Papers by Kristofer Bouchard
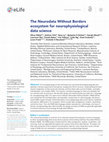
eLife, Oct 4, 2022
The neurophysiology of cells and tissues are monitored electrophysiologically and optically in di... more The neurophysiology of cells and tissues are monitored electrophysiologically and optically in diverse experiments and species, ranging from flies to humans. Understanding the brain requires integration of data across this diversity, and thus these data must be findable, accessible, interoperable, and reusable (FAIR). This requires a standard language for data and metadata that can coevolve with neuroscience. We describe design and implementation principles for a language for neurophysiology data. Our open-source software (Neurodata Without Borders, NWB) defines and modularizes the interdependent, yet separable, components of a data language. We demonstrate NWB's impact through unified description of neurophysiology data across diverse modalities and species. NWB exists in an ecosystem, which includes data management, analysis, visualization, and archive tools. Thus, the NWB data language enables reproduction, interchange, and reuse of diverse neurophysiology data. More broadly, the design principles of NWB are generally applicable to enhance discovery across biology through data FAIRness.
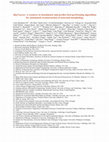
ABSTRACTBigNeuron is an open community bench-testing platform combining the expertise of neurosci... more ABSTRACTBigNeuron is an open community bench-testing platform combining the expertise of neuroscientists and computer scientists toward the goal of setting open standards for accurate and fast automatic neuron reconstruction. The project gathered a diverse set of image volumes across several species representative of the data obtained in most neuroscience laboratories interested in neuron reconstruction. Here we report generated gold standard manual annotations for a selected subset of the available imaging datasets and quantified reconstruction quality for 35 automatic reconstruction algorithms. Together with image quality features, the data were pooled in an interactive web application that allows users and developers to perform principal component analysis, t-distributed stochastic neighbor embedding, correlation and clustering, visualization of imaging and reconstruction data, and benchmarking of automatic reconstruction algorithms in user-defined data subsets. Our results show ...
arXiv (Cornell University), Oct 14, 2022
Self-driving labs (SDLs) combine fully automated experiments with artificial intelligence (AI) th... more Self-driving labs (SDLs) combine fully automated experiments with artificial intelligence (AI) that decides the next set of experiments. Taken to their ultimate expression, SDLs could usher a new paradigm of scientific research, where the world is probed, interpreted, and explained by machines for human benefit. While there are functioning SDLs in the fields of chemistry and materials science, we contend that synthetic biology provides a unique opportunity since the genome provides a single target for affecting the incredibly wide repertoire of biological cell behavior. However, the level of investment required for the creation of biological SDLs is only warranted if directed towards solving difficult and enabling biological questions. Here, we discuss challenges and opportunities in creating SDLs for synthetic biology.
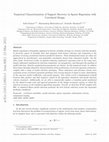
arXiv (Cornell University), Mar 23, 2021
Sparse regression is frequently employed in diverse scientific settings as a feature selection me... more Sparse regression is frequently employed in diverse scientific settings as a feature selection method. A pervasive aspect of scientific data that hampers both feature selection and estimation is the presence of strong correlations between predictive features. These fundamental issues are often not appreciated by practitioners, and jeapordize conclusions drawn from estimated models. On the other hand, theoretical results on sparsity-inducing regularized regression such as the Lasso have largely addressed conditions for selection consistency via asymptotics, and disregard the problem of model selection, whereby regularization parameters are chosen. In this numerical study, we address these issues through exhaustive characterization of the performance of several regression estimators, coupled with a range of model selection strategies. These estimators and selection criteria were examined across correlated regression problems with varying degrees of signal to noise, distribution of the non-zero model coefficients, and model sparsity. Our results reveal a fundamental tradeoff between false positive and false negative control in all regression estimators and model selection criteria examined. Additionally, we are able to numerically explore a transition point modulated by the signal-to-noise ratio and spectral properties of the design covariance matrix at which the selection accuracy of all considered algorithms degrades. Overall, we find that SCAD coupled with BIC or empirical Bayes model selection performs the best feature selection across the regression problems considered.
