Papers by Vladimir Katkovnik
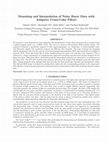
We propose a novel approach for joint denoising and interpolation of noisy Bayer-patterned data a... more We propose a novel approach for joint denoising and interpolation of noisy Bayer-patterned data acquired from a digital imaging sensor (e.g., CMOS, CCD). The aim is to obtain a full-resolution RGB noiseless image. The proposed technique is speciÞcally targeted to Þlter signal-dependant, e.g. Poissonian, or heteroscedastic noise, and effectively exploits the correlation between the different color channels. The joint technique for denoising and interpolation is based on the concept of local polynomial approximation (LPA) and intersection of conÞdence intervals (ICI). These directional Þlters utilize simultaneously the green, red, and blue color channels. This is achieved by a linear combination of complementary-supported smoothing and derivative kernels designed for the Bayer data grid. With these Þlters, the denoised and the interpolated estimates are obtained by convolutions over the Bayer data. The ICI rule is used for data-adaptive selection of the length of the designed cross-color directional Þlter. Fusing estimates from multiple directions provides the Þnal anisotropic denoised and interpolated values. The full-size RGB image is obtained by placing these values into the corresponding positions in the image grid. The efficiency of the proposed approach is demonstrated by experimental results with simulated and real camera data.
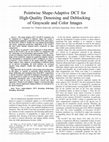
IEEE Transactions on Image Processing, 2007
The shape-adaptive DCT (SA-DCT) transform can be computed on a support of arbitrary shape, but re... more The shape-adaptive DCT (SA-DCT) transform can be computed on a support of arbitrary shape, but retains a computational complexity comparable to that of the usual separable block-DCT (B-DCT). Despite the near-optimal decorrelation and energy compaction properties, application of the SA-DCT has been rather limited, targeted nearly exclusively to video compression. In this paper we present a novel approach to image Þltering based on the SA-DCT. We use the SA-DCT in conjunction with the Anisotropic Local Polynomial Approximation (LPA)-Intersection of ConÞdence Intervals (ICI) technique, which deÞnes the shape of the transform's support in a pointwise adaptive manner. The thresholded or attenuated SA-DCT coefÞcients are used to reconstruct a local estimate of the signal within the adaptiveshape support. Since supports corresponding to different points are in general overlapping, the local estimates are averaged together using adaptive weights that depend on the region's statistics. This approach can be used for various image processing tasks. In this paper we consider in particular image denoising and image deblocking and deringing from block-DCT compression. A special structural constraint in luminance-chrominance space is also proposed to enable an accurate Þltering of color images. Simulation experiments show a state-of-the-art quality of the Þnal estimate, both in terms of objective criteria and visual appearance. Thanks to the adaptive support, reconstructed edges are clean, and no unpleasant ringing artifacts are introduced by the Þtted transform.
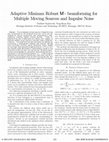
Proceedings of the 2001 IEEE Radar Conference (Cat. No.01CH37200)
The windowed multiple source M-beamforming and M-estimates of the direction-of-arrival (DOA) are ... more The windowed multiple source M-beamforming and M-estimates of the direction-of-arrival (DOA) are developed for source visualization and nonparametric highresolution tracking rapidly moving sources. These Mbeamforming and M-estimates are robust with respect to impulse random errors having a unknown heavy-tailed distribution. The beamforming and estimates are defined by minimizing a nonquadratic residual loss function derived from the Huber minimax robust estimation theory. Based on the linear local polynomial approximation (LP A) of DOA the method gives estimates of instantaneous values of the directions as well as their first derivatives. Marginal beamformers are proposed for estimation and moving source visualization in two-dimensional space θ −θ (1). These marginal beamformers are able to localize and track every source individually nulling signals from all other moving sources. Recursive implementation of estimation algorithms are developed.
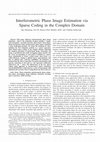
IEEE Transactions on Geoscience and Remote Sensing, 2015
This paper addresses interferometric phase image estimation-that is, the estimation of phase modu... more This paper addresses interferometric phase image estimation-that is, the estimation of phase modulo-2π images from sinusoidal 2π-periodic and noisy observations. These degradation mechanisms make interferometric phase image estimation a quite challenging problem. We tackle this challenge by reformulating the true estimation problem as a sparse regression, often termed sparse coding, in the complex domain. Following the standard procedure in patch-based image restoration, the image is partitioned into small overlapping square patches and the vector corresponding to each patch is modeled as a sparse linear combination of vectors, termed atoms, taken from a set called dictionary. Aiming at optimal sparse representations, and thus at optimal noise removing capabilities, the dictionary is learned from the data it represents via matrix factorization with sparsity constraints on the code (i.e., the regression coefficients) enforced by the ℓ1 norm. The effectiveness of the new sparse coding based approach to interferometric phase estimation, termed SpInPHASE, is illustrated in a series of experiments with simulated and real data where it outperforms the state-of-the-art.
