Papers by Desiree Eglee Villalta
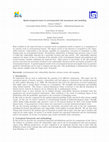
Risk is defined as the expected losses in economic terms or population death or injured, as a con... more Risk is defined as the expected losses in economic terms or population death or injured, as a consequence of an extreme event or environmental hazard. The degree of loss or loss function is recognized as the vulnerability function. Vulnerability is the intrinsic capability of a population, ecosystem or community to suffer damage from an environmental hazard. Documentary information of damage from previous events is used to build this vulnerability function for a particular location and time period. The vulnerability function expresses the degree of loss as a function of the intensity of the environmental hazard and geophysical and social-economic variables explaining the observed loses. Main sources of uncertainty in the risk modeling process come from the uncertainty attached to the vulnerability function and the uncertainty attached to the environmental hazard. A spatial-temporal model of the environmental hazard and samples from its posterior predictive distribution can be used to estimate and map risk. This methodology is demonstrated with data from extreme rainfall events for Vargas state, Venezuela.
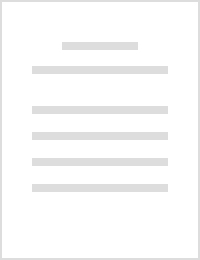
AStA Advances in Statistical Analysis, Apr 24, 2012
ABSTRACT Malaria is a parasitic infectious tropical disease that causes high mortality rates in t... more ABSTRACT Malaria is a parasitic infectious tropical disease that causes high mortality rates in the tropical belt. In Venezuela, Sucre state is considered the third state with most disease prevalence. This paper presents a hierarchical regression log-Poisson space-time model within a Bayesian approach to represent the incidence of malaria in Sucre state, Venezuela, during the period 1990–2002 in 15 municipalities of the state. Several additive models for the logarithm of the relative risk of the disease for each district were considered. These models differ in their structure by including different combinations of social-economic and climatic covariates in a multiple regression term. A random effect that captures the spatial heterogeneity in the study region, and a CAR (Conditionally Autoregressive) component that recognizes the effect of nearby municipalities in the transmission of the disease each year, are also included in the model. A simpler version without including the CAR component was also fitted to the data. Model estimation and predictive inference was carried out through the implementation of a computer code in the WinBUGS software, which makes use of Markov Chain Monte Carlo (MCMC) methods. For model selection the criterion of minimum posterior predictive loss (D) was used. The Moran I statistic was calculated to test the independence of the residuals of the resulting model. Finally, we verify the model fit by using the Bayesian p-value, and in most cases the selected model captures the spatial structure of the relative risks among the neighboring municipalities each year. For years with a poor model fit, the t-Student distribution is used as an alternative model for the spatial local random effect with better fit to the tail behavior of the data probability distribution.
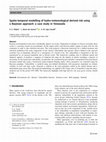
Stochastic Environmental Research and Risk Assessment, 2020
Extreme environmental events have considerable impacts on society. Preparation to mitigate or for... more Extreme environmental events have considerable impacts on society. Preparation to mitigate or forecast accurately these events is a growing concern for governments. In this regard, policy and decision makers require accurate tools for risk estimation in order to take informed decisions. This work proposes a Bayesian framework for a unified treatment and statistical modeling of the main components of risk: hazard, vulnerability and exposure. Risk is defined as the expected economic loss or population affected as a consequence of a hazard event. The vulnerability is interpreted as the loss experienced by an exposed population due to hazard events. The framework combines data of different spatial and temporal supports. It produces a sequence of temporal risk maps for the domain of interest including a measure of uncertainty for the hazard and vulnerability. In particular, the considered hazard (rainfall) is interpolated from point-based measured rainfall data using a hierarchical spatio-temporal Kriging model, whose parameters are estimated using the Bayesian paradigm. Vulnerability is modeled using zero-inflated distributions with parameters dependent on climatic variables at local and large scales. Exposure is defined as the total population settled in the spatial domain and is interpolated using census data. The proposed methodology was applied to the Vargas state of Venezuela to map the spatiotemporal risk for the period 1970-2006. The framework highlights both high and low risk areas given extreme rainfall events.
