Papers by Carlos E Thomaz
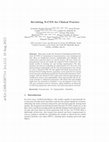
arXiv (Cornell University), Aug 10, 2023
This paper revisits the Neonatal Convolutional Neural Network (N-CNN) by optimizing its hyperpara... more This paper revisits the Neonatal Convolutional Neural Network (N-CNN) by optimizing its hyperparameters and evaluating how they affect its classification metrics, explainability and reliability, discussing their potential impact in clinical practice. We have chosen hyperparameters that do not modify the original N-CNN architecture, but mainly modify its learning rate and training regularization. The optimization was done by evaluating the improvement in F1 Score for each hyperparameter individually, and the best hyperparameters were chosen to create a Tuned N-CNN. We also applied soft labels derived from the Neonatal Facial Coding System, proposing a novel approach for training facial expression classification models for neonatal pain assessment. Interestingly, while the Tuned N-CNN results point towards improvements in classification metrics and explainability, these improvements did not directly translate to calibration performance. We believe that such insights might have the potential to contribute to the development of more reliable pain evaluation tools for newborns, aiding healthcare professionals in delivering appropriate interventions and improving patient outcomes.
Although it is widely accepted that corporate reputation influences organizationstakeholder inter... more Although it is widely accepted that corporate reputation influences organizationstakeholder interactions, there is no theoretical framework that conceptualizes this aspect in stakeholders' decision-making processes for establishing various forms of relationships with a firm. By adopting an interdisciplinary approach, this article provides a theoretical model that explains the role corporate reputation has in the process through which stakeholders decide to establish relationships with a firm. It is argued that the stakeholder decision-making process for exchange with a company is based on several exchange rules: corporate reputation, social legitimacy, pragmatic legitimacy, and exchange benefits. The article concludes with a case study of James Hardie Industries in Australia, which illustrates the function of the proposed conceptual model.
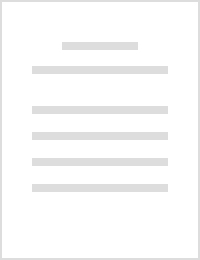
SAE technical paper series, Sep 22, 2015
In order to make devices partially or completely autonomous, it is imperative nowadays to extract... more In order to make devices partially or completely autonomous, it is imperative nowadays to extract relevant information from the myriad of data available. In the last years, it has become very common to use images as signals of interest to propose feasible solution to this problem. Image recognition can be used with high accuracy rates when the object of interest or the environment are controlled or well known. However, in open urban spaces, for instance, where there are all sorts of visual artifacts and stimuli (information), the segmentation of the object of interest (foreground) from the rest of the image (background) is a challenging issue. One possible way to tackle this problem is to use low-depth of field images, which analogously to our visual perception highlight the object of interest from the rest of the image. In this work, some methods and algorithms for segmenting low-depth of field images are analyzed and compared, providing an updated and contextualized version of the state-of-the-art of this topic.
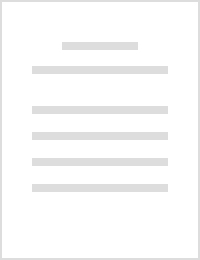
In this paper we present a nonlinear version of the discriminant principal component analysis, na... more In this paper we present a nonlinear version of the discriminant principal component analysis, named NDPCA, that is based on kernel support vector machines (KSVM) and the AdaBoost technique. Specifically, the problem of ranking principal components, computed from two-class databases, is addressed by applying the AdaBoost procedure in a nested loop: each iteration of the inner loop boosts weak classifiers to a moderate one while the outer loop combines the moderate classifiers to build the global discriminant vector. In the proposed NDPCA, each weak learner is a linear classifier computed through a separating hyperplane defined by a KSVM decision boundary in the PCA space. We compare the proposed methodology with counterpart ones using facial expressions of the Radboud and Jaffe image databases. Our experimental results have shown that NDPCA outperforms the PCA in classification tasks. Also, it is competitive if compared with counterpart techniques given also suitable results for reconstruction.
