Papers by SILVIA CATERINE MORENO VILLA
Romanizacion Fronteras Y Etnias En La Roma Antigua El Caso Hispano 2013 Isbn 978 84 9860 780 2 Pags 307 334, 2012
Estudios De Filosofia Practica E Historia De Las Ideas, Dec 1, 2012
Numerical Functional Analysis and Optimization, 2010
Jmlr, 2010
In this paper we consider a regularization approach to variable selection when the regression fun... more In this paper we consider a regularization approach to variable selection when the regression function depends nonlinearly on a few input variables. The proposed method is based on a regularized least square estimator penalizing large values of the partial derivatives. An efficient iterative procedure is proposed to solve the underlying variational problem, and its convergence is proved. The empirical properties of the obtained estimator are tested both for prediction and variable selection. The algorithm compares favorably to more standard ridge regression and 1 regularization schemes.
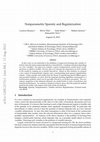
In this work we are interested in the problems of supervised learning and variable selection when... more In this work we are interested in the problems of supervised learning and variable selection when the input-output dependence is described by a nonlinear function depending on a few variables. Our goal is to consider a sparse nonparametric model, hence avoiding linear or additive models. The key idea is to measure the importance of each variable in the model by making use of partial derivatives. Based on this intuition we propose a new notion of nonparametric sparsity and a corresponding least squares regularization scheme. Using concepts and results from the theory of reproducing kernel Hilbert spaces and proximal methods, we show that the proposed learning algorithm corresponds to a minimization problem which can be provably solved by an iterative procedure. The consistency properties of the obtained estimator are studied both in terms of prediction and selection performance. An extensive empirical analysis shows that the proposed method performs favorably with respect to the state-of-the-art methods.
We consider the fundamental question of learnability of a hypotheses class in the supervised lear... more We consider the fundamental question of learnability of a hypotheses class in the supervised learning setting and in the general learning setting introduced by Vladimir Vapnik. We survey classic results characterizing learnability in term of suitable notions of complexity, as well as more recent results that establish the connection between learnability and stability of a learning algorithm.
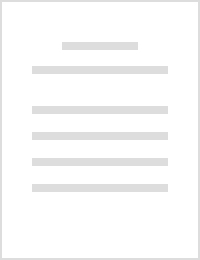
Brit J Haematol, 1984
Peripheral human blood mononuclear leucocytes (MNL) aggregated in response to arachidonic acid (A... more Peripheral human blood mononuclear leucocytes (MNL) aggregated in response to arachidonic acid (AA) in vitro. This phenomenon was similar to that already described for polymorphonuclear cells (PMN). The effect of AA was concentration-dependent and was shared only by the structurally related di-homo-gamma-linolenic acid among the other fatty acids tested. A number of agents able to induce platelet aggregation such as ADP, collagen, serotonin and a stable prostaglandin analogue all failed to stimulate MNL or PMN aggregation. Inhibitors of cyclo-oxygenase activity such as acetylsalicylic acid and indomethacin not only did not prevent AA-induced aggregation, but even potentiated it. In contrast, both nordihydroguaiaretic acid and BW 755C, two inhibitors of cyclo-oxygenase and lipoxygenase, strongly prevented MNL aggregation. Thus AA seems to aggregate MNL through the mediation of lipoxygenase products. This is supported by the observation that leukotriene B4 (LTB4) also induced MNL aggregation. When highly purified lymphocyte and monocyte preparations were assessed separately, the latter responded to AA similarly to mixed MNL whereas lymphocyte aggregation was inconsistent, small and reversible even at high concentrations of AA. Although the pathophysiological significance of the MNL aggregation described here is still obscure, assembly of these cells--particularly monocytes--at the site of injury might be a crucial event.
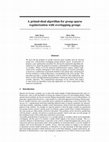
We deal with the problem of variable selection when variables must be selected group-wise, with p... more We deal with the problem of variable selection when variables must be selected group-wise, with possibly overlapping groups defined a priori. In particular we propose a new optimization procedure for solving the regularized algorithm presented in , where the group lasso penalty is generalized to overlapping groups of variables. While in [12] the proposed implementation requires explicit replication of the variables belonging to more than one group, our iterative procedure is based on a combination of proximal methods in the primal space and projected Newton method in a reduced dual space, corresponding to the active groups. This procedure provides a scalable alternative with no need for data duplication, and allows to deal with high dimensional problems without pre-processing for dimensionality reduction. The computational advantages of our scheme with respect to state-of-the-art algorithms using data duplication are shown empirically with numerical simulations.
