Papers by Paulin Coulibaly
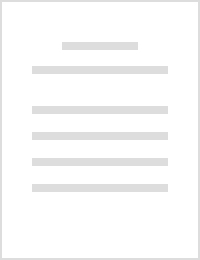
Journal of Hydrology, 2015
Summary This study highlights the impacts that the selected hydrologic characteristics of a basin... more Summary This study highlights the impacts that the selected hydrologic characteristics of a basin have on the spatial variability of hydrometric networks. The study was conducted using streamflow monitoring networks in two Canadian basins, specifically in the Hamilton, Halton, Credit Valley basins of Ontario and the Columbia River basin of British Columbia. The Dual Entropy-Multiobjective Optimization (DEMO) model which has been demonstrated to be sufficiently robust for designing optimum networks in a large basin was used in these analyses. In addition to the entropy functions, the spatial variability of flow networks was examined by either excluding or including hydrologic signatures and indicators of hydrologic alteration (IHA) of a basin. The entropy functions are used to identify optimal trade-offs between the maximum possible information content and the minimum shared information among stations. The hydrologic signatures are used to quantify hydrological response characteristics among sub-basins, and the IHAs, which are normally used to determine how a hydrologic regime has been altered by an impact, are instead used to quantify differences between sub-basins. It was found that the optimal locations for the new stations were well captured by the objective functions. Also, new stations covered a wider area when hydrological signatures and IHAs were considered, enhancing the objective functions. The inclusion of streamflow signatures increased emphasis on the headwaters whereas the inclusion of IHAs increased emphasis on the downstream and disturbed regions. Accounting for such spatial variability in designing hydrometric networks is crucial in obtaining an optimal network.
Canadian Water Resources Journal, 2013
ABSTRACT

Water Resources Research, 2013
Establishing an adequate hydrometric network to provide accurate and reliable continuous flow inf... more Establishing an adequate hydrometric network to provide accurate and reliable continuous flow information for various users remains a major challenge. This includes the design of new networks or the evaluation of existing networks. This study proposes a combined regionalization and dual entropy-multiobjective optimization (CRDEMO) method for determining minimum network that meets the World Meteorology Organization (WMO) standards, which is considered herein an optimal minimum network. A regionalization approach is used to estimate flows in potential locations for new additional stations, and a dual entropy-multiobjective optimization approach is used to identify optimal trade-offs between the maximum possible information content and the minimum shared information among the stations. The method was examined in two Canadian River basins, namely, the St. John River and St. Lawrence River basins. The St. John River basin has a higher network density compared to the St. Lawrence River basin. Results of the analysis indicate that the St. Lawrence River basin requires a high number of new additional stations to meet the WMO minimum network density. It was also determined that existing stations do not have a significant influence in determining the locations of new stations in the St. Lawrence River basin. Conversely, however, in the St. John River basin, existing stations have significant influence on the determination of locations of new stations due to the higher number of existing stations. Overall, the CRDEMO technique is shown to be robust for designing optimal minimum hydrometric networks and can be a useful tool for evaluating current and proposed networks.

