Papers by SUDIPTO BANERJEE
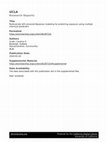
Environmetrics, 2018
Environmental health exposures to airborne chemicals often originate from chemical mixtures. Envi... more Environmental health exposures to airborne chemicals often originate from chemical mixtures. Environmental health professionals may be interested in assessing exposure to one or more of the chemicals in these mixtures, but often exposure measurement data are not available, either because measurements were not collected/assessed for all exposure scenarios of interest or because some of the measurements were below the analytical methods' limits of detection (i.e. censored). In some cases, based on chemical laws, two or more components may have linear relationships with one another, whether in a single or in multiple mixtures. Although bivariate analyses can be used if the correlation is high, often correlations are low. To serve this need, this paper develops a multivariate framework for assessing exposure using relationships of the chemicals present in these mixtures. This framework accounts for censored measurements in all chemicals, allowing us to develop unbiased exposure esti...
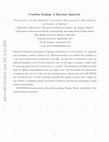
Annals of work exposures and health, Jan 23, 2018
Statistical interpolation of chemical concentrations at new locations is an important step in ass... more Statistical interpolation of chemical concentrations at new locations is an important step in assessing a worker's exposure level. When measurements are available from coastlines, as is the case in coastal clean-up operations in oil spills, one may need a mechanism to carry out spatial interpolation at new locations along the coast. In this article, we present a simple model for analyzing spatial data that is observed over a coastline. We demonstrate four different models using two different representations of the coast using curves. The four models were demonstrated on simulated data and one of them was also demonstrated on a dataset from the GuLF STUDY (Gulf Long-term Follow-up Study). Our contribution here is to offer practicing hygienists and exposure assessors with a simple and easy method to implement Bayesian hierarchical models for analyzing and interpolating coastal chemical concentrations.
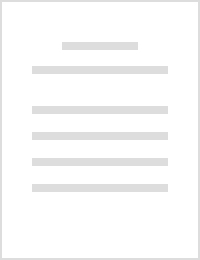
Journal of exposure science & environmental epidemiology, May 18, 2017
The GuLF STUDY is a cohort study investigating the health of workers who responded to the Deepwat... more The GuLF STUDY is a cohort study investigating the health of workers who responded to the Deepwater Horizon oil spill in the Gulf of Mexico in 2010. The objective of this effort was to develop an ordinal job-exposure matrix (JEM) of airborne total hydrocarbons (THC), dispersants, and particulates to estimate study participants' exposures. Information was collected on participants' spill-related tasks. A JEM of exposure groups (EGs) was developed from tasks and THC air measurements taken during and after the spill using relevant exposure determinants. THC arithmetic means were developed for the EGs, assigned ordinal values, and linked to the participants using determinants from the questionnaire. Different approaches were taken for combining exposures across EGs. EGs for dispersants and particulates were based on questionnaire responses. Considerable differences in THC exposure levels were found among EGs. Based on the maximum THC level participants experienced across any job...
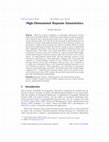
Bayesian Analysis
With the growing capabilities of Geographic Information Systems (GIS) and user-friendly software,... more With the growing capabilities of Geographic Information Systems (GIS) and user-friendly software, statisticians today routinely encounter geographically referenced data containing observations from a large number of spatial locations and time points. Over the last decade, hierarchical spatiotemporal process models have become widely deployed statistical tools for researchers to better understand the complex nature of spatial and temporal variability. However, fitting hierarchical spatiotemporal models often involves expensive matrix computations with complexity increasing in cubic order for the number of spatial locations and temporal points. This renders such models unfeasible for large data sets. This article offers a focused review of two methods for constructing well-defined highly scalable spatiotemporal stochastic processes. Both these processes can be used as "priors" for spatiotemporal random fields. The first approach constructs a lowrank process operating on a lower-dimensional subspace. The second approach constructs a Nearest-Neighbor Gaussian Process (NNGP) that ensures sparse precision matrices for its finite realizations. Both processes can be exploited as a scalable prior embedded within a rich hierarchical modeling framework to deliver full Bayesian inference. These approaches can be described as model-based solutions for big spatiotemporal datasets. The models ensure that the algorithmic complexity has ∼ n floating point operations (flops), where n the number of spatial locations (per iteration). We compare these methods and provide some insight into their methodological underpinnings.
