Papers by Caterina Peris Ferrús
Atmospheric Environment, 2017
h i g h l i g h t s We analyze the radiative impact of a dust storm though satellite observations... more h i g h l i g h t s We analyze the radiative impact of a dust storm though satellite observations and detailed radiative transfer modeling. We evaluate the sensitivity of dust radiative effects to changes in dust optical properties. Experimentally derived dust properties reduce its radiative effects with respect to prescribed dust models.

Las nubes producen grandes cambios en el balance radiativo terrestre. Sin embargo, sigue habiendo... more Las nubes producen grandes cambios en el balance radiativo terrestre. Sin embargo, sigue habiendo incógnitas en aspectos clave concernientes a ellas, tales como sus propiedades físicas, radiativas y estructura interna. Con ello, el conocimiento acerca de su influencia en el sistema climático permanece incompleto. El efecto de las nubes es clave para el calentamiento global, y las incertidumbres acerca de sus propiedades deben ser resueltas en aras de llevar a cabo predicciones correctas del futuro cambio climático. Con todo ello, las observaciones continuas y sistemáticas de dichas propiedades es crucial. En este trabajo nos servimos de la teledetección desde suelo mediante una cámara de nubes, así como de un modelo de transferencia radiativa para inferir el espesor óptico de las nubes en todo cielo mediante un método de inversión. Los estudios de cierre radiativo de este tipo tradicionalmente se han llevado a cabo mediante una concepción plano-paralela del medio y la aplicación de ...
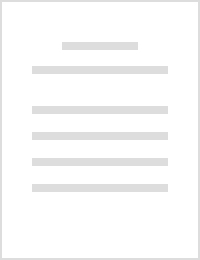
Remote Sensing of Clouds and the Atmosphere XXVI
The radiative closure methodologies to obtain Cloud Optical Depth (COD) from Remote Sensing techn... more The radiative closure methodologies to obtain Cloud Optical Depth (COD) from Remote Sensing techniques have traditionally relied on one-dimensional (1D) assumptions. These assumptions might be far away from the radiation transport over a realistic three-dimensional (3D) atmosphere, especially in cloudy conditions, as the natural inhomogeneities of clouds are not conveniently represented and treated in 1D models. The differences between the 1D and 3D approaches manifests in the 3D effects: a) the plane-parallel albedo bias and, b) the horizontal transport effect. The plane-parallel albedo bias is usually addressed by means of the Independent Pixel approximation (IPA), that considers each pixel radiatively independent from the others. Nevertheless, the IPA neglects the horizontal transport, entailing bias in the retrievals. In this work, we use the advantages of 3D radiative transfer (RT) to analyze COD and parameterize the 3D biases in terms of the plane-parallel approach. Detailed 3D RT simulations using MYSTIC are performed over two Highly Resolved Large Eddy Simulations cloud fields of known optical thickness. The output radiance is analyzed by a 1D IPA inversion retrieval based on a radiative closure to obtain the COD. The comparison between the retrieved COD fields for diverse illumination conditions and the real COD allow us to study the 3D effects separately and evaluate the retrieval. Our results show radiation enhancement in cloud edges depending on solar, viewing and cloud geometries, that induces a COD underestimation. The 1D approach works well for overcast conditions and underestimates the COD in broken clouds scenarios.

Remote Sensing
We propose a methodological approach to provide the accurate and calibrated measurements of sky r... more We propose a methodological approach to provide the accurate and calibrated measurements of sky radiance and broadband solar irradiance using the High Dynamic Range (HDR) images of a sky-camera. This approach is based on a detailed instrumental characterization of a SONA sky-camera in terms of image acquisition and processing, as well as geometric and radiometric calibrations. As a result, a 1 min time resolution database of geometrically and radiometrically calibrated HDR images has been created and has been available since February 2020, with daily updates. An extensive validation of our radiometric retrievals has been performed in all sky conditions. Our results show a very good agreement with the independent measurements of the AERONET almucantar for sky radiance and pyranometers for broadband retrievals. The SONA sky radiance shows a difference of an RMBD < 10% while the broadband diffuse radiation shows differences of 2% and 5% over a horizontal plane and arbitrarily orient...