Journal of Hydrology, 2014
Data assimilation (DA) has been widely used in hydrological models to improve model state and sub... more Data assimilation (DA) has been widely used in hydrological models to improve model state and subsequent streamflow estimates. However, for poor or non-existent state observations, the state estimation in hydrological DA can be problematic, leading to inaccurate streamflow updates. This study evaluates the soil moisture and flow variations and forecasts by assimilating streamflow and soil moisture. Three approaches of Ensemble Kalman Filter (EnKF) with dual state-parameter estimation are applied: (1) streamflow assimilation, (2) soil moistue assimilation, and (3) combined assimilation of soil moisture and streamflow. The assimilation approaches are evaluated using the Sacramento Soil Moisture Accounting (SAC-SMA) model in the Spencer Creek catchment in southern Ontario, Canada. The results show that there are significant differences in soil moisture variations and streamflow estimates when the three assimilation approaches were applied. In the streamflow assimilation, soil moisture states were markedly distorted, particularly soil moisture of lower soil layer; whereas, in the soil moisture assimilation, streamflow estimates are inaccurate. The combined assimilation of streamflow and soil moisture provides more accurate forecasts of both soil moisture and streamflow, particularly for shorter lead times. The combined approach has the flexibility to account for model adjustment through the time variation of parameters together with state variables when soil moisture and streamflow observations are integrated into the assimilation procedure. This evaluation is important for the application of DA methods to simultaneously estimate soil moisture states and watershed response and forecasts.
Journal of Hydrologic Engineering, 2011
... Jos Samuel, Paulin Coulibaly, Robert A. Metcalfe. Abstract. ... Since then, significant progr... more ... Jos Samuel, Paulin Coulibaly, Robert A. Metcalfe. Abstract. ... Since then, significant progress has been made in the development of regionalization methods [see Zhang and Chiew (2009) for a recent review]. However, there is no universal method for a given region or catchment. ...

Hydrological Processes, 2011
Baseflow is an integral component of environmental flow prescriptions to mitigate the impacts of ... more Baseflow is an integral component of environmental flow prescriptions to mitigate the impacts of flow regime alteration on the ecological condition of rivers and often the most contentious issue in project planning, particularly for waterpower development. Baseflow prescriptions to meet ecological objectives are increasingly derived from a natural reference condition, often a simulated streamflow time series for an ungauged basin. Accuracy of baseflow estimates is important for identifying ecological water requirements and possible ecosystem and economic tradeoffs with confidence. Estimating baseflow time series in ungauged basins to accurately quantify a natural reference condition in all regions of Ontario is particularly difficult given the heterogeneity in landscape, climate, and size of the basins where simulated time series are required. To identify the optimal hydrologic model for baseflow simulation in all regions of Ontario, five variants of McMaster University‐Hydrologiska...

Water
Flows originating from cold and mountainous watersheds are highly dependent on temperature and pr... more Flows originating from cold and mountainous watersheds are highly dependent on temperature and precipitation patterns, and the resulting snow accumulation and melt conditions, affecting the magnitude and timing of annual peak flows. This study applied a multiple linear regression (MLR) modelling framework to investigate spatial variations and relative importance of hydroclimatic drivers of annual maximum flows (AMF) and mean spring flows (MAMJflow) in 25 river basins across western Canada. The results show that basin average maximum snow water equivalent (SWEmax), April 1st SWE and spring precipitation (MAMJprc) are the most important predictors of both AMF and MAMJflow, with the proportion of explained variance averaging 51.7%, 44.0% and 33.5%, respectively. The MLR models’ abilities to project future changes in AMF and MAMJflow in response to changes to the hydroclimatic controls are also examined using the Canadian Regional Climate Model (CanRCM4) output for RCP 4.5 and RCP8.5 sc...

Climate, 2019
Flows originating from alpine dominated cold region watersheds typically experience extended wint... more Flows originating from alpine dominated cold region watersheds typically experience extended winter low flows followed by spring snowmelt and summer rainfall driven high flows. In a warmer climate, there will be a temperature-induced shift in precipitation from snowfall towards rain along with changes in precipitation intensity and snowmelt timing, resulting in alterations in the frequency and magnitude of peak flow events. This study examines the potential future changes in the frequency and severity of peak flow events in the Athabasca River watershed in Alberta, Canada. The analysis is based on simulated flow data by the variable infiltration capacity (VIC) hydrologic model driven by statistically downscaled climate change scenarios from the latest coupled model inter-comparison project (CMIP5). The hydrological model projections show an overall increase in mean annual streamflow in the watershed and a corresponding shift in the freshet timing to an earlier period. The river flow...
Neural Networks, Mar 1, 2006
(In 2 Volumes, with CD-ROM), 2004
ABSTRACT

