Papers by Caterina Lázaro
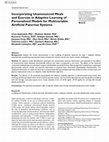
Journal of diabetes science and technology, Jan 31, 2018
Despite the recent advancements in the modeling of glycemic dynamics for type 1 diabetes mellitus... more Despite the recent advancements in the modeling of glycemic dynamics for type 1 diabetes mellitus, automatically considering unannounced meals and exercise without manual user inputs remains challenging. An adaptive model identification technique that incorporates exercise information and estimates of the effects of unannounced meals obtained automatically without user input is proposed in this work. The effects of the unknown consumed carbohydrates are estimated using an individualized unscented Kalman filtering algorithm employing an augmented glucose-insulin dynamic model, and exercise information is acquired from noninvasive physiological measurements. The additional information on meals and exercise is incorporated with personalized estimates of plasma insulin concentration and glucose measurement data in an adaptive model identification algorithm. The efficacy of the proposed personalized and adaptive modeling algorithm is demonstrated using clinical data involving closed-loop...
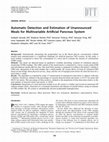
Diabetes technology & therapeutics, Mar 1, 2018
Automatically attenuating the postprandial rise in the blood glucose concentration without manual... more Automatically attenuating the postprandial rise in the blood glucose concentration without manual meal announcement is a significant challenge for artificial pancreas (AP) systems. In this study, a meal module is proposed to detect the consumption of a meal and to estimate the amount of carbohydrate (CHO) intake. The meals are detected based on qualitative variables describing variation of continuous glucose monitoring (CGM) readings. The CHO content of the meals/snacks is estimated by a fuzzy system using CGM and subcutaneous insulin delivery data. The meal bolus amount is computed according to the patient's insulin to CHO ratio. Integration of the meal module into a multivariable AP system allows revision of estimated CHO based on knowledge about physical activity, sleep, and the risk of hypoglycemia before the final decision for a meal bolus is made. The algorithm is evaluated by using 117 meals/snacks in retrospective data from 11 subjects with type 1 diabetes. Sensitivity, ...
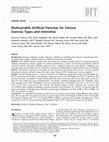
Diabetes technology & therapeutics, Oct 1, 2018
Exercise challenges people with type 1 diabetes in controlling their glucose concentration (GC). ... more Exercise challenges people with type 1 diabetes in controlling their glucose concentration (GC). A multivariable adaptive artificial pancreas (MAAP) may lessen the burden. The MAAP operates without any user input and computes insulin based on continuous glucose monitor and physical activity signals. To analyze performance, 18 60-h closed-loop experiments with 96 exercise sessions with three different protocols were completed. Each day, the subjects completed one resistance and one treadmill exercise (moderate continuous training [MCT] or high-intensity interval training [HIIT]). The primary outcome is time spent in each glycemic range during the exercise + recovery period. Secondary measures include average GC and average change in GC during each exercise modality. The GC during exercise + recovery periods were within the euglycemic range (70-180 mg/dL) for 69.9% of the time and within a safe glycemic range for exercise (70-250 mg/dL) for 93.0% of the time. The exercise sessions are...
Proceedings of the 20th Annual SIG Conference on Information Technology Education, 2019
An artificial pancreas system is implemented as a mobile application which connects a glucose sen... more An artificial pancreas system is implemented as a mobile application which connects a glucose sensor, an insulin pump and wearable physical activity sensors. It automatically delivers the optimal insulin amounts, based on a multivariable control algorithm. The algorithm, previously tested on a laptop, is to be hosted on a smartphone. This requires a fast, reliable method to translate the inherent functions. Validation tests of the new mobile application confirm there are no deviations from the original code.
Nutrition and Diabetes, 2019
2017 IEEE 60th International Midwest Symposium on Circuits and Systems (MWSCAS), 2017
This work presents security solutions related to multi-sensor closed-loop artificial pancreas (AP... more This work presents security solutions related to multi-sensor closed-loop artificial pancreas (AP) systems. The proposed AP system is built on a heterogeneous platform incorporating a smartphone, activity sensors, a glucose monitor, an insulin pump, a laptop hosting the multi-variable control algorithm and a cloud server. Developing a secure AP system is essential for mass adoption among diabetes patients. However, various communication interfaces and dynamics among the AP components result in multiple security vulnerabilities and intrusion points. We first identify the threats related to both AP data communication and AP data storage. Then, we propose several cryptography and authentication measures to address the threats related to the man-in-the-middle attacks.
2017 IEEE 14th International Conference on Wearable and Implantable Body Sensor Networks (BSN), 2017
Stress causes many physiological changes in the body and has significant effects on physiology. V... more Stress causes many physiological changes in the body and has significant effects on physiology. Various types of acute stress include social, competition, emotional and mental stress. Several studies and experiments have been conducted to investigate stress detection and measurement with physiological signals. We designed social and competition stress experiments to test our algorithms to discriminate between stress and non-stress states with physiological signals from an Empatica wristband. The algorithms were successful in detecting the presence of stress with approximately 87% accuracy.
