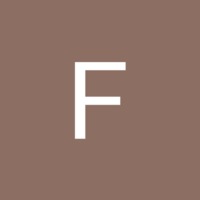
Francesco Rea
Related Authors
Sara Incao
Istituto Italiano di Tecnologia / Italian Institute of Technology
Giorgio Metta
Istituto Italiano di Tecnologia / Italian Institute of Technology
John Michael
Università degli Studi di Milano - State University of Milan (Italy)
Carlo Mazzola
Istituto Italiano di Tecnologia / Italian Institute of Technology
Chrystopher L Nehaniv
University of Waterloo, Canada
Reza Azadeh
University of Massachusetts, Lowell
Uploads
Papers by Francesco Rea