Papers by Adrianna Loback
bioRxiv (Cold Spring Harbor Laboratory), Oct 21, 2017
Memories link information about specific experiences to more general knowledge that is abstracted... more Memories link information about specific experiences to more general knowledge that is abstracted from and contextualizes those experiences, but how neuronal activity patterns support this link is not known. Here we show that during memory reactivation in a foraging task with multiple spatial paths, specific hippocampal place representations are .
medRxiv (Cold Spring Harbor Laboratory), Mar 22, 2024
arXiv (Cornell University), Oct 21, 2016
<p>Likelihood of the hidden Markov model plotted as a function of the total number of modes... more <p>Likelihood of the hidden Markov model plotted as a function of the total number of modes. The real data (blue) contained many more modes (50 modes) than either a linear-nonlinear (LN) model fit to the same data (green, 6 modes) or the data with each cell’s spike train randomly shuffled to eliminate correlations (red, 1 mode). All likelihoods were estimated in 10-fold cross-validation. For each fold, we defined a normalized log-likelihood by subtracting the maximum log-likelihood over mode number. These normalized log-likelihoods were then averaged over the 10 folds. Error bars: +/- 1 standard deviation across folds.</p
<p>(A) Average number of cells within a given mode (red) and average number of modes contai... more <p>(A) Average number of cells within a given mode (red) and average number of modes containing a given cell (blue) plotted as a function of the firing threshold, <i>θ</i>. (B) Histogram of the number of cells within a given mode for <i>θ</i> = 3. (C) Histogram of the number of modes containing a given cell for <i>θ</i> = 3.</p
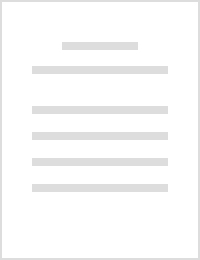
<p>(A) In the limit of no temporal correlations, the probability over all binary spike word... more <p>(A) In the limit of no temporal correlations, the probability over all binary spike words is given by a mixture over different emission distributions. (i) In the case of no correlation within an emission distribution, <i>Q</i><sup><i>ind</i></sup> has a simple product form; (ii) our full model includes pairwise correlations within an emission distribution, <i>Q</i><sup><i>tree</i></sup>, having a tree graphical structure. (B) <i>Left</i>: The full model also includes temporal correlation through a transition probability matrix, <i>P</i>(<i>α</i>(<i>t</i>)|<i>α</i>(<i>t</i>−1)), which describes the probability of finding mode <i>α</i> at time step <i>t</i> given the mode present in the previous time step, <i>t-1</i>. The hidden state (mode identity), <i>α</i>, evolves probabilistically over time according to the transition matrix, and the observed pattern of spiking at time <i>t</i>, {<i>σ</i><sub><i>i</i></sub>(<i>t</i>)}, has a mode-dependent emission probability, . <i>Right</i>: Schematic representation of the tree graphical structure of each emission distribution. (C) In order to find the stationary distribution of the HMM, we solved for the set of mode weights, {<i>w</i><sub><i>α</i></sub>}, using the detailed balance equation. (D) The total number of modes, <i>M</i>, was determined by finding the maximum 2-fold cross-validated likelihood of the model. Note that the optimum was relatively shallow, so that the model is not sensitive to the precise number of modes, as long as it lies within a certain range (for this dataset, roughly 30–100 modes).</p
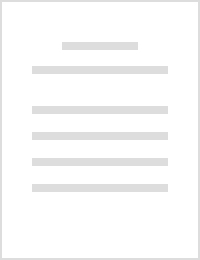
<p>(A) Overall mode weight, <i>w</i><sub><i>α</i></sub>... more <p>(A) Overall mode weight, <i>w</i><sub><i>α</i></sub>, versus mode index. (B) The mean spiking probability of each mode, <i>m</i><sub><i>iα</i></sub>, projected into two dimensions by multidimensional scaling (MDS). The color of each point indicates that mode’s log probability (as in A) while the size scales with the mode’s average population spike count, ⟨<i>k</i>⟩. Modes with higher ⟨<i>k</i>⟩ pack response space less densely. (C) Entropy rate of mode emission distribution, <i>S</i><sub><i>α</i></sub> (see <a href="http://www.ploscompbiol.org/article/info:doi/10.1371/journal.pcbi.1005148#sec014" target="_blank">Methods</a>), plotted against average population spike count of the mode. Dashed gray line: <i>Smax</i>, the maximum entropy for fixed <i>k</i>; i.e. the entropy of the uniform distribution over all <i>k</i>-spike words (see <a href="http://www.ploscompbiol.org/article/info:doi/10.1371/journal.pcbi.1005148#sec014" target="_blank">Methods</a>). <i>Inset</i>: the ratio of maximum entropy to mode entropy. (D) Schematic representation of the structure of the response probability distribution. Each lump represents one mode, with the overall amplitude decreasing and width increasing with average response magnitude, <i>m</i>. The tall, narrow, central peak corresponds to the silent and near-silent words near <i>m</i> = 0.</p