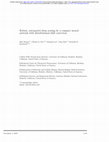
bioRxiv (Cold Spring Harbor Laboratory), Oct 21, 2019
Studying the biology of sleep requires the accurate assessment of the state of experimental subje... more Studying the biology of sleep requires the accurate assessment of the state of experimental subjects, and manual analysis of relevant data is a major bottleneck. Recently, deep learning applied to electroencephalogram and electromyogram data has shown great promise as a sleep scoring method, approaching the limits of inter-rater reliability. As with any machine learning algorithm, the inputs to a sleep scoring classifier are typically standardized in order to remove distributional shift caused by variability in the signal collection process. However, in scientific data, experimental manipulations introduce variability that should not be removed. For example, in sleep scoring, the fraction of time spent in each arousal state can vary between control and experimental subjects. We introduce a standardization method, mixture z-scoring, that preserves this crucial form of distributional shift. Using both a simulated experiment and mouse in vivo data, we demonstrate that a common standardization method used by state-of-the-art sleep scoring algorithms introduces systematic bias, but that mixture z-scoring does not. We present a free, open-source user interface that uses a compact neural network and mixture z-scoring to allow for rapid sleep scoring with accuracy that compares well to contemporary methods. This work provides a set of computational tools for the robust automation of sleep scoring.
bioRxiv (Cold Spring Harbor Laboratory), Jul 12, 2023
In the brain, all neurons are driven by the activity of other neurons, some of which maybe simult... more In the brain, all neurons are driven by the activity of other neurons, some of which maybe simultaneously recorded, but most are not. As such, models of neuronal activity need to account for simultaneously recorded neurons and the influences of unmeasured neurons. This can be done through inclusion of model terms for observed external variables (e.g., tuning to stimuli) as well as terms for latent sources of variability. Determining the influence of groups of neurons on each .
Biomolecules, Mar 24, 2023
This article is an open access article distributed under the terms and conditions of the Creative... more This article is an open access article distributed under the terms and conditions of the Creative Commons Attribution (CC BY
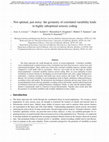
bioRxiv (Cold Spring Harbor Laboratory), Mar 9, 2022
The brain represents the world through the activity of neural populations. Correlated variability... more The brain represents the world through the activity of neural populations. Correlated variability across simultaneously recorded neurons (noise correlations) has been observed across cortical areas and experimental paradigms. Many studies have shown that correlated variability improves stimulus coding compared to a null model with no correlations. However, such results do not shed light on whether neural populations' correlated variability achieves optimal coding. Here, we assess optimality of noise correlations in diverse datasets by developing two novel null models each with a unique biological interpretation: a uniform correlations null model and a factor analysis null model. We show that across datasets, the correlated variability in neural populations leads to highly suboptimal coding performance according to these null models. We demonstrate that biological constraints prevent many subsets of the neural populations from achieving optimality according to these null models, and that subselecting based on biological criteria leaves coding performance suboptimal. Finally, we show that the optimal subpopulation is exponentially small as a function of neural dimensionality. Together, these results show that the geometry of correlated variability leads to highly suboptimal sensory coding.
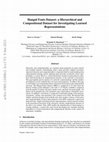
arXiv (Cornell University), May 23, 2019
Hierarchy and compositionality are common latent properties in many natural and scientific datase... more Hierarchy and compositionality are common latent properties in many natural and scientific datasets. Determining when a deep network's hidden activations represent hierarchy and compositionality is important both for understanding deep representation learning and for applying deep networks in domains where interpretability is crucial. However, current benchmark machine learning datasets either have little hierarchical or compositional structure, or the structure is not known. This gap impedes precise analysis of a network's representations and thus hinders development of new methods that can learn such properties. To address this gap, we developed a new benchmark dataset with known hierarchical and compositional structure. The Hangul Fonts Dataset (HFD) is comprised of 35 fonts from the Korean writing system (Hangul), each with 11,172 blocks (syllables) composed from the product of initial consonant, medial vowel, and final consonant glyphs. All blocks can be grouped into a few geometric types which induces a hierarchy across blocks. In addition, each block is composed of individual glyphs with rotations, translations, scalings, and naturalistic style variation across fonts. We find that both shallow and deep unsupervised methods only show modest evidence of hierarchy and compositionality in their representations of the HFD compared to supervised deep networks. Supervised deep network representations contain structure related to the geometrical hierarchy of the characters, but the compositional structure of the data is not evident. Thus, HFD enables the identification of shortcomings in existing methods, a critical first step toward developing new machine learning algorithms to extract hierarchical and compositional structure in the context of naturalistic variability. Preprint. Under review.