IFAC-PapersOnLine, 2018
Continuous glucose monitoring (CGM) sensors are a critical component of artificial pancreas (AP) ... more Continuous glucose monitoring (CGM) sensors are a critical component of artificial pancreas (AP) systems that enable individuals with type 1 diabetes to achieve tighter blood glucose control. CGM sensor signals are often afflicted by a variety of anomalies, such as biases, drifts, random noises, and pressure-induced sensor attenuations. To improve the accuracy of CGM measurements, an on-line fault detection method is proposed based on sparse recursive kernel filtering algorithms to identify faults in glucose concentration values. The fault detection algorithm is designed to effectively handle the nonlinearity of the measurements and to differentiate the normal variability in the glycemic dynamics from sensor anomalies. The effectiveness of the proposed recursive kernel filtering algorithm for sensor error detection is demonstrated using simulation studies.
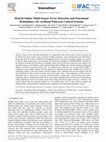
IFAC-PapersOnLine, 2018
Sensor errors limit the performance of a supervision and control system. Sensor accuracy can be a... more Sensor errors limit the performance of a supervision and control system. Sensor accuracy can be affected by many factors such as extreme working conditions, sensor deterioration and interferences from other devices. It may be difficult to distinguish sensor errors and real dynamic changes in a system. A hybrid online multi-sensor error detection and functional redundancy (HOMSED&FR) algorithm is developed to monitor the performance of multiple sensors and reconcile the erroneous sensor signals. The algorithm relies on two methods, outlier-robust Kalman filter (ORKF) and a locally-weighted partial least squares (LW-PLS) regression model. The two methods have different way of using data, ORKF is comparing current signal samples with the signal trace indicated by previous samples and LW-PLS is comparing samples in the past window with the samples from a database and uses the samples with the most similarity to build a model to predict the current signal values. The performance of this system is illustrated with a clinical case involving artificial pancreas experiments, which include data from a continuous glucose monitoring (CGM) sensor, and energy expenditure (EE) and Galvanic Skin Response (GSR) information based on wearable sensors that collect data from people with type 1 diabetes. The results indicate that the proposed method can successfully detect most of the erroneous signals and substitute them with reasonably estimated values computed by the functional redundancy system.
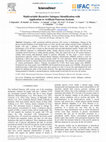
IFAC-PapersOnLine, 2017
Designing a fully automated artificial pancreas (AP) system is challenging. Changes in the glucos... more Designing a fully automated artificial pancreas (AP) system is challenging. Changes in the glucose-insulin dynamics in the human body over time, and the inter-subject and day-today variability of people with type 1 diabetes (T1D) are two important factors that would highly undermine the performance of an AP that is based on time-invariant and non-individualized models. People with T1D show different responses to carbohydrate intake, insulin, physical activity and stress with day-today variability present between or within specific patients. Thus, the control law in an AP system requires a reliable time-varying individualized model to perform efficiently. In this work, a novel recursive identification approach called a Predictor-Based Subspace Identification (PBSID) method is used for identifying a linear time-varying glucose-insulin model for each individual. Model identification and validation are based on clinical data from closed-loop experiments. The models are evaluated by means of various performances indices: Variance Accounted For (VAF), Root mean square error (RMSE), Normalized root mean square error (NRMSE) and Normalized mean square error (NMSE). The proposed method provides a stable time-varying state space model over time. It can be also individualized for each patient by defining the order of the system correctly. The approach proposed in this work has shown a strong potential to identify a consistent glucose-insulin model in real time for use in an AP system.
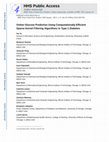
IEEE Transactions on Control Systems Technology, 2018
Streaming data from continuous glucose monitoring (CGM) systems enable the recursive identificati... more Streaming data from continuous glucose monitoring (CGM) systems enable the recursive identification of models to improve estimation accuracy for effective predictive glycemic control in patients with type-1 diabetes. A drawback of conventional recursive identification techniques is the increase in computational requirements, which is a concern for online and real-time applications such as the artificial pancreas systems implemented on handheld devices and smartphones where computational resources and memory are limited. To improve predictions in such computationally constrained hardware settings, efficient adaptive kernel filtering algorithms are developed in this paper to characterize the nonlinear glycemic variability by employing a sparsification criterion based on the information theory to reduce the computation time and complexity of the kernel filters without adversely deteriorating the predictive performance. Furthermore, the adaptive kernel filtering algorithms are designed to be insensitive to abnormal CGM measurements, thus compensating for measurement noise and disturbances. As such, the sparsification-based real-time model update framework can adapt the prediction models to accurately characterize the time-varying and nonlinear dynamics of glycemic measurements. The proposed recursive kernel filtering algorithms leveraging sparsity for improved computational efficiency are applied to both in-silico and clinical subjects, and the results demonstrate the effectiveness of the proposed methods.