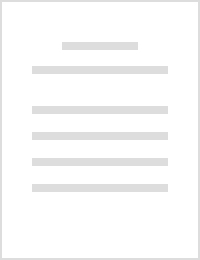
Remote Sensing of Clouds and the Atmosphere XXVI, 2021
All sky-cameras are devices with a very high potential in order to study atmospheric phenomena an... more All sky-cameras are devices with a very high potential in order to study atmospheric phenomena and were originally designed to obtain the cloud cover. However, methods based in different approaches produce significant differences in the results. State-of-art methods usually offer better performance, thanks to computer vision and machine learning (ML) techniques, than traditional algorithms based on channel ratios using both fixed and adaptive thresholds to classify the pixels of one image as cloud or cloud free. We have developed a cloud cover adaptive threshold algorithm base on Probability Density Function (PDF) of the Blue to Red Ratio (BRR), standing out in: simplicity; ease of implementation; compatibility with any sky-camera in terms of technical requirement and type of image acquisition. The goal of this study is to compare our algorithm with a most fashionable method based on Machine learning, discovering the pros and cons of each one and weather ultimately less can be more. The comparison has been done using a set of 1-year HDR imagery database, representing a wider range of atmospheric scenarios such as clear sky, cloudy, partly cloudy and different types of aerosol conditions and clouds such as cirrus, cumulus, stratum and nimbus. To stablish a quantitative comparison of both methods, a limited set of images has been chosen. The PDF method show better agreement than our ML implementation, with a better performance for all weather conditions, in comparison against our cloud cover database.
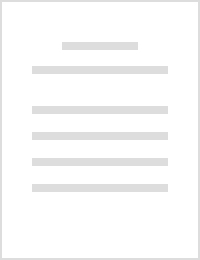
Remote Sensing of Clouds and the Atmosphere XXVI, 2021
The aim of this study is to predict the main aerosol properties in the atmosphere, Aerosol Optica... more The aim of this study is to predict the main aerosol properties in the atmosphere, Aerosol Optical Depth (AOD) and Angstrom Exponent (AE), with the aid of machine learning techniques and images from an All-Sky camera. Two different machine learning techniques have been used in this work: a random forest (RF) and an artificial neural network (ANN) with target values furnished by AERONET database. HDR images from the All-Sky camera sited in Burjassot (Spain) have been used. All of them have been taken in a clear-sky condition (without clouds) and with different aerosol depth. Selected images come out with a range from 0 to 0.5 of AOD at 500 nm as reference. The data in the groundbased station are available since the 10th of February of 2020 to the 31th March of 2021 in almost one year of samples. We have developed two ways of building signals combined with the two machine learning methods. Firstly, a signal generated from scattering angles in a single image which is obtained as the average of relative irradiance (RGB) using 100 random points in each scattering angle isoline, obtaining 29 values for each signal. Secondly, the signal has been generated in the same way but from zenith angles isolines of a single image. The main result obtained is that we improve significantly the state of art results of not calibrated images. For example, the red channel improves the percentage of predicted AOD values within the AERONET uncertainties from 62% to 90%-93% using an ANN and the zenith method.
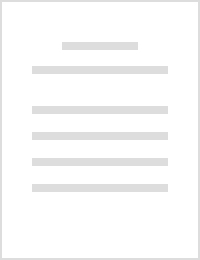
Remote Sensing of Clouds and the Atmosphere XXVI, 2021
A commercial all-sky-camera is employed to derive a whole-sky product of Cloud Optical Depth. The... more A commercial all-sky-camera is employed to derive a whole-sky product of Cloud Optical Depth. The methodology consists in a radiative closure combining measurements of the blue and red channels with libRadtran 1D monochromatic radiance simulations. Besides, a matrix of data quality Flags is obtained for every COD image. The data quality Flags indicate the reliability of the retrieval at each pixel, and gives information about the method to solve the radiance monochromatic ambivalence. In addition, the Flags product also indicates the presence of out-of-range radiances with respect to the RT simulations. Such out-of-range radiances are related to the neglection of horizontal radiation transport in the 1D plane-parallel approach. A set of around 2000 images during 2020 have been analyzed and COD has been obtained for each pixel. The COD shows values ranging from 0 to 130, with around 83% of the cases between 5 and 30. Our COD results have been validated using the zenith COD retrieval from AERONET. Our results present a very good agreement with AERONET cloud mode retrieval and show a correlation factor of 0.94 and a slope of 0.99 respectively.
Uploads
Papers by Caterina Peris Ferrús