Papers by Shankar Thawkar
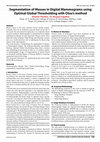
IJCST, 2014
Breast Cancer is the most common Cancer among women. Currently there are no methods to prevent br... more Breast Cancer is the most common Cancer among women. Currently there are no methods to prevent breast cancer that is the reason why early detection represents a very important factor in cancer treatment. Mammography is currently the best available radiological technique for early detection of breast cancer. Mass detection using Computer Aided Design and Detection systems serve as a second decision tool to radiologists for discovering masses in the mammograms. This work presents a methodology for segmentation of masses in Mammogram Images. The proposed algorithm uses Median filtering, Optimal Global thresholding using Otsu's method and morphological processing in order to: (1) enhance quality of mammography image, (2) Segmentation of masses based on threshold resulting Region of Interest (ROI)(3) extracting segmented masses from image. The proposed techniques are implemented and tested under MATLAB environment.
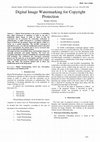
IJCSIT, 2012
Digital Watermarking is the process of embedding data called watermark or signature or label or t... more Digital Watermarking is the process of embedding data called watermark or signature or label or tag into a multimedia object (image or audio or video) so that the watermark can be extracted for ownership verification or authentication. A visible watermark is a secondary translucent image overlaid into the primary image and appears visible to a viewer on a careful inspection. The invisible watermark is embedded in such a way that the modifications made to the pixel value is perceptually not noticed and it can be recovered only with an appropriate decoding mechanism This paper presents an invisible image watermarking scheme for copyright protection and temper detection. The secret key encryption algorithm is used for embedding the watermark using LSB technique. The verification (the watermark extraction) process uses the same key as in encryption, and hence it can be used for copyright protection of digital media such as images, audio and video. The proposed method able to detect any modification made in the image pixels.
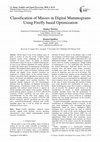
Breast cancer is one of the leading causes of death in women all over the world. Computer based d... more Breast cancer is one of the leading causes of death in women all over the world. Computer based diagnosis system assists radiologist in the effective treatment of breast cancer. To design an efficient classification system for masses in digital mammograms, we have to use efficient algorithms for feature selection to reduce the feature space of mammogram classification problem. The proposed study explores the use of Firefly algorithm to select a subset of features. Artificial neural network and support vector machine classifiers are employed to evaluate fitness of the selected features. Features selected by Firefly algorithm are used to classify masses into benign and malignant, using artificial neural network and support vector machine classifiers. The proposed method employed over 651 mammograms obtained from the Database of Digitized Screen-film Mammograms. Classification results show that Firefly algorithm with artificial neural network is superior to Firefly algorithm with support vector machine. Artificial neural network achieves accuracy of 95.23% with 94.43% sensitivity, 93.94% specificity and area under curve Az=0.965±0.008. On the other hand, support vector machine classifier achieves an accuracy of 92.47% with 96.14% sensitivity, 88.53% specificity and area under curve Az=0.951±0.009.Results obtained with Firefly algorithm shows that it will be useful for effective treatment of breast cancer.

All over the world, breast cancer is the second leading cause of death in women above 40 years of... more All over the world, breast cancer is the second leading cause of death in women above 40 years of age. To design an efficient classification system for breast cancer diagnosis, one has to use efficient algorithms for feature selection to reduce the feature space of mammogram classification. The current work investigates the use of hybrid genetic ensemble method for feature selection and classification of masses. Genetic algorithm (GA) is used to select a subset of features and to evaluate the fitness of the selected features, Adaptive boosting (AdaBoost) and Random Forest (RF) ensembles with 10-fold cross-validation are employed. The selected features are used to classify masses into benign or malignant using AdaBoost, RF, and single Decision Tree (DT) classifiers. The performance evaluation of classifiers indicates that AdaBoost outperforms both RF and single DT classifiers. AdaBoost achieves an accuracy of 96.15%, with 97.32% sensitivity, 95.90% speci-ficity, and area under curve of A Z = 0.982 ± 0.004. The results obtained with the proposed method are better when compared with extant research work.