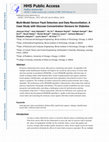
AIChE Journal, 2018
Erroneous information from sensors affect process monitoring and control. An algorithm with multi... more Erroneous information from sensors affect process monitoring and control. An algorithm with multiple model identification methods will improve the sensitivity and accuracy of sensor fault detection and data reconciliation (SFD&DR). A novel SFD&DR algorithm with four types of models including outlier robust Kalman filter, locally weighted partial least squares, predictorbased subspace identification, and approximate linear dependency-based kernel recursive least squares is proposed. The residuals are further analyzed by artificial neural networks and a voting algorithm. The performance of the SFD&DR algorithm is illustrated by clinical data from artificial pancreas experiments with people with diabetes. The glucose-insulin metabolism has time-varying parameters and nonlinearities, providing a challenging system for fault detection and data reconciliation. Data from 17 clinical experiments collected over 896 hours were analyzed; the results indicate that the proposed SFD&DR algorithm is capable of detecting and diagnosing sensor faults and reconciling the erroneous sensor signals with better model-estimated values.
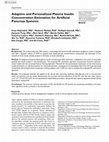
Journal of diabetes science and technology, 2018
The artificial pancreas (AP) system, a technology that automatically administers exogenous insuli... more The artificial pancreas (AP) system, a technology that automatically administers exogenous insulin in people with type 1 diabetes mellitus (T1DM) to regulate their blood glucose concentrations, necessitates the estimation of the amount of active insulin already present in the body to avoid overdosing. An adaptive and personalized plasma insulin concentration (PIC) estimator is designed in this work to accurately quantify the insulin present in the bloodstream. The proposed PIC estimation approach incorporates Hovorka's glucose-insulin model with the unscented Kalman filtering algorithm. Methods for the personalized initialization of the time-varying model parameters to individual patients for improved estimator convergence are developed. Data from 20 three-days-long closed-loop clinical experiments conducted involving subjects with T1DM are used to evaluate the proposed PIC estimation approach. The proposed methods are applied to the clinical data containing significant disturba...
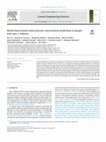
Control engineering practice, 2018
Accurate predictions of glucose concentrations are necessary to develop an artificial pancreas (A... more Accurate predictions of glucose concentrations are necessary to develop an artificial pancreas (AP) system for people with type 1 diabetes (T1D). In this work, a novel glucose forecasting paradigm based on a model fusion strategy is developed to accurately characterize the variability and transient dynamics of glycemic measurements. To this end, four different adaptive filters and a fusion mechanism are proposed for use in the online prediction of future glucose trajectories. The filter fusion mechanism is developed based on various prediction performance indexes to guide the overall output of the forecasting paradigm. The efficiency of the proposed model fusion based forecasting method is evaluated using simulated and clinical datasets, and the results demonstrate the capability and prediction accuracy of the data-based fusion filters, especially in the case of limited data availability. The model fusion framework may be used in the development of an AP system for glucose regulatio...
International Journal of Handheld Computing Research, 2017
In this work, an artificial pancreas (AP) system, implemented on a mobile device is described. Th... more In this work, an artificial pancreas (AP) system, implemented on a mobile device is described. The proposed AP platform integrates hardware (insulin pump, glucose monitor, various sensors for vital signs and physical activities) and software (closed-loop control algorithm, sensor fusion, data storage and remote server access) components via smartphone that is running a dedicated Operating System designed for AP systems. Interfacing with this OS and custom application development steps are presented. Closed loop operation is demonstrated with case studies.
International Journal of Handheld Computing Research, 2015
This paper describes a distributed sensor platform for a new breed of artificial pancreas devices... more This paper describes a distributed sensor platform for a new breed of artificial pancreas devices. In recent work, a multi-variable adaptive algorithm has been proposed which incorporates physical activity of the patients for accurate prediction and control of glucose levels. In order to facilitate this algorithm, the authors integrate a smartphone and multiple sensors including activity trackers and a glucose monitor into a distributed system. The proposed sensor platform provides real-time data access for the artificial pancreas control algorithm hosted on a remote device.
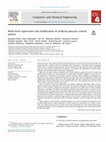
Computers & chemical engineering, Jan 6, 2018
Artificial pancreas (AP) systems provide automated regulation of blood glucose concentration (BGC... more Artificial pancreas (AP) systems provide automated regulation of blood glucose concentration (BGC) for people with type 1 diabetes (T1D). An AP includes three components: a continuous glucose monitoring (CGM) sensor, a controller calculating insulin infusion rate based on the CGM signal, and a pump delivering the insulin amount calculated by the controller to the patient. The performance of the AP system depends on successful operation of these three components. Many APs use model predictive controllers that rely on models to predict BGC and to calculate the optimal insulin infusion rate. The performance of model-based controllers depends on the accuracy of the models that is affected by large dynamic changes in glucose-insulin metabolism or equipment performance that may move the operating conditions away from those used in developing the models and designing the control system. Sensor errors and missing signals will cause calculation of erroneous insulin infusion rates. And the pe...
Uploads
Papers by Caterina Lázaro