Papers by Komminist Weldemariam
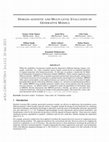
arXiv (Cornell University), Jan 20, 2023
While the capabilities of generative models heavily improved in different domains (images, text, ... more While the capabilities of generative models heavily improved in different domains (images, text, graphs, molecules, etc.), their evaluation metrics largely remain based on simplified quantities or manual inspection with limited practicality. To this end, we propose a framework for Multilevel Performance Evaluation of Generative mOdels (MPEGO), which could be employed across different domains. MPEGO aims to quantify generation performance hierarchically, starting from a sub-feature-based low-level evaluation to a global features-based high-level evaluation. MPEGO offers great customizability as the employed features are entirely user-driven and can thus be highly domain/problem-specific while being arbitrarily complex (e.g., outcomes of experimental procedures). We validate MPEGO using multiple generative models across several datasets from the material discovery domain. An ablation study is conducted to study the plausibility of intermediate steps in MPEGO. Results demonstrate that MPEGO provides a flexible, user-driven, and multi-level evaluation framework, with practical insights on the generation quality. The framework, source code, and experiments will be available at: https://github.com/GT4SD/mpego.

This study aimed at identifying the factors associated with neonatal mortality. We analyzed the D... more This study aimed at identifying the factors associated with neonatal mortality. We analyzed the Demographic and Health Survey (DHS) datasets from 10 Sub-Saharan countries. For each survey, we trained machine learning models to identify women who had experienced a neonatal death within the 5 years prior to the survey being administered. We then inspected the models by visualizing the features that were important for each model, and how, on average, changing the values of the features affected the risk of neonatal mortality. We confirmed the known positive correlation between birth frequency and neonatal mortality and identified an unexpected negative correlation between household size and neonatal mortality. We further established that mothers living in smaller households have a higher risk of neonatal mortality compared to mothers living in larger households; and that factors such as the age and gender of the head of the household may influence the association between household size...

