Papers by Seiichi Nakamori

In the conventional estimation problem, we assume that the observed value always contains a signa... more In the conventional estimation problem, we assume that the observed value always contains a signal to be estimated and observation noise. However, in communication systems, there arises the case where the observed value consists of noise alone during observing the signal. This paper, in the estimation problem with the uncertain observation, designs algorithms for fixed-point smoothing and filtering estimates by use of a probability that the signal exists, a crosscovariance function of the signal with the observed value, an autocovariance function of the signal plus colored noise besides the observed value when the signal is observed with additional white Gaussian and colored noises. The signal might be correlated with colored noise. In the current approach, it is advantageous over the existing one阜n that the present estimator necessitates the covariance information without requiring complete knowledge of state-space model of the signal. A numerical simulation example is shown to dem...

Frontiers in Signal Processing
This paper proposes the robust recursive least-squares (RLS) Wiener fixed-point smoother and filt... more This paper proposes the robust recursive least-squares (RLS) Wiener fixed-point smoother and filter in linear discrete-time stochastic systems with parameter uncertainties. The uncertain parameters exist in the observation matrix and the system matrix. The uncertain parameters cause to generate the degraded signal. In this paper, the degraded signal process is fitted to the finite order autoregressive (AR) model. The robust RLS Wiener estimators use the system matrices and the observation matrices for both the signal and the degraded signal, the variance of the state vector for the degraded signal, the cross-variance of the state vector for the signal with the state vector for the degraded signal, and the variance of the white observation noise. Also, this paper proposes the robust recursive fixed-point smoother and filter, by using the covariance information of the state vector for the degraded signal, the cross-covariance information of the state vector for the signal with the state vector for the degraded signal, the observation matrices for both the degraded signal and the signal besides the variance of the white observation noise. In estimating the signal process expressed by the second order AR model, the proposed robust RLS Wiener filter is superior in estimation accuracy to the robust Kalman filter and the RLS Wiener filter.
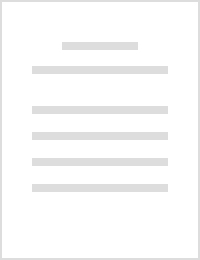
Jordan Journal of Electrical Engineering
In the centralized robust multi-sensor recursive least-square (RLS) Wiener filtering algorithm, t... more In the centralized robust multi-sensor recursive least-square (RLS) Wiener filtering algorithm, the number of recursive equations increases compared to that of the centralized multi-sensor RLS Wiener filter in linear discrete-time stationary stochastic systems with uncertain parameters. Due to the increase in the number of recursive Riccati-type algebraic equations, the accumulation of round-off errors is not negligible. The round-off errors cause unstable numerical characteristics of the filter, especially for the small variance of the observation noise. To reduce the round-off errors-as the first attempt in the research field of centralized robust multi-sensor estimation-this paper designs the Chandrasekhar-type centralized robust multi-sensor RLS Wiener filter, which updates the filter gains recursively. To verify the effectiveness of the proposed filter, a numerical simulation example is demonstrated and its estimation accuracy is compared with the centralized robust multi-sensor RLS Wiener filter and the centralized multi-sensor RLS-Wiener filter. The obtained results show that the proposed filter exhibits better stability.

Frontiers in Signal Processing
This paper proposes the robust recursive least-squares (RLS) finite impulse response (FIR) filter... more This paper proposes the robust recursive least-squares (RLS) finite impulse response (FIR) filtering algorithm using the covariance information and the robust RLS Wiener FIR filtering algorithm in linear discrete-time stochastic systems with the parameter uncertainties. The observation and system matrices contain the uncertain parameters. The uncertain parameters cause the degraded signal. Theorem 2 proposes the robust RLS FIR filter using the covariance information of the state vector for the degraded signal, the cross-covariance information of the state vector for the signal with the state vector for the degraded signal, the observation matrices for the signal and the degraded signal, and the variance of the white observation noise. Here, it is assumed that the signal and the degraded signal are fitted to the finite-order autoregressive (AR) models. Theorem 3 proposes the robust RLS Wiener FIR filter. The robust RLS Wiener FIR filtering algorithm uses the system and observation matrices for the signal and the degraded signal, the variance of the state vector for the degraded signal, the cross-variance function of the state vector for the signal with the state vector for the degraded signal, and the variance of the white observation noise.
IFAC Proceedings Volumes
... Aurora Hermoso-Carazo**, Jos'e Domingo Jim'enez-L'opez*** and Josefa Linarez-P... more ... Aurora Hermoso-Carazo**, Jos'e Domingo Jim'enez-L'opez*** and Josefa Linarez-P'erez** * Department of Technology, Faculty of Education, Kagoshima University **Departamento de Estadística e Investigaci'on Operativa, Universidad de Granada * * ^Departamento de ...

