (CEs) of each DMU with respect to other DMUs were proposed to address this issue. However, the suggested targets, fixed value 1 and the CCR efficiency score, are not achievable for all CEs. Moreover, the proposed secondary goal models based on these targets are sensitive to outlier DMUs, and may generate unrealistic CE scores. In this manuscript, we prove that the spectrum of achievable targets of CEs can be obtained using the most resonated appreciative (MRA) model, proposed by Oral et al. (2015), and the least resonated appreciative (LRA) model that we introduce. To this end, we propose a general secondary goal model using multi-objective programming and show that the CEs generated using MRA (LRA) model for each DMU is greater (less) than the corresponding CEs obtained by any other model that can be derived from the proposed benevolent (aggressive) general model. Using this achievable spectrum, we then propose several benevolent, aggressive and neutral secondary goal, and a weighted average CE evaluation model. Using two real examples, we compare the results of the proposed CE methods with those obtained from several other CE methods. Our data analyses indicate that our proposed methods are less sensitive to outliers, less biased towards one, has better discriminatory power and can identify pseudo-efficient DMUs.
Biometrika, Jul 24, 2012
Incidence is an important epidemiological concept most suitably studied using an incident cohort ... more Incidence is an important epidemiological concept most suitably studied using an incident cohort study. However, data are often collected from the more feasible prevalent cohort study, whereby diseased individuals are recruited through a cross-sectional survey and followed in time. In the absence of temporal trends in survival, we derive an efficient nonparametric estimator of the cumulative incidence based on such data and study its asymptotic properties. Arbitrary calendar time variations in disease incidence are allowed. Age-specific incidence and adjustments for both stratified sampling and temporal variations in survival are also discussed. Simulation results are presented and data from the Canadian Study of Health and Aging are analysed to infer the incidence of dementia in the Canadian elderly population.

arXiv (Cornell University), Sep 22, 2020
Nesterov's accelerated gradient (AG) is a popular technique to optimize objective functions compr... more Nesterov's accelerated gradient (AG) is a popular technique to optimize objective functions comprising two components: a convex loss and a penalty function. While AG methods perform well for convex penalties, such as the LASSO, convergence issues may arise when it is applied to nonconvex penalties, such as SCAD. A recent proposal generalizes Nesterov's AG method to the nonconvex setting. The proposed algorithm requires specification of several hyperparameters for its practical application. Aside from some general conditions, there is no explicit rule for selecting the hyperparameters, and how different selection can affect convergence of the algorithm. In this article, we propose a hyperparameter setting based on the complexity upper bound to accelerate convergence, and consider the application of this nonconvex AG algorithm to high-dimensional linear and logistic sparse learning problems. We further establish the rate of convergence and present a simple and useful bound to characterize our proposed optimal damping sequence. Simulation studies show that convergence can be made, on average, considerably faster than that of the conventional proximal gradient algorithm. Our experiments also show that the proposed method generally outperforms the current state-of-the-art methods in terms of signal recovery.

The International Journal of Biostatistics, 2015
Length bias in survival data occurs in observational studies when, for example, subjects with sho... more Length bias in survival data occurs in observational studies when, for example, subjects with shorter lifetimes are less likely to be present in the recorded data. In this paper, we consider estimating the causal exposure (treatment) effect on survival time from observational data when, in addition to the lack of randomization and consequent potential for confounding, the data constitute a length-biased sample; we hence term this a double-bias problem. We develop estimating equations that can be used to estimate the causal effect indexing the structural Cox proportional hazard and accelerated failure time models for point exposures in double-bias settings. The approaches rely on propensity score-based adjustments, and we demonstrate that estimation of the propensity score must be adjusted to acknowledge the length-biased sampling. Large sample properties of the estimators are established and their small sample behavior is studied using simulations. We apply the proposed methods to a set of, partly synthesized, length-biased survival data collected as part of the Canadian Study of Health and Aging (CSHA) to compare survival of subjects with dementia among institutionalized patients versus those recruited from the community and depict their adjusted survival curves.
Often, in cross-sectional-follow-up studies, survival data are obtained from prevalent cases only... more Often, in cross-sectional-follow-up studies, survival data are obtained from prevalent cases only. This sampling mechanism introduces lenght-bias. An added difficulty is that in some cases the times of the onset cannot be ascertained or are recorded with great uncertainty. Such was the situation in the Canadian Study of Health and Aging (CSHA), an ongoing nationwide study of dementia conducted by Health Canada. This paper proposes methods to estimate the survival function nonparametrically, when the data are length-biased and only partially observed. By using the "forward recurrence times" only, we suggest how one can overcome the difficulty caused by missing onset times, while by using the "backward recurrence times" only, one can avoid the cost and effort of follow-up. We illustrate our methods through an application to data derived from the CSHA.
Uploads
Papers by Masoud Asgharian