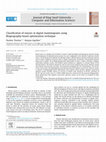
Breast cancer is a leading cause of death in women in both developed and developing countries. De... more Breast cancer is a leading cause of death in women in both developed and developing countries. Design and development of computer-based systems can assist radiologists in the effective treatment of breast cancer. For the design of an efficient classification system, efficient feature selection techniques must be used to reduce complexity of feature space in digital mammogram classification. The proposed methodology aims to explore use of Biogeography-based optimization to select a subset of features. Adaptive neuro-fuzzy inference system and artificial neural network are employed to evaluate fitness of the selected features. The features selected are used to train and test adaptive neuro-fuzzy inference system and artificial neural network classifiers. The experiment employed over 651 mammograms. The classification results shows that Biogeography-based optimization with adaptive neuro-fuzzy inference system is superior to Biogeography-based optimization with artificial neural network. Adaptive neuro-fuzzy inference system classifier achieve an accuracy of 98.92% with sensitivity of 99.10%, specificity of 98.72% and area under curve A Z = 0.999 ± 0.000. Outcomes achieved with the proposed Biogeography-based optimization with adaptive neuro-fuzzy inference system are far better as compared to some recent work. Ó 2018 The Authors. Production and hosting by Elsevier B.V. on behalf of King Saud University. This is an open access article under the CC BY-NC-ND license (http://creativecommons.org/licenses/by-nc-nd/4.0/).
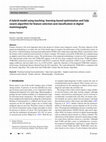
Springer Nature, 2021
Feature selection is the most important step in the design of a breast cancer diagnosis system. T... more Feature selection is the most important step in the design of a breast cancer diagnosis system. The basic objective of the proposed methodology is to reduce the size of the feature space to improve the performance of the classification system. In this article, a hybrid teaching-learning based optimization (TLBO) with a Salp swarm algorithm (SSA) is presented to select the features with an artificial neural network as a fitness evaluator. The features selected by TLBO-SSA are evaluated using an adaptive neuro-fuzzy inference system. The performance of the proposed methodology is tested over 651 mammograms. The experimental results show that TLBO-SSA appears to be the best when compared with the basic TLBO algorithm. TLBO-SSA archived an accuracy of 98.46% with 98.81% sensitivity, 98.08% specificity, 0.9852 F-score, 0.9692 Cohen's kappa coefficient, and area under curve A Z = 0.997 ± 0.001. Again the robustness of the proposed TLBO-SSA method is tested using a benchmark dataset obtained from the UCI repository. The result obtained by TLBO-SSA is compared with the Genetic Algorithm. The results show that TLBO-SSA is better than the Genetic Algorithm.

Breast cancer is still one of the leading diseases in women all over the World. Mammography is th... more Breast cancer is still one of the leading diseases in women all over the World. Mammography is the best tool to discover breast tumor in its early stage. The Computer based system will assist the radiologist for detection and classification of masses. These systems will help to improve breast cancer diagnosis and minimize unnecessary biopsies. In this work we investigate efficient methods for classification of masses into benign and malignant to improve breast cancer diagnosis. Initially Twenty-five features based on intensity, texture and shape are extracted from each of the detected masses. Then six most significant features are selected by step-wise Forward Logistic Regression Technique. These features are used to train and test Support Vector Machine, K-Nearest Neighbor and Decision Tree classifiers with 10-fold cross validation. The experiment was conducted on 651 mammogram images with 314 benign and 337 malignant cases obtained from Digital Database for screening Mammography. The performance evaluation of classifiers indicates that SVM with radial bias function is better than both K-NN and Decision Tree classifier. Our method achieve best results of 97.32% sensitivity, 90.44% specificity and 94% accuracy for SVM with area under Receiver Operating Characteristics curve AZ=0.963±0.008. All the results achieved are promising when compared with some existing works.
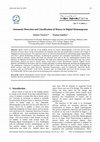
Breast Cancer is still one of the leading cancers in women. Mammography is the best tool for earl... more Breast Cancer is still one of the leading cancers in women. Mammography is the best tool for early detection of breast cancer. In this work methods for automatic detection and classification of masses into benign or malignant has been proposed. The suspicious masses are detected automatically by performing image segmentation with Otsu's global thresholding technique, morphological operations and watershed transformation. Twenty-five features based on intensity, texture and shape are extracted from each of the 651 mammograms obtained from Database of Digitized Screen-film Mammograms. The Eight most significant features selected by step-wise Linear Discriminate Analysis are used to classify masses using Fisher's Linear Discriminate Analysis, Support Vector Machine and Multilayer Perceptron with two training algorithms Levenberg-Marquardt and Bayesian Regularization. The performance evaluation of classifiers indicates that MLP is better than both LDA and SVM. MLP-RBF has 98.9% accuracy with area under Receiver Operating Characteristics curve AZ=0.98±0.007, MLP-LM 96.0% accuracy with AZ=0.97±0.007, SVM 91.4% accuracy with AZ=0.956±0.009 and LDA 90.3% accuracy with AZ=0.956±0.009. All the results achieved are promising when compared with some existing work.
Uploads
Papers by Shankar Thawkar