Journal of Signal and Information Processing
Almost estimators are designed for the white observation noise. In the estimation problems, rathe... more Almost estimators are designed for the white observation noise. In the estimation problems, rather than the white observation noise, there might be actual cases where the observation noise is modeled by the colored noise process. This paper examines to design a new estimation technique of recursive least-squares (RLS) Wiener fixed-point smoother and filter for colored observation noise in linear discrete-time wide-sense stationary stochastic systems. The observation y k is given as the sum of the signal z k Hx k and the colored observation noise. The RLS Wiener estimators explicitly require the following information: 1) the system matrix for the state vector c v k x k ; 2) the observation matrix H; 3) the variance of the state vector x k ; 4) the system matrix for the colored observation noise c v k ; 5) the variance of the colored observation noise; 6) the input noise variance in the state equation for the colored observation noise.
International Journal of Information Technology and Computer Science
Almost estimators are designed for the white observation noise. In the estimation problems, rathe... more Almost estimators are designed for the white observation noise. In the estimation problems, rather than the white observation noise, there might be actual cases where the observation noise is colored. This paper, from the viewpoint of the innovation theory, based on the recursive least-squares (RLS) Wiener fixed-point smoother and filter for the colored
International Journal of Information Technology and Computer Science

IFAC Proceedings Volumes
This paper addresses the QR decomposition and UD factorization based square-root algorithms of th... more This paper addresses the QR decomposition and UD factorization based square-root algorithms of the recursive least-squares (RLS) Wiener fixed-point smoother and filter. In the RLS Wiener estimators, the Riccati-type difference equations for the auto-variance function of the filtering estimate are included. Hence, by the roundoff errors, in the case of the small value of the observation noise variance, under a single precision computation, the auto-variance function becomes asymmetric and the estimators tend to be numerically instable. From this viewpoint, in the proposed square-root RLS Wiener estimators, in each stage updating the estimates, the auto-variance function of the filtering estimate is expressed in a symmetric positive semi-definite matrix and the stability of the RLS Wiener estimators is improved. In addition, in the squareroot RLS Wiener estimation algorithms, the variance function of the state prediction error is expressed as a symmetric positive semi-definite matrix in terms of the UD factorization method.
Mathematical and Computer Modelling
Ieice Transactions on Fundamentals of Electronics Communications and Computer Sciences, Dec 1, 2004
鹿児島大学教育学部研究紀要 自然科学篇, 2011
Ieice Transactions on Fundamentals of Electronics Communications and Computer Sciences, Mar 1, 2008
Applied Mathematics and Computation, Jul 1, 2004
In this paper recursive least mean-squared error quadratic filtering and fixed-point smoothing al... more In this paper recursive least mean-squared error quadratic filtering and fixed-point smoothing algorithms to estimate signals from uncertain observations are obtained for the case of white plus coloured observation noises. It is assumed that the state-space model of the signal is not known and only the information on the moments, up to the fourth one, of the involved processes and the probability that the signal exists in the observations are available. The estimators require the covariance functions of the signal and coloured noise, as well as the covariance functions of its second-order powers in a semi-degenerate kernel form.
鹿児島大学教育学部研究紀要 自然科学篇, 2009
鹿児島大学教育学部研究紀要 自然科学編, Mar 18, 2003
Ieice Transactions on Fundamentals of Electronics Communications and Computer, 2004
鹿児島大学教育学部研究紀要 自然科学編 Bulletin of the Faculty of Education Kagoshima University Natural Science, Mar 11, 2015
Uploads
Papers by Seiichi Nakamori