Neural Networks, 2006
This paper presents an application of temporal neural networks for downscaling global climate mod... more This paper presents an application of temporal neural networks for downscaling global climate models (GCMs) output. Because of computational constraints, GCMs are usually run at coarse grid resolution (in the order of 100s of kilometres) and as a result they are inherently unable to present local sub-grid scale features and dynamics. Consequently, outputs from these models cannot be used directly in many climate change impact studies. This research explored the issues of 'downscaling' the outputs of GCMs using a temporal neural network (TNN) approach. The method is proposed for downscaling daily precipitation and temperature series for a region in northern Quebec, Canada. The downscaling models are developed and validated using large-scale predictor variables derived from the National Center for Environmental Prediction (NCEP) reanalysis data set. The performance of the temporal neural network downscaling model is also compared to a regression-based statistical downscaling model with emphasis on their ability in reproducing the observed climate variability and extremes. The downscaling results for the base period (1961-2000) suggest that the TNN is an efficient method for downscaling both daily precipitation as well as daily maximum and minimum temperature series. Furthermore, the different model test results indicate that the TNN model mostly outperforms the statistical models for the downscaling of daily precipitation extremes and variability.
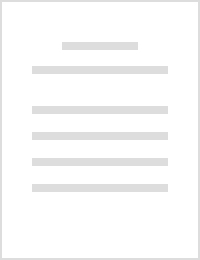
Journal of Hydrometeorology, 2005
The issues of downscaling the outputs of a global climate model (GCM) to a scale that is appropri... more The issues of downscaling the outputs of a global climate model (GCM) to a scale that is appropriate to hydrological impact studies are investigated using a temporal neural network approach. The time-lagged feed-forward neural network (TLFN) is proposed for downscaling daily total precipitation and daily maximum and minimum temperature series for the Serpent River watershed in northern Quebec (Canada). The downscaling models are developed and validated using large-scale predictor variables derived from the National Centers for Environmental Prediction–National Center for Atmospheric Research (NCEP–NCAR) reanalysis dataset. Atmospheric predictors such as specific humidity, wind velocity, and geopotential height are identified as the most relevant inputs to the downscaling models. The performance of the TLFN downscaling model is also compared to a statistical downscaling model (SDSM). The downscaling results suggest that the TLFN is an efficient method for downscaling both daily preci...
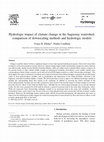
Journal of Hydrology, 2005
Changes in global climate will have significant impact on local and regional hydrological regimes... more Changes in global climate will have significant impact on local and regional hydrological regimes, which will in turn affect ecological, social and economical systems. However, climate-change impact studies on hydrologic regime have been relatively rare until recently, mainly because Global Circulation Models, which are widely used to simulate future climate scenarios, do not provide hourly or daily rainfall reliable enough for hydrological modeling. Nevertheless, more reliable rainfall series corresponding to future climate scenarios can be derived from GCM outputs using the so called 'downscaling techniques'. This study applies two types of statistical (a stochastic and a regression based) downscaling techniques to generate the possible future values of local meteorological variables such as precipitation and temperature in the Chute-du-Diable sub-basin of the Saguenay watershed in northern Québec, Canada. The downscaled data is used as input to two different hydrologic models to simulate the corresponding future flow regime in the catchment. In addition to assessing the relative potential of the downscaling methods, the paper also provides comparative study results of the possible impact of climate change on river flow and total reservoir inflow in the Chute-du-Diable basin. Although the two downscaling techniques do not provide identical results, the time series generated by both methods indicates a general increasing trend in the mean daily temperature values. While the regression based downscaling technique resulted in an increasing trend in the mean and variability of daily precipitation values, such a trend is not obvious in the case of precipitation time series downscaled with the stochastic weather generator. Moreover, the hydrologic impact analysis made with the downscaled precipitation and temperature time series as input to the two hydrological models suggest an overall increasing trend in mean annual river flow and reservoir inflow as well as earlier spring peak flows in the basin.
Journal of Hydrologic Engineering, 2011
[Journal of Hydrologic Engineering 16, 534 (2011)]. Manu Sharma, Paulin Coulibaly, Yonas Dibike. ... more [Journal of Hydrologic Engineering 16, 534 (2011)]. Manu Sharma, Paulin Coulibaly, Yonas Dibike. Abstract. ... A key area of concern for hydrologists and engineers is to determine how this warming will affect various hydrologic processes. ...