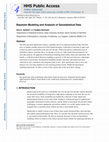
Annual Review of Statistics and Its Application
The most prevalent spatial data setting is, arguably, that of so-called geostatistical data, data... more The most prevalent spatial data setting is, arguably, that of so-called geostatistical data, data that arise as random variables observed at fixed spatial locations. Collection of such data in space and in time has grown enormously in the past two decades. With it has grown a substantial array of methods to analyze such data. Here, we attempt a review of a fully model-based perspective for such data analysis, the approach of hierarchical modeling fitted within a Bayesian framework. The benefit, as with hierarchical Bayesian modeling in general, is full and exact inference, with proper assessment of uncertainty. Geostatistical modeling includes univariate and multivariate data collection at sites, continuous and categorical data at sites, static and dynamic data at sites, and datasets over very large numbers of sites and long periods of time. Within the hierarchical modeling framework, we offer a review of the current state of the art in these settings. Keywords big spatial data; data assimilation; data fusion; Gaussian processes; integrated nested Laplace approximation; Markov chain Monte Carlo; multivariate spatial processes; spatiotemporal processes DISCLOSURE STATEMENT The authors are not aware of any affiliations, memberships, funding, or financial holdings that might be perceived as affecting the objectivity of this review.
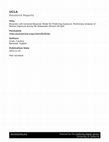
Annals of work exposures and health, 2017
In April 2010, the Deepwater Horizon oil rig caught fire and exploded, releasing almost 5 million... more In April 2010, the Deepwater Horizon oil rig caught fire and exploded, releasing almost 5 million barrels of oil into the Gulf of Mexico over the ensuing 3 months. Thousands of oil spill workers participated in the spill response and clean-up efforts. The GuLF STUDY being conducted by the National Institute of Environmental Health Sciences is an epidemiological study to investigate potential adverse health effects among these oil spill clean-up workers. Many volatile chemicals were released from the oil into the air, including total hydrocarbons (THC), which is a composite of the volatile components of oil including benzene, toluene, ethylbenzene, xylene, and hexane (BTEXH). Our goal is to estimate exposure levels to these toxic chemicals for groups of oil spill workers in the study (hereafter called exposure groups, EGs) with likely comparable exposure distributions. A large number of air measurements were collected, but many EGs are characterized by datasets with a large percentag...
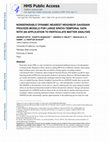
The Annals of Applied Statistics, 2016
Particulate matter (PM) is a class of malicious environmental pollutants known to be detrimental ... more Particulate matter (PM) is a class of malicious environmental pollutants known to be detrimental to human health. Regulatory efforts aimed at curbing PM levels in different countries often require high resolution space-time maps that can identify red-flag regions exceeding statutory concentration limits. Continuous spatio-temporal Gaussian Process (GP) models can deliver maps depicting predicted PM levels and quantify predictive uncertainty. However, GP-based approaches are usually thwarted by computational challenges posed by large datasets. We construct a novel class of scalable Dynamic Nearest Neighbor Gaussian Process (DNNGP) models that can provide a sparse approximation to any spatio-temporal GP (e.g., with nonseparable covariance structures). The DNNGP we develop here can be used as a sparsity-inducing prior for spatio-temporal random effects in any Bayesian hierarchical model to deliver full posterior inference. Storage and memory requirements for a DNNGP model are linear in the size of the dataset, thereby delivering massive scalability without sacrificing inferential richness. Extensive numerical studies reveal that the DNNGP provides substantially superior approximations to the underlying process than low-rank
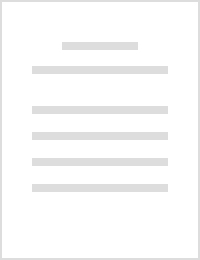
Wiley Interdisciplinary Reviews: Computational Statistics, 2016
Gaussian Process (GP) models provide a very flexible nonparametric approach to modeling location-... more Gaussian Process (GP) models provide a very flexible nonparametric approach to modeling location-and-time indexed datasets. However, the storage and computational requirements for GP models are infeasible for large spatial datasets. Nearest Neighbor Gaussian Processes (Datta A, Banerjee S, Finley AO, Gelfand AE. Hierarchical nearest-neighbor gaussian process models for large geostatistical datasets. 2016., JASA) provide a scalable alternative by using local information from few nearest neighbors. Scalability is achieved by using the neighbor sets in a conditional specification of the model. We show how this is equivalent to sparse modeling of Cholesky factors of large covariance matrices. We also discuss a general approach to construct scalable Gaussian Processes using sparse local kriging. We present a multivariate data analysis which demonstrates how the nearest neighbor approach yields inference indistinguishable from the full rank GP despite being several times faster. Finally, we also propose a variant of the NNGP model for automating the selection of the neighbor set size.