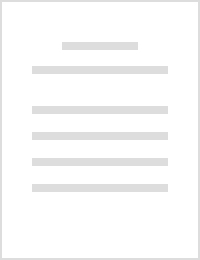
<p>Performance was assessed by the log-likelihood evaluated on a 50% held-out test set. We ... more <p>Performance was assessed by the log-likelihood evaluated on a 50% held-out test set. We generated 10 random 50% train-test splits; the box plots indicate the distribution of test log-likelihood over these splits. Red line = median; blue box = 25% (lower) and 75% (upper) percentiles; black lines = minimum (lower) and maximum (upper). (A) The HMM outperformed the K-pairwise maximum entropy model. (B) Comparison of the HMM, the same maximum entropy fits as <i>A</i>, and the reliable interactions model constrained on words occurring at least twice in the train set. Note that the reliable interactions model is a pseudo-likelihood model and therefore does not allow calculation of a meaningful log-likelihood: we therefore followed the convention established in [<a href="http://www.ploscompbiol.org/article/info:doi/10.1371/journal.pcbi.1005148#pcbi.1005148.ref052" target="_blank">52</a>] and calculated the log-likelihood summed only over words occurring at least twice in the data. In panel (B), we compare the same truncated log-likelihood across all three models.</p
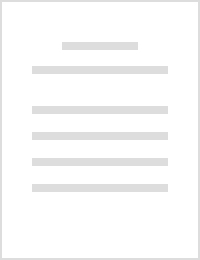
<p>Example receptive fields of modes, measured as the mode-triggered stimulus average durin... more <p>Example receptive fields of modes, measured as the mode-triggered stimulus average during a white noise checkerboard stimulus. In each panel, the left sub-panel exhibits the mode’s spatial receptive field (<i>top</i>) and temporal profile (<i>bottom</i>). Spatial receptive fields have been smoothed with a Gaussian kernel (1 check standard deviation) and interpolated by a factor of 4. The right sub-panel shows a 1-standard deviation contour of the best Gaussian fit to the mode receptive field (black ellipse) compared to the same fit to individual cells’ spike-triggered average receptive field (red: ON-type cells, blue: OFF-type cells). The saturation of the ellipse’s color represents the cell’s mode-dependent spiking probability <i>m</i><sub><i>iα</i></sub>. Scale bars = 5 checks. We observed three qualitative categories of mode receptive field: (A) <i>Intersection</i>. These had highly localized, compact, and symmetrical receptive fields located at the intersection of many single-cell fields. Half the modes were of the intersection type. (B) <i>Union</i>. These were larger and more elongated, were spanned by several individual receptive fields, and occurred as both OFF-type (<i>left</i>) and ON-type (<i>right</i>). (C) <i>Dipole</i>. These received a strong contribution from both ON- and OFF-cells, with the two populations forming separate clusters with low overlap. The overall mode receptive field therefore consisted of an oriented pair of ON- and OFF-subfields.</p
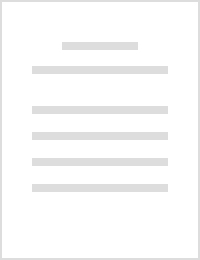
<p>(A – C) Low-order moments predicted by the model (y-axis) vs. measured in the data (x-ax... more <p>(A – C) Low-order moments predicted by the model (y-axis) vs. measured in the data (x-axis). Each data point is an average over an adaptive bin chosen to contain at least 100 cell pairs (B), or triplets (C). Error bars represent ±1 standard deviation. Shaded blue band indicates the 95% confidence interval. (D) Model prediction of individual word probabilities. Words that occurred fewer than 4 times in the data are omitted, as is the all-silent state (whose predictive accuracy is visible in (F)). The blue band indicates the 95% confidence interval, dashed line represents equality. (E) Comparison of model-predicted with empirical word frequencies, extended to include all observed words except the silent state. The gray band represents the range of values for synthetic data sampled from the fit model, with the number of samples matched to the test set (parametric bootstrap). This exhibits almost as much scatter as the true data, even though the model is exactly correct in this synthetic case. (F) Model prediction of population spike-count distribution <i>P</i>(<i>k</i>), where . <i>Inset</i>: same data as main panel, but with the y-axis log-scaled. Note the close match in the tail of the distribution, which corresponds to very rare events. All goodness-of-fit statistics displayed in this figure were calculated on a 50% held-out test set.</p