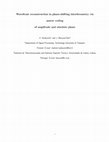
Journal of the Optical Society of America. A, Optics, image science, and vision, 2014
Phase-shifting interferometry is a coherent optical method that combines high accuracy with high ... more Phase-shifting interferometry is a coherent optical method that combines high accuracy with high measurement speeds. This technique is therefore desirable in many applications such as the efficient industrial quality inspection process. However, despite its advantageous properties, the inference of the object amplitude and the phase, herein termed wavefront reconstruction, is not a trivial task owing to the Poissonian noise associated with the measurement process and to the 2π phase periodicity of the observation mechanism. In this paper, we formulate the wavefront reconstruction as an inverse problem, where the amplitude and the absolute phase are assumed to admit sparse linear representations in suitable sparsifying transforms (dictionaries). Sparse modeling is a form of regularization of inverse problems which, in the case of the absolute phase, is not available to the conventional wavefront reconstruction techniques, as only interferometric phase modulo-2π is considered therein....
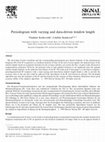
Signal Processing, 1998
The short-time Fourier transform and the corresponding periodogram give biased estimates of the i... more The short-time Fourier transform and the corresponding periodogram give biased estimates of the instantaneous frequency (IF) if the IF in question is a nonlinear function of time. In the case of noisy signals, the optimal choice of the window length, based on asymptotic formulae for the variance and bias, can resolve the bias-variance trade-off usual for nonparametric estimation. However, the practical value of such optimal estimator is not significant since the optimal window length depends on the unknown smoothness of the IF. The main goal of this paper is to develop an adaptive, periodogram-based IF estimator with a time-varying and data-driven window length which is able to provide the accuracy close to the one that could be achieved if the smoothness of the IF were known in advance. The developed algorithm uses only the estimates of the IF and the formula for the variance of these estimates. Simulation shows good accuracy ability of the adaptive algorithm. 1998 Elsevier Science B.V. All rights reserved. Zusammenfassung Die Kurzzeit-Fouriertransformation und das entsprechende Periodogramm liefern biasbehaftete Scha¨tzer der Momentanfrequenz (IF), wenn diese eine nichtlineare Funktion der Zeit ist. Bei verrauschten Signalen kann eine optimale Wahl der Fensterla¨nge, basierend auf einer asymptotischen Formulierung fu¨r Varianz und Bias, dem u¨blichen Bias-Varianz Verlust bei nicht-parametrischen Scha¨tzungen begegnen. Der praktische Wert eines solchen Optimalscha¨tzers ist allerdings nicht signifikant, solange die optimale Fensterla¨nge von der unbekannten Gla¨tte der Momentanfrequenz (IF) abha¨ngig ist. Ziel dieses Papiers ist es nun, einen adaptiven, auf Periodogrammen basierenden Scha¨tzer fu¨r die Momentanfrequenz zu entwickeln, wobei eine zeitvariierende und datenabha¨ngige Fensterla¨nge betrachtet wird. Mit diesem Scha¨tzer ist man in der Lage, Aussagen u¨ber die Genauigkeit der Scha¨tzung zu machen, und zwar in a¨hnlicher Weise, wie es bei Kenntnis der Gla¨tte der IF mo¨glich wa¨re. Der hier entwickelte Algorithmus benutzt nur die Scha¨tzungen der IF und einen Ausdruck fu¨r die Varianz dieser Scha¨tzungen. Simulationen zeigen die guten Eigenschaften des adaptiven Algorithmus in Bezug auf die Scha¨tzgenauigkeit.