ArXiv, 2018
This work views neural networks as data generating systems and applies anomalous pattern detectio... more This work views neural networks as data generating systems and applies anomalous pattern detection techniques on that data in order to detect when a network is processing an anomalous input. Detecting anomalies is a critical component for multiple machine learning problems including detecting adversarial noise. More broadly, this work is a step towards giving neural networks the ability to recognize an out-of-distribution sample. This is the first work to introduce "Subset Scanning" methods from the anomalous pattern detection domain to the task of detecting anomalous input of neural networks. Subset scanning treats the detection problem as a search for the most anomalous subset of node activations (i.e., highest scoring subset according to non-parametric scan statistics). Mathematical properties of these scoring functions allow the search to be completed in log-linear rather than exponential time while still guaranteeing the most anomalous subset of nodes in the network i...
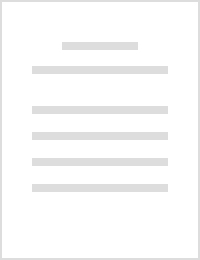
2019 IEEE International Conference on Healthcare Informatics (ICHI)
Clinical records capture the temporal, participatory, and interventional details of the care prov... more Clinical records capture the temporal, participatory, and interventional details of the care provision process. The exchange of these records plays a critical role in care continuity. Recently, there has been increasing attention on health data privacy and confidentiality which translates to questions on ownership and accessibility of clinical records. Traditional approaches to remedy this stand the risk of reducing the accessibility of these records, making care continuity across facilities more difficult. This poses a need for mechanisms that would enable the secure exchange of health data without adversely affecting the access to clinical records. This paper presents the Digital Health Wallet (DHW); a blockchain-enabled system that allows seamless clinical workflow orchestration and patient-mediated data exchange through consent management in a privacy-preserving manner. We conducted a preliminary test to benchmark the performance of DHW in resource-constrained healthcare facilities in developing countries.
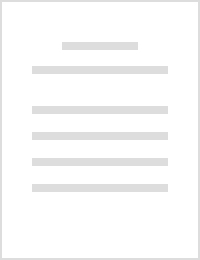
ICASSP 2020 - 2020 IEEE International Conference on Acoustics, Speech and Signal Processing (ICASSP), 2020
We investigate the effect of variational autoencoder (VAE) based data anonymization and its abili... more We investigate the effect of variational autoencoder (VAE) based data anonymization and its ability to preserve anomalous subgroup properties. We present a Utility Guaranteed Deep Privacy (UGDP) system which casts existing anomalous pattern detection methods as a new utility measure for data synthesis. UGDP’s approach shows that properties of an anomalous subset of records, identified in the original data set, are preserved through the anonymization of a VAE. This is despite the newly generated records being completely synthetic. More specifically, the Bias-Scan algorithm identifies a subgroup of records that are consistently over- (or under-) risked by a black-box classifier as an area of ’poor fit’. This scanning process is applied on both pre- and post- VAE synthesized data. The areas of poor fit (i.e. anomalous records) persist in both settings. We evaluate our approach using publicly available datasets from the financial industry. Our evaluation confirmed that the approach is able to produce synthetic datasets that preserved a high level of subgroup differentiation as identified initially in the original dataset. Such a distinction was maintained while having distinctly different records between the synthetic and original dataset.
This document describes the details of the BON Egocentric vision dataset. BON denotes the initial... more This document describes the details of the BON Egocentric vision dataset. BON denotes the initials of the locations where the dataset was collected; Barcelona (Spain); Oxford (UK); and Nairobi (Kenya). BON comprises first-person video, recorded when subjects were conducting common office activities. The preceding version of this dataset, FPV-O dataset has fewersubjects for only a single location (Barcelona). To develop a location agnostic framework, data from multiple locations and/or office settings is essential. Thus, BON comprises videos from an increased number of participants and office settings, resulting in a six-fold increase in the number of video segments, i.e., 2639 (BON) vs. 464 (FPV-O). In the follow up sections, we describe the details of the dataset, data collection, stratification across activities, duration, locations, and participants (genders)
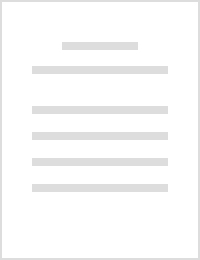
2017 IEEE/ACM 39th International Conference on Software Engineering: Software Engineering in Society Track (ICSE-SEIS), 2017
In this paper, we address the problem of improving data collection of the education system by pre... more In this paper, we address the problem of improving data collection of the education system by presenting School Census Hub (SCH). The SCH concept emerged from field studies with stakeholders in Kenya. The goal of these studies were to help unlocking three key high-level requirements for the design of SCH. i) Budget allocation, allocating budget should be based on a verifiable number of active students and teachers, ii) Spending, spending on assets should be transparent and verifiable, iii) and, Improving learning environment, unlocking the limited insight into statistical relationship between school effectiveness and demographic variables. We present the overall architecture and design of SCH based on the findings from the field studies. The first version supporting a core set of capabilities for school data collection has been implemented. To evaluate the system, we conducted a large scale pilot in 97 schools. We report on a usability study of SCH that demonstrates user awareness and support for data acquisition and reporting in education management information system in Sub-Sharan Africa.
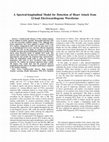
2020 42nd Annual International Conference of the IEEE Engineering in Medicine & Biology Society (EMBC), 2020
Cardiovascular diseases (CVDs) remain responsible for millions of deaths annually. Myocardial inf... more Cardiovascular diseases (CVDs) remain responsible for millions of deaths annually. Myocardial infarction (MI) is the most prevalent condition among CVDs. Although datadriven approaches have been applied to predict CVDs from ECG signals, comparatively little work has been done on the use of multiple-lead ECG traces and their efficient integration to diagnose CVDs. In this paper, we propose an end-to-end trainable and joint spectral-longitudinal model to predict heart attack using data-level fusion of multiple ECG leads. The spectral stage transforms the time-series waveforms to stacked spectrograms and encodes the frequency-time characteristics, whilst the longitudinal model helps to utilise the temporal dependency that exists in these waveforms using recurrent networks. We validate the proposed approach using a public MI dataset. Our results show that the proposed spectrallongitudinal model achieves the highest performance compared to the baseline methods.
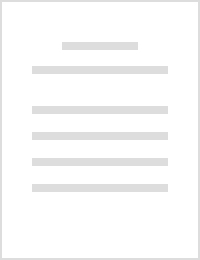
2019 IEEE Global Conference on Signal and Information Processing (GlobalSIP), 2019
Bias in data can have unintended consequences which propagate to the design, development, and dep... more Bias in data can have unintended consequences which propagate to the design, development, and deployment of machine learning models. In the financial services sector, this can result in discrimination from certain financial instruments and services. At the same time, data privacy is of paramount importance, and recent data breaches have seen reputational damage for large institutions. Presented in this paper is a trusted model-lifecycle management platform that attempts to ensure consumer data protection, anonymization, and fairness. Specifically, we examine how datasets can be reproduced using deep learning techniques to effectively retain important statistical features in datasets whilst simultaneously protecting data privacy and enabling safe and secure sharing of sensitive personal information beyond the current state-of-practice.
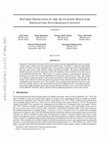
Pattern Recognition Letters, 2021
Generative Adversarial Networks (GANs) have recently achieved unprecedented success in photoreali... more Generative Adversarial Networks (GANs) have recently achieved unprecedented success in photorealistic image synthesis from low-dimensional random noise. The ability to synthesize high-quality content at a large scale brings potential risks as the generated samples may lead to misinformation that can create severe social, political, health, and business hazards. We propose SubsetGAN to identify generated content by detecting a subset of anomalous node-activations in the inner layers of pre-trained neural networks. These nodes, as a group, maximize a non-parametric measure of divergence away from the expected distribution of activations created from real data. This enable us to identify synthesised images without prior knowledge of their distribution. SubsetGAN efficiently scores subsets of nodes and returns the group of nodes within the pre-trained classifier that contributed to the maximum score. The classifier can be a general fake classifier trained over samples from multiple sources or the discriminator network from different GANs. Our approach shows consistently higher detection power than existing detection methods across several state-of-the-art GANs (PGGAN, StarGAN, and CycleGAN) and over different proportions of generated content.