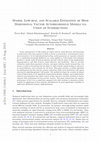
arXiv (Cornell University), Aug 29, 2019
Vector autoregressive (V AR) models are widely used for causal discovery and forecasting in multi... more Vector autoregressive (V AR) models are widely used for causal discovery and forecasting in multivariate time series analyses in fields as diverse as neuroscience, environmental science, and econometrics. In the high-dimensional setting, model parameters are typically estimated by L 1-regularized maximum likelihood; yet, when applied to V AR models, this technique produces a sizable trade-off between sparsity and bias with the choice of the regularization hyperparameter, and thus between causal discovery and prediction. That is, low-bias estimation entails dense parameter selection, and sparse selection entails increased bias; the former is useful in forecasting but less likely to yield scientific insight leading to discovery of causal influences, and conversely for the latter. This paper presents a scalable algorithm for simultaneous low-bias and low-variance estimation (hence good prediction) with sparse selection for high-dimensional V AR models. The method leverages the recently developed Union of Intersections (U oI) algorithmic framework for flexible, modular, and scalable feature selection and estimation that allows control of false discovery and false omission in feature selection while maintaining low bias and low variance. This paper demonstrates the superior performance of the U oI V AR algorithm compared with other methods in simulation studies, exhibits its application in data analysis, and illustrates its good algorithmic scalability in multi-node distributed memory implementations.
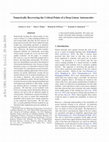
arXiv (Cornell University), Jan 29, 2019
Numerically locating the critical points of nonconvex surfaces is a long-standing problem central... more Numerically locating the critical points of nonconvex surfaces is a long-standing problem central to many fields. Recently, the loss surfaces of deep neural networks have been explored to gain insight into outstanding questions in optimization, generalization, and network architecture design. However, the degree to which recentlyproposed methods for numerically recovering critical points actually do so has not been thoroughly evaluated. In this paper, we examine this issue in a case for which the ground truth is known: the deep linear autoencoder. We investigate two sub-problems associated with numerical critical point identification: first, because of large parameter counts, it is infeasible to find all of the critical points for contemporary neural networks, necessitating sampling approaches whose characteristics are poorly understood; second, the numerical tolerance for accurately identifying a critical point is unknown, and conservative tolerances are difficult to satisfy. We first identify connections between recently-proposed methods and well-understood methods in other fields, including chemical physics, economics, and algebraic geometry. We find that several methods work well at recovering certain information about loss surfaces, but fail to take an unbiased sample of critical points. Furthermore, numerical tolerance must be very strict to ensure that numericallyidentified critical points have similar properties to true analytical critical points. We also identify a recently-published Newton method for optimization that outperforms previous methods as
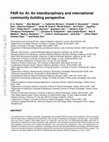
arXiv (Cornell University), Sep 30, 2022
A foundational set of findable, accessible, interoperable, and reusable (FAIR) principles were pr... more A foundational set of findable, accessible, interoperable, and reusable (FAIR) principles were proposed in 2016 as prerequisites for proper data management and stewardship, with the goal of enabling the reusability of scholarly data. The principles were also meant to apply to other digital assets, at a high level, and over time, the FAIR guiding principles have been reinterpreted or extended to include the software, tools, algorithms, and workflows that produce data. FAIR principles are now being adapted in the context of AI models and datasets. Here, we present the perspectives, vision, and experiences of researchers from different countries, disciplines, and backgrounds who are leading the definition and adoption of FAIR principles in their communities of practice, and discuss outcomes that may result from pursuing and incentivizing FAIR AI research. The material for this report builds on the FAIR for AI Workshop held at Argonne National Laboratory on June 7, 2022.
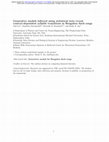
Generative models are widely used in modeling sequences from language to birdsong. Here we show t... more Generative models are widely used in modeling sequences from language to birdsong. Here we show that a statistical test designed to guard against overgeneralization of a model in generating sequences can be used to infer minimal models for the variable syllable sequences of Bengalese finch songs. Specifically, the generative model we consider is the partially observable Markov model (POMM). A POMM consists of states and probabilistic transitions between them. Each state is associated with a syllable, and one syllable can be associated with multiple states. This multiplicity of association from syllable to states distinguishes a POMM from a simple Markov model, in which one syllable is associated with one state. The multiplicity indicates that syllable transitions are context-dependent. The statistical test is used to infer a POMM with minimal number of states from a finite number of observed sequences. We apply the method to infer POMMs for songs of six adult male Bengalese finches ...