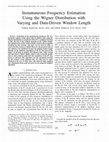
IEEE Transactions on Signal Processing, 1998
Estimation of the instantaneous frequency (IF) of a harmonic complex-valued signal with an additi... more Estimation of the instantaneous frequency (IF) of a harmonic complex-valued signal with an additive noise using the Wigner distribution is considered. If the IF is a nonlinear function of time, the bias of the estimate depends on the window length. The optimal choice of the window length, based on the asymptotic formulae for the variance and bias, can be used in order to resolve the bias-variance tradeoff. However, the practical value of this solution is not significant because the optimal window length depends on the unknown smoothness of the IF. The goal of this paper is to develop an adaptive IF estimator with a time-varying and data-driven window length, which is able to provide quality close to what could be achieved if the smoothness of the IF were known in advance. The algorithm uses the asymptotic formula for the variance of the estimator only. Its value may be easily obtained in the case of white noise and relatively high sampling rate. Simulation shows good accuracy for the proposed adaptive algorithm. I. INTRODUCTION A complex-valued harmonic with a time-varying phase is a key model of the instantaneous frequency (IF) concept, as well as an important model in the general theory of time-frequency distributions. It has been utilized for the study of a wide range of signals, including speech, music, biological, radar, sonar, and geophysical ones [14]. An overview of methods for the IF estimation, as well as an interpretation of the IF concept itself, is presented in [2] and [6]. Beside other efficient techniques for the IF estimation (e.g., [2], [11], [15], [16]), the time-frequency distribution approach is interesting and commonly applied [2], [6]. This approach is based on the property of time-frequency distributions to concentrate the energy of a signal, in the time-frequency plane, at and around the IF [1], [2], [4], [5], [12]. Out of the general Cohen class of time-frequency distributions with a signalindependent kernel, the Wigner distribution (WD) produces the best concentration along the linear IF [4], [5], [19], [20].
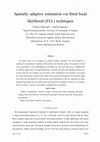
IEEE Transactions on Signal Processing, 2008
This paper offers a new technique for spatially adaptive estimation. The local likelihood is expl... more This paper offers a new technique for spatially adaptive estimation. The local likelihood is exploited for nonparametric modeling of observations and estimated signals. The approach is based on the assumption of a local homogeneity of the signal: for every point there exists a neighborhood in which the signal can be well approximated by a constant. The fitted local likelihood statistics are used for selection of an adaptive size and shape of this neighborhood. The algorithm is developed for a quite general class of observations subject to the exponential distribution. The estimated signal can be uni-and multivariable. We demonstrate a good performance of the new algorithm for image denoising and compare the new method versus the intersection of confidence interval (ICI) technique that also exploits a selection of an adaptive neighborhood for estimation.
The deconvolution in image processing is an inverse illposed problem which necessitates a trade-o... more The deconvolution in image processing is an inverse illposed problem which necessitates a trade-off betweendelity to data and smoothness of a solution adjusted by a regularization parameter. In this paper we propose two techniques for selection of a varying regularization parameter minimizing the mean squared error for every pixel of the image. The rst algorithm uses the estimate of the squared point-wise bias of the regularized inverse. The second algorithm is based on direct multiple statistical hypothesis testing for the estimates calculated with different regularization parameters. The simulation results on images illustrate the ef ciency of the proposed technique.
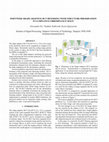
The shape-adaptive DCT (SA-DCT) [12, 13] is a low-complexity transform which can be computed on a... more The shape-adaptive DCT (SA-DCT) [12, 13] is a low-complexity transform which can be computed on a support of arbitrary shape. Particularly suited for coding image patches in the vicinity of edges, the SA-DCT has been included in the MPEG-4 standard [7]. The use of this shape-adaptive transform for grayscale image denoising has been recently proposed [3, 2], showing a remarkable performance. In this paper we extend this approach to color Þltering in luminance-chrominance space, exploiting the structural information obtained from the luminance channel to drive the shape-adaptation for the chrominance channels. Several simulation experiments attest the advanced performance of the proposed color denoising method. The visual quality of the estimates is high, with sharp detail preservation, clean edges, and without unpleasant ringing artifacts introduced by the Þtted transform. Besides noise removal, the proposed method is also effective in dealing with those artifacts which are often encountered in compressed images and videos. Blocking artifacts are suppressed while salient image features are preserved. The SA-DCT Þltering used for the chrominance channels allows to faithfully reconstruct the missing structural information of the chrominances, thus correcting colorbleeding artifacts. Being based on the SA-DCT (which is implemented as standard in modern MPEG hardware), the proposed method can be integrated within existing video platforms as a pre-or post-processing Þlter.
Robust M-periodogram is defined for the analysis of signals with heavy-tailed distribution noise.... more Robust M-periodogram is defined for the analysis of signals with heavy-tailed distribution noise. In the form of a robust spectrogram it can be used for the analysis of nonstationary signals. In this paper a robust spectrogram based instantaneous frequency (IF) estimator, with a time-varying window length, is presented. The optimal choice of the window length, based on asymptotic formulae for the variance and bias, can resolve the bias-variance trade-off in the robust spectrogram based IF estimation. However, it depends on the unknown nonlinearity of the IF. The algorithm used in this paper is able to provide the accuracy close to the one that could be achieved if the IF, to be estimated, were known in advance. Simulations show good accuracy ability of the adaptive algorithm and good robustness properties with respect to rare high magnitude noise values.