Knowledge Management and Acquisition for Intelligent Systems, 2021
Visual inspection of electrocardiograms (ECGs) is a common clinical practice to diagnose heart di... more Visual inspection of electrocardiograms (ECGs) is a common clinical practice to diagnose heart diseases (HDs), which are still responsible for millions of deaths globally every year. In particular, myocardial infarction (MI) is the leading cause of mortality among HDs. ECGs reflect the electrical activity of the heart and provide a quicker process of diagnosis compared to laboratory blood tests. However, still it requires trained clinicians to interpret ECG waveforms, which poses a challenge in low-resourced healthcare systems, such as poor doctorto-patient ratios. Previous works in this space have shown the use of data-driven approaches to predict HDs from ECG signals but focused on domain-specific features that are less generalizable across patient and device variations. Moreover, limited work has been conducted on the use of longitudinal information and fusion of multiple ECG leads. In contrast, we propose an end-to-end trainable solution for MI diagnosis, which (1) uses 12 ECG leads; (2) fuses the leads at data-level by stacking their spectrograms; (3) employs transfer learning to encode features rather than learning representations from scratch; and (4) uses a recurrent neural network to encode temporal dependency in long duration ECGs. Our approach is validated using multiple datasets, including tens of thousands of subjects, and encouraging performance is achieved.
arXiv: Atmospheric and Oceanic Physics, 2020
In an effort to provide optimal inputs to downstream modeling systems (e.g., a hydrodynamics mode... more In an effort to provide optimal inputs to downstream modeling systems (e.g., a hydrodynamics model that simulates the water circulation of a lake), we hereby strive to enhance resolution of precipitation fields from a weather model by up to 9x. We test two super-resolution models: the enhanced super-resolution generative adversarial networks (ESRGAN) proposed in 2017, and the content adaptive resampler (CAR) proposed in 2020. Both models outperform simple bicubic interpolation, with the ESRGAN exceeding expectations for accuracy. We make several proposals for extending the work to ensure it can be a useful tool for quantifying the impact of climate change on local ecosystems while removing reliance on energy-intensive, high-resolution weather model simulations.

ArXiv, 2019
In this paper, we investigate the effect of machine learning based anonymization on anomalous sub... more In this paper, we investigate the effect of machine learning based anonymization on anomalous subgroup preservation. In particular, we train a binary classifier to discover the most anomalous subgroup in a dataset by maximizing the bias between the group's predicted odds ratio from the model and observed odds ratio from the data. We then perform anonymization using a variational autoencoder (VAE) to synthesize an entirely new dataset that would ideally be drawn from the distribution of the original data. We repeat the anomalous subgroup discovery task on the new data and compare it to what was identified pre-anonymization. We evaluated our approach using publicly available datasets from the financial industry. Our evaluation confirmed that the approach was able to produce synthetic datasets that preserved a high level of subgroup differentiation as identified initially in the original dataset. Such a distinction was maintained while having distinctly different records between th...
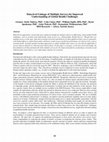
AMIA ... Annual Symposium proceedings. AMIA Symposium, 2021
Data-driven approaches can provide more enhanced insights for domain experts in addressing critic... more Data-driven approaches can provide more enhanced insights for domain experts in addressing critical global health challenges, such as newborn and child health, using surveys (e.g., Demographic Health Survey). Though there are multiple surveys on the topic, data-driven insight extraction and analysis are often applied on these surveys separately, with limited efforts to exploit them jointly, and hence results in poor prediction performance of critical events, such as neonatal death. Existing machine learning approaches to utilise multiple data sources are not directly applicable to surveys that are disjoint on collection time and locations. In this paper, we propose, to the best of our knowledge, the first detailed work that automatically links multiple surveys for the improved predictive performance of newborn and child mortality and achieves cross-study impact analysis of covariates.