<p>(A) Values of the transition matrix, <i>P</i>(<i>α</i> | <i&g... more <p>(A) Values of the transition matrix, <i>P</i>(<i>α</i> | <i>β</i>), which describes the probability of finding mode α at time step <i>t</i> given the presence of mode <i>β</i> at time step <i>t-1</i> (color scale). Notice that with <i>M</i> = 70 modes, this is a 70x70 matrix. (B) Same transition matrix as in A but with only off-diagonal elements displayed. (C) Transition entropy, <i>H</i><sub><i>trans</i></sub>(<i>β</i>), of each mode plotted against the mode’s average firing rate, ⟨<i>k</i>⟩, for the full transition matrix (red) and for the renormalized matrix containing only the off-diagonal elements (blue); maximum possible transition entropy (dashed line).</p
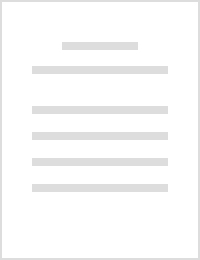
<p>(A,B) Discriminability of some example modes. For each mode, we collected all response w... more <p>(A,B) Discriminability of some example modes. For each mode, we collected all response words assigned to that mode (A-B, <i>top</i>). Words that occurred within one time bin of a transition between two modes were omitted. For each pair of modes, we then carried out Fisher’s linear discriminant analysis (LDA) to find the one-dimensional projection of the two word populations that maximized their discriminability (quantified by <i>d</i>′). A and B each summarize this analysis for an arbitrarily chosen example mode (black) and its nearest-neighbor (the mode with smallest <i>d</i>′), in red. <i>Top</i>: Rasters of unique words assigned to each of the two modes (rows indexing words, columns cells). <i>Bottom</i>: Distribution of LDA projections of the words shown above. (C) Blue points: <i>d</i>′ of nearest-neighbor modes. Discriminability is high and increases with <i>⟨k⟩</i>, consistent with the suggestion of panels A and B. Gray points: results of the same analysis carried out on shuffled data in which words were randomly assigned to modes, keeping the same number of words in each mode. Shuffled modes are highly overlapping, even though the LDA projection maximizes <i>d</i>′. (D) Blue: distribution of <i>d</i>′ for all mode pairs, not just nearest neighbor. Gray: result on shuffled data, as in (C).</p
The file contains spike time data from multi-electrode array recordings of salamander retinal gan... more The file contains spike time data from multi-electrode array recordings of salamander retinal ganglion cells under four stimulus conditions: a white noise checkerboard, a repeated natural movie, a non-repeated natural movie, and a bar exhibiting random one-dimensional motion. For each experiment, spike time data is provided in the file 'data.mat' and information necessary to reconstruct the stimulus and align to spike times is contained in 'aux_data' where relevant. All recordings were sampled at 10 kHz. Data is in Matlab .mat format
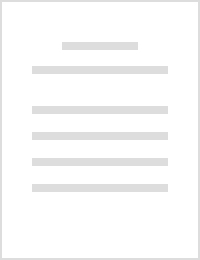
Across the nervous system, certain population spiking patterns are observed far more frequently t... more Across the nervous system, certain population spiking patterns are observed far more frequently than others. A hypothesis about this structure is that these collective activity patterns function as population codewords–collective modes–carrying information distinct from that of any single cell. We investigate this phenomenon in recordings of ∼150 retinal ganglion cells, the retina’s output. We develop a novel statistical model that decomposes the population response into modes; it predicts the distribution of spiking activity in the ganglion cell population with high accuracy. We found that the modes represent localized features of the visual stimulus that are distinct from the features represented by single neurons. Modes form clusters of activity states that are readily discriminated from one another. When we repeated the same visual stimulus, we found that the same mode was robustly elicited. These results suggest that retinal ganglion cells’ collective signaling is endowed with a form of error-correcting code–a principle that may hold in brain areas beyond retina