This paper offers a new technique for spatially adaptive estimation. The local likelihood is expl... more This paper offers a new technique for spatially adaptive estimation. The local likelihood is exploited for nonparametric modelling of observations and estimated signals. The approach is based on the assumption of a local homogeneity of the signal: for every point there exists a neighborhood in which the signal can be well approximated by a constant. The fitted local likelihood statistics is used for selection of an adap-tive size of this neighborhood. The algorithm is developed for quite a general class of observations subject to the exponential distribution. The estimated signal can be uni-and multivariable. We demonstrate a good performance of the new algorithm for Poissonian image denoising and compare of the new method versus the intersection of confidence interval (ICI) technique that also exploits a selection of an adaptive neighborhood for estimation.
We outline the evolution of the nonparametric regression modelling in imaging from the local Nada... more We outline the evolution of the nonparametric regression modelling in imaging from the local Nadaraya-Watson estimates to the nonlocal means and further to the latest nonlocal block-matching techniques based on transform-domain Þltering. The considered methods are classiÞed mainly according to two leading features: local/nonlocal and pointwise/multipoint. Here nonlocal is an alternative to local, and multipoint is alternative to pointwise. The alternatives, though an obvious simpliÞcation, allow to impose a fruitful and transparent classiÞcation of the basic ideas in the advanced techniques. Within this framework, we introduce a novel multiplemodel interpretation of the basic modelling used in the BM3D algorithm [11], highlighting a source of the outstanding performance of this type of algorithms.
Proceedings of the 2000 IEEE Sensor Array and Multichannel Signal Processing Workshop. SAM 2000 (Cat. No.00EX410)
A windowed local polynomial approximation (LPA) of time-varying directions-of-arrival (DOA) is ex... more A windowed local polynomial approximation (LPA) of time-varying directions-of-arrival (DOA) is exploited to derive a new form of beamformer for source localization and tracking in highly nonstationary environments. The relationship between our LPA-beamformer and the conventional beamformer is derived. Closed-form expressions are obtained for the bias and variance of the LPA-beamformer in the nonstationary case and the problem of optimal
Publication in the conference proceedings of EUSIPCO, Lausanne, Switzerland, 2008
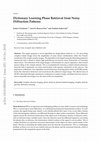
This paper proposes a novel algorithm for image phase retrieval, i.e., for recovering complex-val... more This paper proposes a novel algorithm for image phase retrieval, i.e., for recovering complex-valued images from the amplitudes of noisy linear combinations (often the Fourier transform) of the sought complex images. The algorithm is developed using the alternating projection framework and is aimed to obtain high performance for heavily noisy (Poissonian or Gaussian) observations. The estimation of the target images is reformulated as a sparse regression, often termed sparse coding, in the complex domain. This is accomplished by learning a complex domain dictionary from the data it represents via matrix factorization with sparsity constraints on the code (i.e., the regression coefficients). Our algorithm, termed dictionary learning phase retrieval (DLPR), jointly learns the referred to dictionary and reconstructs the unknown target image. The effectiveness of DLPR is illustrated through experiments conducted on complex images, simulated and real, where it shows noticeable advantages...
Publication in the conference proceedings of EUSIPCO, Kos island, Greece, 2017
Publication in the conference proceedings of EUSIPCO, Tampere, Finland, 2000
2018 26th European Signal Processing Conference (EUSIPCO), 2018
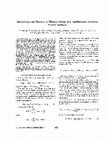
1998 IEEE International Joint Conference on Neural Networks Proceedings. IEEE World Congress on Computational Intelligence (Cat. No.98CH36227)
The number of hidden nodes is a crucial parameter of a feedforward artificial neural network. A n... more The number of hidden nodes is a crucial parameter of a feedforward artificial neural network. A neural network with too many nodes may overfit the data, causing poor generalization on d a t a not used for training, while too few hidden units underfit the model, and is not sufficiently accurate. The mean square error between the estimated network and a target function has a minimum with respect t o the number of nodes in the hidden layer, and is used t o measure t h e accuracy. In this paper a n algorithm is developed which optimizes the number of hidden nodes by minimizing the mean square error over noisy training data. The algorithm combines training sessions with statistical analyses and experimental design t o generate new sessions. Simulations show t h a t t h e developed algorithm requires fewer sessions t o establish the optimal number of hidden nodes than using the straightforward way of eliminating nodes successively one by one.
Uploads
Papers by Vladimir Katkovnik