2020 IEEE International Conference on Blockchain (Blockchain), 2020
Attacks targeting several millions of non-internet based application users are on the rise. These... more Attacks targeting several millions of non-internet based application users are on the rise. These applications such as SMS and USSD typically do not benefit from existing multi-factor authentication methods due to the nature of their interaction interfaces and mode of operations. To address this problem, we propose an approach that augments blockchain with multi-factor authentication based on evidence from blockchain transactions combined with risk analysis. A profile of how a user performs transactions is built overtime and is used to analyse the risk level of each new transaction. If a transaction is flagged as high risk, we generate n-factor layers of authentication using past endorsed blockchain transactions. A demonstration of how we used the proposed approach to authenticate critical financial transactions in a blockchainbased asset financing platform is also discussed.

Contraceptive use improves the health of women and children in several ways, yet data shows high ... more Contraceptive use improves the health of women and children in several ways, yet data shows high rates of discontinuation which is not well understood. We introduce an AI-based decision platform capable of analyzing event data to identify patterns of contraceptive uptake that are unique to a subpopulation of interest. These discriminatory patterns provide valuable, interpretable insights to policymakers. The sequences then serve as a hypothesis for downstream causal analysis to estimate the effect of specific variables on discontinuation outcomes. Our platform presents a way to visualize, stratify, compare, and perform a causal analysis on covariates that determine contraceptive uptake behavior, and yet is general enough to be extended to a variety of applications. 1 Study of Contraceptive Discontinuation Family Planning (FP) has emerged as a crucial component of sustainable global development [Osotimehin, 2015]. Effective use of contraceptives can significantly improve the nutritio...
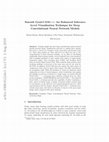
ArXiv, 2019
Gaining insight into how deep convolutional neural network models perform image classification an... more Gaining insight into how deep convolutional neural network models perform image classification and how to explain their outputs have been a concern to computer vision researchers and decision makers. These deep models are often referred to as black box due to low comprehension of their internal workings. As an effort to developing explainable deep learning models, several methods have been proposed such as finding gradients of class output with respect to input image (sensitivity maps), class activation map (CAM), and Gradient based Class Activation Maps (Grad-CAM). These methods under perform when localizing multiple occurrences of the same class and do not work for all CNNs. In addition, Grad-CAM does not capture the entire object in completeness when used on single object images, this affect performance on recognition tasks. With the intention to create an enhanced visual explanation in terms of visual sharpness, object localization and explaining multiple occurrences of objects ...

Reliably detecting attacks in a given set of inputs is of high practical relevance because of the... more Reliably detecting attacks in a given set of inputs is of high practical relevance because of the vulnerability of neural networks to adversarial examples. These altered inputs create a security risk in applications with real-world consequences, such as self-driving cars, robotics and financial services. We propose an unsupervised method for detecting adversarial attacks in inner layers of autoencoder (AE) networks by maximizing a non-parametric measure of anomalous node activations. Previous work in this space has shown AE networks can detect anomalous images by thresholding the reconstruction error produced by the final layer. Furthermore, other detection methods rely on data augmentation or specialized training techniques which must be asserted before training time. In contrast, we use subset scanning methods from the anomalous pattern detection domain to enhance detection power without labeled examples of the noise, retraining or data augmentation methods. In addition to an anom...
Existing datasets available to address crucial problems, such as child mortality and family plann... more Existing datasets available to address crucial problems, such as child mortality and family planning discontinuation in developing countries, are not ample for data-driven approaches. This is partly due to disjoint data collection efforts employed across locations, times, and variations of modalities. On the other hand, state-of-the-art methods for small data problem are confined to image modalities. In this work, we proposed a data-level linkage of disjoint surveys across Sub-Saharan African countries to improve prediction performance of neonatal death and provide cross-domain explainability.
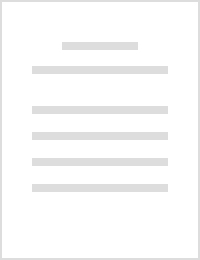
Proceedings of the Ninth International Conference on Information and Communication Technologies and Development, 2017
Several initiatives have been proposed to collect, report, and analyze data about school systems ... more Several initiatives have been proposed to collect, report, and analyze data about school systems for supporting decision-making. These initiatives rely mostly on self-reported and summarized data collected irregularly and rarely. They also lack a single independent and systematic process to validate the collected data during its entire lifecycle. Furthermore, schools in developing countries still do not maintain complete and up-to-date school records. Due to these and other factors addressing the education challenges in those countries remains a high priority for local and international governments, donor and non-governmental agencies across the world. In this paper, we discuss our initial design, implementation, and evaluation of a blockchain-enabled School Information Hub (SIH) using Kenya's school system as a case study.
Uploads
Papers by Komminist Weldemariam