The statistical modelling of spatial data plays an important role in the geological and environme... more The statistical modelling of spatial data plays an important role in the geological and environmental sciences. Multivariate spatial modelling techniques have recently surfaced as important inferential tools of spatial data analysis. This dissertation studies multivariate spatial processes as ...
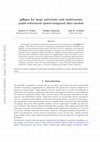
Journal of Statistical Software, 2015
In this paper we detail the reformulation and rewrite of core functions in the spBayes R package.... more In this paper we detail the reformulation and rewrite of core functions in the spBayes R package. These efforts have focused on improving computational efficiency, flexibility, and usability for point-referenced data models. Attention is given to algorithm and computing developments that result in improved sampler convergence rate and efficiency by reducing parameter space; decreased sampler run-time by avoiding expensive matrix computations, and; increased scalability to large datasets by implementing a class of predictive process models that attempt to overcome computational hurdles by representing spatial processes in terms of lower-dimensional realizations. Beyond these general computational improvements for existing model functions, we detail new functions for modeling data indexed in both space and time. These new functions implement a class of dynamic spatio-temporal models for settings where space is viewed as continuous and time is taken as discrete.
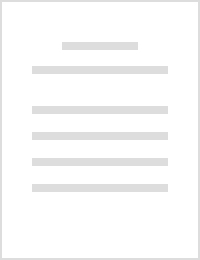
Bayesian Analysis, 2016
Multivariate disease mapping enriches traditional disease mapping studies by analysing several di... more Multivariate disease mapping enriches traditional disease mapping studies by analysing several diseases jointly. This yields improved estimates of the geographical distribution of risk from the diseases by enabling borrowing of information across diseases. Beyond multivariate smoothing for several diseases, several other variables, such as sex, age group, race, time period, and so on, could also be jointly considered to derive multivariate estimates. The resulting multivariate structures should induce an appropriate covariance model for the data. In this paper, we introduce a formal framework for the analysis of multivariate data arising from the combination of more than two variables (geographical units and at least two more variables), what we have called Multidimensional Disease Mapping. We develop a theoretical framework containing both separable and non-separable dependence structures and illustrate its performance on the study of real mortality data in Comunitat Valenciana (Spain).
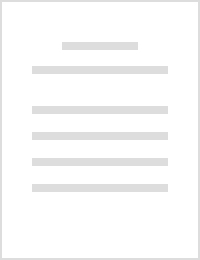
Global change biology, Jan 31, 2015
As global temperatures rise, variation in annual climate is also changing, with unknown consequen... more As global temperatures rise, variation in annual climate is also changing, with unknown consequences for forest biomes. Growing forests have the ability to capture atmospheric CO2 and thereby slow rising CO2 concentrations. Forests' ongoing ability to sequester C depends on how tree communities respond to changes in climate variation. Much of what we know about tree and forest response to climate variation comes from tree-ring records. Yet typical tree-ring datasets and models do not capture the diversity of climate responses that exist within and among trees and species. We address this issue using a model that estimates individual tree response to climate variables while accounting for variation in individuals' size, age, competitive status, and spatially-structured latent covariates. Our model allows for inference about variance within and among species. We quantify how variables influence aboveground biomass growth of individual trees from a representative sample of 15 n...
