Papers by Jhonata E M E R I C K Ramos
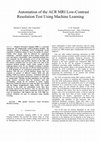
Automation of the ACR MRI Low-Contrast Resolution Test Using Machine Learning, 2018
Magnetic Resonance Imaging (MRI) is a powerful, widespread and indispensable medical imaging moda... more Magnetic Resonance Imaging (MRI) is a powerful, widespread and indispensable medical imaging modality. The American College of Radiology (ACR) recommends weekly acquisition of phantom images to assess the quality of scanner. Usually, these images must be analyzed by experienced technicians. Automatic analysis of these images would reduce costs and improve repeatability. Some automated methods have been proposed, but the automation of two of the ACR image quality tests remains open problem. Reports on the high-and low-contrast resolution tests are scarce and so far none of the proposed methods produce results robust enough to allow replacing human work. We use Machine Learning to emulate, with high accuracy, the detection of 120 low-contrast structures of ACR phantom by an experienced professional. We used a database with 620 sets of ACR phantom images that were acquired on scanners of different vendors, fields and coils, totaling 74,400 low-contrast structures. Technicians with more than 10 years of experience labeled each structure as 'detectable' or 'undetectable'. Machine learning algorithms were fed with image features extracted from the structures and their surroundings. Among the five methods we tested, Logistic Regression yielded the largest area under the ROC curve (0.878) and the highest Krippendorff's alpha (0.995). The results achieved in this study are substantially better than those previously reported in the literature. They are also better than the classifications made by junior technicians (with less than 5 years of experience). This indicate that the ACR MRI lowcontrast resolution test may be automated using Machine Learning.
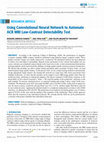
Using Convolutional Neural Network to Automate ACR MRI Low-Contrast Detectability Test, 2022
According to the American College of Radiology (ACR), the performance of magnetic resonance imagi... more According to the American College of Radiology (ACR), the performance of magnetic resonance imaging (MRI) scanners should be monitored using phantom images acquired weekly. These quality assurance images are usually analyzed by a technician, but automated analysis has been proposed to reduce costs and improve repeatability. Reports on the automation of low-contrast detectability tests are scarce, and none can completely replace human work. In previous works, we have demonstrated that machine learning methods can be used to learn the subtleties of image quality and the visual assessment of technicians. We showed that machines are able to mimic human perception quite accurately. In these works, we used hand-designed image quality features. In the present work, we use a deep learning method to automatically design appropriate image features. By training this network on a large base with visual assessments from multiple technicians, we show that the machine can be taught to assess MRI image quality better than any technician alone, justifying its widespread adoption. Our dataset contained 12,000 binary responses to the detectability of low-contrast structures (''holes''). We used the median of the technicians' responses as the gold standard. To increase statistical power, we repeated training and testing 5 times, using 5-fold crossvalidation. We obtained a mean AUC (area under the ROC curve) of 0.983±0.003. At the point of equal error rate, the mean accuracy, sensitivity and specificity were 93.2 ± 0.7%, numbers higher than those achieved by any technician alone. Applying the obtained model to a completely independent test dataset with 10,800 structures, we obtained an AUC of 0.979. The predictions of our model in classifying spokes (sets of 3 holes) agree in 93.83% of the cases with the median of the responses of the technicians. These results again are better than the responses of any individual technician. We conclude that the ACR test can be performed by a machine with greater reliability than individual technicians. INDEX TERMS Artificial intelligence, convolutional neural network, magnetic resonance imaging, machine learning, quality assurance, American College of Radiology.