This paper proposes a unified framework for the investigation of learning theory in Banach spaces... more This paper proposes a unified framework for the investigation of learning theory in Banach spaces of features via regularized empirical risk minimization. The main result establishes the consistency of such learning schemes under general conditions on the loss function, the geometry of the feature space, the regularization function, and the regularization parameters. The focus is placed on Tikhonov-like regularization with totally convex functions. This broad class of regularizers provides a flexible model for various priors on the features, including in particular hard constraints and powers of norms. In addition, the proposed analysis gives new insight into basic tools such as kernel methods, feature maps, and representer theorems in a Banach space setting. Even when specialized to Hilbert spaces, this framework yields new results that significantly extend the state of the art.
We investigate the modeling and the numerical solution of machine learning problems with predicti... more We investigate the modeling and the numerical solution of machine learning problems with prediction functions which are linear combinations of elements of a possibly infinite-dimensional dictionary. We propose a novel flexible composite regularization model, which makes it possible to incorporate various priors on the coefficients of the prediction function, including sparsity and hard constraints. We show that the estimators obtained by minimizing the regularized empirical risk are consistent in a statistical sense, and we design an error-tolerant composite proximal thresholding algorithm for computing such estimators. New results on the asymptotic behavior of the proximal forward-backward splitting method are derived and exploited to establish the convergence properties of the proposed algorithm. In particular, our method features a $o(1/m)$ convergence rate in objective values.
Eur Neurol, 1978
The physiopathological role of platelet aggregation in some thromboembolic and atherosclerotic co... more The physiopathological role of platelet aggregation in some thromboembolic and atherosclerotic complications is strongly suggested on the basis of many indirect findings. The qualitative methodological approach to this problem generally used until recently is rapidly giving way to a quantitative, biochemical approach. Platelet aggregation, however, even if expressed in terms of nanomoles of a product obtained in a sophisticated reaction system, will continue to deceive investigators and clinicians who fail to view it in the adequate (although still uncertain) context of rheological and vascular interactions.
We prove novel convergence results for a stochastic forward-backward splitting algorithm for solv... more We prove novel convergence results for a stochastic forward-backward splitting algorithm for solving monotone inclusions given by the sum of a maximal monotone operator and a single-valued maximal monotone cocoercive operator. We derive convergence rates in expectation in the strongly monotone case, as well as almost sure convergence results under weaker assumptions.
Within a statistical learning setting, we propose and study an iterative regularization algorithm... more Within a statistical learning setting, we propose and study an iterative regularization algorithm for least squares defined by an incremental gradient method. In particular, we show that, if all other parameters are fixed a priori, the number of passes over the data (epochs) acts as a regularization parameter, and prove strong universal consistency, i.e. almost sure convergence of the risk, as well as sharp finite sample bounds for the iterates. Our results are a step towards understanding the effect of multiple epochs in stochastic gradient techniques in machine learning and rely on integrating statistical and optimization results.
Computing Research Repository, 2011
An extension of the Gauss-Newton algorithm is proposed to find local minimizers of penalized nonl... more An extension of the Gauss-Newton algorithm is proposed to find local minimizers of penalized nonlinear least squares problems, under generalized Lipschitz assumptions. Convergence results of local type are obtained, as well as an estimate of the radius of the convergence ball. Some applications for solving constrained nonlinear equations are discussed and the numerical performance of the method is assessed on
International Workshop on Variable Structure Systems, 2006. VSS'06., 2006
ABSTRACT Approximability of sliding motions for control systems governed by nonlinear finite-dime... more ABSTRACT Approximability of sliding motions for control systems governed by nonlinear finite-dimensional differential equations is considered. This regularity property is shown to be equivalent to Tikhonov well-posedness of a related minimisation problem in the context of relaxed controls. This allows us to give a general approximability result, which in the autonomous case has an easy to verify geometrical formulation. In the second part of the paper, we consider non-approximable sliding mode control systems. In the flavour of regularization of ill-posed problems, we propose a method of selection of well-behaved approximating trajectories converging to a prescribed ideal sliding

Nanoscale, 2015
Superparamagnetic iron oxide nanoparticles with a wide size range (2.6-14.1 nm) were synthesized ... more Superparamagnetic iron oxide nanoparticles with a wide size range (2.6-14.1 nm) were synthesized and coated with the amphiphilic poly(amidoamine) PAMAM-C 12 dendrimer. The resulting well dispersed and stable water suspensions were fully characterized in order to explore their possible use in biomedical applications. The structural and magnetic properties of the nanoparticles were preserved during the coating and were related to their relaxometric behaviour. The Nuclear Magnetic Resonance Dispersion (NMRD) profiles were found to be in accordance with the Roch model. The biocompatibility was assessed by means of cell viability tests and Transmission Electron Microscopy (TEM) analysis. The nanoparticles' capability of being detected via Magnetic Resonance Imaging (MRI) was investigated by means of clinical MRI scanners both in water and agar gel phantoms, and in a mouse model. † Electronic supplementary information (ESI) available. See
Uploads
Papers by SILVIA CATERINE MORENO VILLA