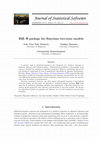
Journal of Statistical Software, 2011
A primary issue in industrial hygiene is the estimation of a worker's exposure to chemical, physi... more A primary issue in industrial hygiene is the estimation of a worker's exposure to chemical, physical and biological agents. Mathematical modeling is increasingly being used as a method for assessing occupational exposures. However, predicting exposure in real settings is constrained by lack of quantitative knowledge of exposure determinants. Recently, Zhang, Banerjee, Yang, Lungu, and Ramachandran (2009) proposed Bayesian hierarchical models for estimating parameters and exposure concentrations for the twozone differential equation models and for predicting concentrations in a zone near and far away from the source of contamination. Bayesian estimation, however, can often require substantial amounts of user-defined code and tuning. In this paper, we introduce a statistical software package, B2Z, built upon the R statistical computing platform that implements a Bayesian model for estimating model parameters and exposure concentrations in two-zone models. We discuss the algorithms behind our package and illustrate its use with simulated and real data examples.
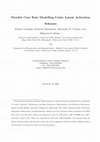
With rapid improvements in medical treatment and health care, many data sets dealing with time to... more With rapid improvements in medical treatment and health care, many data sets dealing with time to relapse or death now reveal a substantial portion of patients who are cured (that is, who never experience the event). Extended survival models called cure rate models account for the probability of a subject being cured and can be broadly classified into the classical mixture models of Berkson and Gage (1952; "BG type") or the stochastic tumor models pioneered by Yakovlev (1996) and extended to a hierarchical framework by Chen, Ibrahim and Sinha (1999; "YCIS type"). Recent developments in Bayesian hierarchical cure models have evoked significant interest regarding relationships and preferences between these two classes of models. Our present work proposes a unifying class of cure rate models that facilitates flexible hierarchical model-building while including both existing cure model classes as special cases. This unifying class enables robust modelling by accounting for uncertainty in underlying mechanisms leading to cure. Issues such as regressing on the cure fraction and propriety of the associated posterior distributions under different modelling assumptions are also discussed. Finally, we offer a simulation study and also illustrate with two data sets (one on melanoma and the other on breast cancer) that reveal our framework's ability to distinguish among underlying mechanisms that lead to relapse and cure.
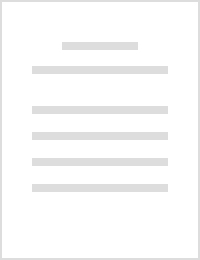
The Annals of occupational hygiene, Jan 24, 2015
Classical statistical methods for analyzing exposure data with values below the detection limits ... more Classical statistical methods for analyzing exposure data with values below the detection limits are well described in the occupational hygiene literature, but an evaluation of a Bayesian approach for handling such data is currently lacking. Here, we first describe a Bayesian framework for analyzing censored data. We then present the results of a simulation study conducted to compare the β-substitution method with a Bayesian method for exposure datasets drawn from lognormal distributions and mixed lognormal distributions with varying sample sizes, geometric standard deviations (GSDs), and censoring for single and multiple limits of detection. For each set of factors, estimates for the arithmetic mean (AM), geometric mean, GSD, and the 95th percentile (X0.95) of the exposure distribution were obtained. We evaluated the performance of each method using relative bias, the root mean squared error (rMSE), and coverage (the proportion of the computed 95% uncertainty intervals containing t...
Nonparametric Bayesian Inference in Biostatistics, 2015
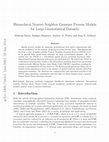
Journal of the American Statistical Association, 2015
Spatial process models for analyzing geostatistical data entail computations that become prohibit... more Spatial process models for analyzing geostatistical data entail computations that become prohibitive as the number of spatial locations become large. This manuscript develops a class of highly scalable Nearest Neighbor Gaussian Process (NNGP) models to provide fully model-based inference for large geostatistical datasets. We establish that the NNGP is a well-defined spatial process providing legitimate finitedimensional Gaussian densities with sparse precision matrices. We embed the NNGP as a dimension-reducing prior within a rich hierarchical modeling framework and develop computationally efficient Markov chain Monte Carlo (MCMC) algorithms avoiding the storage or decomposition of large matrices. The floating point operations (flops) per iteration of this algorithm is linear in the number of spatial locations, thereby delivering substantial scalability. We illustrate the computational and inferential benefits of the NNGP using simulation experiments and also infer on forest biomass from a massive United States Forest Inventory dataset.
Uploads
Papers by SUDIPTO BANERJEE