Thesis Chapters by Jhonata E M E R I C K Ramos
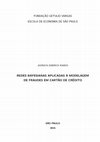
REDES BAYESIANAS APLICADAS À MODELAGEM DE FRAUDES EM CARTÃO DE CRÉDITO, 2015
Modelos para detecção de fraude são utilizados para identificar se uma transação é legítima
ou fr... more Modelos para detecção de fraude são utilizados para identificar se uma transação é legítima
ou fraudulenta com base em informações cadastrais e transacionais. A técnica proposta
no estudo apresentado, nesta dissertação, consiste na de Redes Bayesianas (RB); seus
resultados foram comparados à técnica de Regressão Logística (RL), amplamente utilizada
pelo mercado. As Redes Bayesianas avaliadas foram os classificadores bayesianos, com a
estrutura Naive Bayes. As estruturas das redes bayesianas foram obtidas a partir de dados
reais, fornecidos por uma instituição financeira. A base de dados foi separada em amostras
de desenvolvimento e validação por cross validation com dez partições. Naive Bayes foram
os classificadores escolhidos devido à simplicidade e a sua eficiência. O desempenho do
modelo foi avaliado levando-se em conta a matriz de confusão e a área abaixo da curva ROC.
As análises dos modelos revelaram desempenho, levemente, superior da regressão logística
quando comparado aos classificadores bayesianos. A regressão logística foi escolhida como
modelo mais adequado por ter apresentado melhor desempenho na previsão das operações
fraudulentas, em relação à matriz de confusão. Baseada na área abaixo da curva ROC, a
regressão logística demonstrou maior habilidade em discriminar as operações que estão
sendo classificadas corretamente, daquelas que não estão.
Uploads
Papers by Jhonata E M E R I C K Ramos
Thesis Chapters by Jhonata E M E R I C K Ramos
ou fraudulenta com base em informações cadastrais e transacionais. A técnica proposta
no estudo apresentado, nesta dissertação, consiste na de Redes Bayesianas (RB); seus
resultados foram comparados à técnica de Regressão Logística (RL), amplamente utilizada
pelo mercado. As Redes Bayesianas avaliadas foram os classificadores bayesianos, com a
estrutura Naive Bayes. As estruturas das redes bayesianas foram obtidas a partir de dados
reais, fornecidos por uma instituição financeira. A base de dados foi separada em amostras
de desenvolvimento e validação por cross validation com dez partições. Naive Bayes foram
os classificadores escolhidos devido à simplicidade e a sua eficiência. O desempenho do
modelo foi avaliado levando-se em conta a matriz de confusão e a área abaixo da curva ROC.
As análises dos modelos revelaram desempenho, levemente, superior da regressão logística
quando comparado aos classificadores bayesianos. A regressão logística foi escolhida como
modelo mais adequado por ter apresentado melhor desempenho na previsão das operações
fraudulentas, em relação à matriz de confusão. Baseada na área abaixo da curva ROC, a
regressão logística demonstrou maior habilidade em discriminar as operações que estão
sendo classificadas corretamente, daquelas que não estão.
ou fraudulenta com base em informações cadastrais e transacionais. A técnica proposta
no estudo apresentado, nesta dissertação, consiste na de Redes Bayesianas (RB); seus
resultados foram comparados à técnica de Regressão Logística (RL), amplamente utilizada
pelo mercado. As Redes Bayesianas avaliadas foram os classificadores bayesianos, com a
estrutura Naive Bayes. As estruturas das redes bayesianas foram obtidas a partir de dados
reais, fornecidos por uma instituição financeira. A base de dados foi separada em amostras
de desenvolvimento e validação por cross validation com dez partições. Naive Bayes foram
os classificadores escolhidos devido à simplicidade e a sua eficiência. O desempenho do
modelo foi avaliado levando-se em conta a matriz de confusão e a área abaixo da curva ROC.
As análises dos modelos revelaram desempenho, levemente, superior da regressão logística
quando comparado aos classificadores bayesianos. A regressão logística foi escolhida como
modelo mais adequado por ter apresentado melhor desempenho na previsão das operações
fraudulentas, em relação à matriz de confusão. Baseada na área abaixo da curva ROC, a
regressão logística demonstrou maior habilidade em discriminar as operações que estão
sendo classificadas corretamente, daquelas que não estão.