Current Opinion in Biotechnology
Self-driving labs (SDLs) combine fully automated experiments with artificial intelligence (AI) th... more Self-driving labs (SDLs) combine fully automated experiments with artificial intelligence (AI) that decides the next set of experiments. Taken to their ultimate expression, SDLs could usher a new paradigm of scientific research, where the world is probed, interpreted, and explained by machines for human benefit. While there are functioning SDLs in the fields of chemistry and materials science, we contend that synthetic biology provides a unique opportunity since the genome provides a single target for affecting the incredibly wide repertoire of biological cell behavior. However, the level of investment required for the creation of biological SDLs is only warranted if directed toward solving difficult and enabling biological questions. Here, we discuss challenges and opportunities in creating SDLs for synthetic biology.
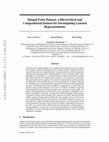
arXiv (Cornell University), May 23, 2019
Hierarchy and compositionality are common latent properties in many natural and scientific datase... more Hierarchy and compositionality are common latent properties in many natural and scientific datasets. Determining when a deep network's hidden activations represent hierarchy and compositionality is important both for understanding deep representation learning and for applying deep networks in domains where interpretability is crucial. However, current benchmark machine learning datasets either have little hierarchical or compositional structure, or the structure is not known. This gap impedes precise analysis of a network's representations and thus hinders development of new methods that can learn such properties. To address this gap, we developed a new benchmark dataset with known hierarchical and compositional structure. The Hangul Fonts Dataset (HFD) is comprised of 35 fonts from the Korean writing system (Hangul), each with 11,172 blocks (syllables) composed from the product of initial consonant, medial vowel, and final consonant glyphs. All blocks can be grouped into a few geometric types which induces a hierarchy across blocks. In addition, each block is composed of individual glyphs with rotations, translations, scalings, and naturalistic style variation across fonts. We find that both shallow and deep unsupervised methods only show modest evidence of hierarchy and compositionality in their representations of the HFD compared to supervised deep networks. Supervised deep network representations contain structure related to the geometrical hierarchy of the characters, but the compositional structure of the data is not evident. Thus, HFD enables the identification of shortcomings in existing methods, a critical first step toward developing new machine learning algorithms to extract hierarchical and compositional structure in the context of naturalistic variability. Preprint. Under review.
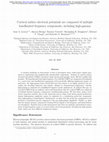
A common challenge in neuroscience is how to decompose noisy, multi-source signals measured in ex... more A common challenge in neuroscience is how to decompose noisy, multi-source signals measured in experiments into biophysically interpretable components. Analysis of cortical surface electrical potentials (CSEPs) measured using electrocorticography arrays (ECoG) typifies this problem. We hypothesized that high frequency (70-1,000 Hz) CSEPs are composed of broadband (i.e., power-law) and bandlimited components with potentially differing biophysical origins. In particular, the high-gamma band (70-150 Hz) has been shown to be highly predictive for encoding and decoding behaviors and stimuli. Despite its demonstrated importance, whether high-gamma is composed of a bandlimited signal is poorly understood. To address this gap, we recorded CSEPs from rat auditory cortex and demonstrate that the evoked CSEPs are composed of multiple distinct frequency components, including high-gamma. We then show, using a novel robust regression method, that at fast timescales and on single trials during spe...
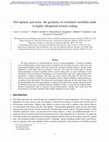
The brain represents the world through the activity of neural populations. Correlated variability... more The brain represents the world through the activity of neural populations. Correlated variability across simultaneously recorded neurons (noise correlations) has been observed across cortical areas and experimental paradigms. Many studies have shown that correlated variability improves stimulus coding compared to a null model with no correlations. However, such results do not shed light on whether neural populations’ correlated variability achieves optimal coding. Here, we assess optimality of noise correlations in diverse datasets by developing two novel null models each with a unique biological interpretation: a uniform correlations null model and a factor analysis null model. We show that across datasets, the correlated variability in neural populations leads to highly suboptimal coding performance according to these null models. We demonstrate that biological constraints prevent many subsets of the neural populations from achieving optimality according to these null models, and ...
Dynamical Components Analysis is a Python implementation of the method described in "Unsuper... more Dynamical Components Analysis is a Python implementation of the method described in "Unsupervised discovery of temporal structure in noisy data with dynamical components analysis". It implements the method as well as related data analysis functions.
Uploads
Papers by Kristofer Bouchard