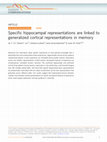
Nature communications, Jan 7, 2018
Memories link information about specific experiences to more general knowledge that is abstracted... more Memories link information about specific experiences to more general knowledge that is abstracted from and contextualizes those experiences. Hippocampal-cortical activity patterns representing features of past experience are reinstated during awake memory reactivation events, but whether representations of both specific and general features of experience are simultaneously reinstated remains unknown. We examined hippocampal and prefrontal cortical firing patterns during memory reactivation in rats performing a well-learned foraging task with multiple spatial paths. We found that specific hippocampal place representations are preferentially reactivated with the subset of prefrontal cortical task representations that generalize across different paths. Our results suggest that hippocampal-cortical networks maintain links between stored representations for specific and general features of experience, which could support abstraction and task guidance in mammals.
When correlations within a neural population are strong enough, neural activity in early visual a... more When correlations within a neural population are strong enough, neural activity in early visual areas is organized into a discrete set of clusters. Here, we show that a simple, biologically plausible circuit can learn and then readout in real-time the identity of experimentally measured clusters of retinal ganglion cell population activity. After learning, individual readout neurons develop cluster tuning, meaning that they respond strongly to any neural activity pattern in one cluster and weakly to all other inputs. Different readout neurons specialize for different clusters, and all input clusters can be learned, as long as the number of readout units is mildly larger than the number of input clusters. We argue that this operation can be repeated as signals flow up the cortical hierarchy.
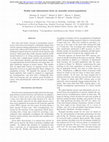
Over days and weeks, neural activity representing an animal’s position and movement in sensorimot... more Over days and weeks, neural activity representing an animal’s position and movement in sensorimotor cortex has been found to continually reconfigure or ‘drift’ during repeated trials of learned tasks, with no obvious change in behavior. This challenges classical theories which assume stable engrams underlie stable behavior. However, it is not known whether this drift occurs systematically, allowing downstream circuits to extract consistent information. We show that drift is systematically constrained far above chance, facilitating a linear weighted readout of behavioural variables. However, a significant component of drift continually degrades a fixed readout, implying that drift is not confined to a null coding space. We calculate the amount of plasticity required to compensate drift independently of any learning rule, and find that this is within physiologically achievable bounds. We demonstrate that a simple, biologically plausible local learning rule can achieve these bounds, ac...
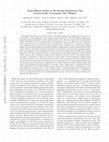
Throughout the brain, local circuits encode information using large populations of neurons, yet t... more Throughout the brain, local circuits encode information using large populations of neurons, yet the structure of this population code remains poorly understood. Previous studies applying the maximum entropy principle to retinal multi-electrode array data have shown that the joint probability distribution over all retinal ganglion cell population states is proliferated by local maxima, which represents a form of clustering of visual stimuli into a discrete set of categories. However, these studies focused on responses to highly repeated stimuli. Another recent study using a hidden Markov model approach likewise found that ganglion cell population activity partitioned into noiserobust clusters, and further that this clustering was present for the non-repeated stimulus regime. Here, we investigate the relationship between the clusters found using these two different modeling approaches. We first demonstrate that local maxima in the probability landscape result from the repeat structure...
Uploads
Papers by Adrianna Loback