Journal of Hydroinformatics, 2008
Watershed runoff in areas with heavy seasonal snow cover is usually estimated using physically ba... more Watershed runoff in areas with heavy seasonal snow cover is usually estimated using physically based conceptual hydrologic models. Such simulation models normally require a snowmelt algorithm consisting of a surface energy balance and some accounting of internal snowpack processes to be part of the modeling system. On the other hand, artificial neural networks are flexible mathematical structures that are capable of identifying such complex nonlinear relationships between input and output datasets from historical precipitation, temperature and streamflow records. This paper presents the findings of a study on using a form of time-delayed neural network, namely time-lagged feedforward neural network (TLFN), that implicitly accounts for snow accumulation and snowmelt processes through the use of logical values and tapped delay lines. The logical values (in the form of symbolic inputs) are used to implicitly include seasonal information in the TLFN model. The proposed method has been successfully applied for improved precipitation-runoff modeling of both the Chute-du-Diable reservoir inflows and the Serpent River flows in northeastern Canada where river flows and reservoir inflows are highly influenced by seasonal snowmelt effects. The study demonstrates that the TLFN with logical values is capable of modeling the precipitation-runoff process in a cold and snowy climate by relying on 'logical input values' and tapped delay lines to implicitly recognize the temporal inputoutput patterns in the historical data. The study results also show that, once the appropriate input patterns are identified, the time-lagged neural network based models performed quite well, especially for spring peak flows, and demonstrated comparable performance in simulating the precipitation-runoff processes to that of a physically based hydrological model, namely HBV.
Hydrological Processes, 2007
... onto the same grid as that of the CGCM1. One of the well-recognized multiple linear-regressio... more ... onto the same grid as that of the CGCM1. One of the well-recognized multiple linear-regression-based statisti-cal downscaling tools, ie the Statistical Down-Scaling Model (SDSM; Wilby et al., 2002), is used to downscale the NCEP and CGCM1 predictor variables into daily ...
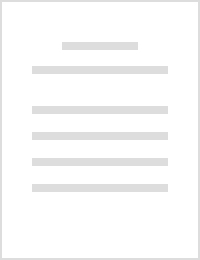
The issues of downscaling the outputs of global circulation model (GCM) to a scale appropriate to... more The issues of downscaling the outputs of global circulation model (GCM) to a scale appropriate to hydrological impact studies are investigated using functionally different methods. Three types of dynamic artificial neural networks (DANN) with different inherent representations of temporal information are investigated. Time lagged feedforward neural network (TLFN), and two types of globally recurrent neural networks (Elman and Jordan networks) are proposed for downscaling daily precipitation and temperature series for the Serpent watershed in northern Quebec (Canada). The performance of the optimal DANN model is compared to benchmarks from a statistical downscaling model and a stochastic weather generator. Overall, the downscaling results for the current period (1961-2000) suggest that the TLFN is the most efficient of the DANN models tested for downscaling both daily precipitation as well as daily temperature series. The Elman and Jordan networks performed poorly on precipitation downscaling as compared to the TDNN. Furthermore, the different model test results indicate that the optimal DANN model significantly outperforms the statistical and stochastic models for the downscaling of precipitation whatever the season. However, for minimum and maximum temperature, the TLFN and the statistical model are almost equivalent because the inherent physical process is likely less nonlinear. While changes in precipitation between the current and the future scenarios produced by the TLFN are smaller than those produced by the statistical model (except for the winter), they remain significantly larger than those suggested by the stochastic model. Thus suggesting that the TLFN can be a good trade-off alternative to the other models. Changes in streamflows between current and future periods (2020s, 2050s, and 2080s) are also compared and discussed with regard to the downscaling methods.
Uploads
Papers by Paulin Coulibaly