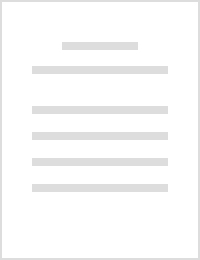
Miradas a la Venezuela del XXI. Temáticas, enfoques y evidencias. Es el resultado de las X jornad... more Miradas a la Venezuela del XXI. Temáticas, enfoques y evidencias. Es el resultado de las X jornadas de investigación, realizadas por el Instituto de Investigaciones Económicas y Sociales "Dr. Rodolfo Quintero", de la Facultad de Ciencias Económicas y Sociales, FaCES, de la Universidad Central de Venezuela, UCV. Los documentos aquí presentados, constituyen un aporte a la discusión, a la indagación crítica y el análisis sistemático de la realidad política, económica, social, humanística y tecnológica de Venezuela. Esos estudios han sido elaborados por profesores e investigadores de la Universidad Central de Venezuela y de otras instituciones académicas. Estos documentos representan las líneas de trabajo que actualmente integran la investigación y tributan un panorama de las principales preocupaciones de las Ciencias Sociales, las Humanidades, las Políticas, Económicas e incluso, lo relacionado con la informática y la web 2.0
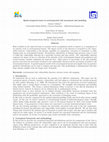
Risk is defined as the expected losses in economic terms or population death or injured, as a con... more Risk is defined as the expected losses in economic terms or population death or injured, as a consequence of an extreme event or environmental hazard. The degree of loss or loss function is recognized as the vulnerability function. Vulnerability is the intrinsic capability of a population, ecosystem or community to suffer damage from an environmental hazard. Documentary information of damage from previous events is used to build this vulnerability function for a particular location and time period. The vulnerability function expresses the degree of loss as a function of the intensity of the environmental hazard and geophysical and social-economic variables explaining the observed loses. Main sources of uncertainty in the risk modeling process come from the uncertainty attached to the vulnerability function and the uncertainty attached to the environmental hazard. A spatial-temporal model of the environmental hazard and samples from its posterior predictive distribution can be used to estimate and map risk. This methodology is demonstrated with data from extreme rainfall events for Vargas state, Venezuela.
Asia-Pacific Journal of Clinical Oncology
Asia-Pacific Journal of Clinical Oncology
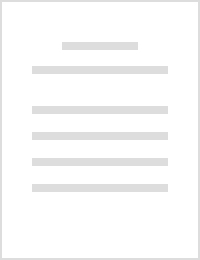
AStA Advances in Statistical Analysis, 2012
ABSTRACT Malaria is a parasitic infectious tropical disease that causes high mortality rates in t... more ABSTRACT Malaria is a parasitic infectious tropical disease that causes high mortality rates in the tropical belt. In Venezuela, Sucre state is considered the third state with most disease prevalence. This paper presents a hierarchical regression log-Poisson space-time model within a Bayesian approach to represent the incidence of malaria in Sucre state, Venezuela, during the period 1990–2002 in 15 municipalities of the state. Several additive models for the logarithm of the relative risk of the disease for each district were considered. These models differ in their structure by including different combinations of social-economic and climatic covariates in a multiple regression term. A random effect that captures the spatial heterogeneity in the study region, and a CAR (Conditionally Autoregressive) component that recognizes the effect of nearby municipalities in the transmission of the disease each year, are also included in the model. A simpler version without including the CAR component was also fitted to the data. Model estimation and predictive inference was carried out through the implementation of a computer code in the WinBUGS software, which makes use of Markov Chain Monte Carlo (MCMC) methods. For model selection the criterion of minimum posterior predictive loss (D) was used. The Moran I statistic was calculated to test the independence of the residuals of the resulting model. Finally, we verify the model fit by using the Bayesian p-value, and in most cases the selected model captures the spatial structure of the relative risks among the neighboring municipalities each year. For years with a poor model fit, the t-Student distribution is used as an alternative model for the spatial local random effect with better fit to the tail behavior of the data probability distribution.
Uploads
Papers by Desiree Eglee Villalta