Anais do ... Congresso Ibero-Latino-Americano de Métodos Computacionais em Engenharia, 2015
Computer Vision and Image Understanding, Oct 1, 2023
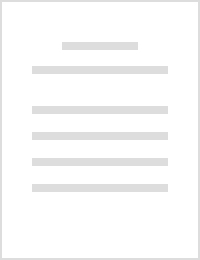
IEEE Latin America Transactions, Mar 1, 2016
Owing to its compact and controlled environment, chess has provided in the last few decades a fru... more Owing to its compact and controlled environment, chess has provided in the last few decades a fruitful domain for fundamental questions about human reasoning and promoted the development of several research papers in different areas of scientific knowledge. This paper describes and implements a computational framework for acquiring and processing electroencephalography signals of players with different levels of experience with the goal of identifying distinct patterns of cognitive brain mapping during specific well-known chess problems. Our experimental results have showed a neural organisation consistent with the activity performed by the sample groups of volunteers who took part in this study, highlighting discriminant differences in cortical brain areas between beginners and specialists ranked by their proficiency levels. We believe that such information would be helpful for the formulation of more efficient methodologies to teaching and learning chess.
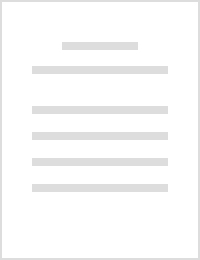
IEEE Latin America Transactions, Apr 1, 2022
Identification of appropriate content-based features for the description of audio signals can pro... more Identification of appropriate content-based features for the description of audio signals can provide a better repre-sentation of naturalistic music stimuli which, in recent years, have been used to understand how the human brain processes such information. In this work, an extensive clustering analysis has been carried out on a large and benchmark audio dataset to assess whether features commonly extracted in the literature are in fact statistically relevant. Our results show that not all of these well-known acoustic features might be statistically necessary. We also demonstrate quantitatively that, regardless of the musical genre, the same acoustic feature is selected to represent each cluster. This finding discloses that there is a general redundancy among the set of audio descriptors used, that does not depend on a particular music track or genre, allowing an expressive reduction of the number of features necessary to identify apropriate time instants on the audio for further brain signal processing of music stimuli.
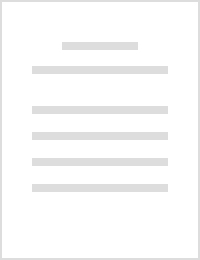
IEEE Latin America Transactions, 2021
Electroencephalography (EEG) is an important toolfor the study of the human brain because it prov... more Electroencephalography (EEG) is an important toolfor the study of the human brain because it provides potentiallyuseful signals for understanding the spatial and temporal dynam-ics of neural information processing. These signals are commonlyrepresented by vector or matrix mathematical structures, whichmay counteract their natural behaviour for a multidimensionalrepresentation. Thus, in this case, the information from an EEGsignal should be represented using tensors. This study presentsan analysis of how these different mathematical structures canbe explored to obtain functional brain information. Two matrixmodels and one tensor model were investigated and assessed usingbrain maps and classification results. Our results show at leastthree different and complementary ways for the representationof cognitive brain maps and, as far as our exploratory analysis isconcerned, the tensorial model stands out in terms of the highestlevel of compression and precision in comparison to the othermodels.

The feature extraction is one of the most important steps in face analysis applications and this ... more The feature extraction is one of the most important steps in face analysis applications and this subject always received attention in the computer vision and pattern recognition areas due to its applicability and wide scope. However, to define the correct spatial relevance of physiognomical features remains a great challenge. It has been proposed recently, with promising results, a statistical spatial mapping technique that highlights the most discriminating facial features using some task driven information from data mining. Such priori information has been employed as a spatial weighted map on Local Binary Pattern (LBP), that uses Chi-Square distance as a nearest neighbour based classifier. Intending to reduce the dimensionality of LBP descriptors and improve the classification rates we propose and implement in this paper two quad-tree image decomposition algorithms to task related spatial map segmentation. The first relies only on split step (top-down) of distinct regions and the second performs the split step followed by a merge step (bottom-up) to combine similar adjacent regions. We carried out the experiments with two distinct face databases and our preliminary results show that the top-down approach achieved similar classification results to standard segmentation using though less regions.

Springer eBooks, 2001
In several pattern recognition problems, particularly in image recognition ones, there are often ... more In several pattern recognition problems, particularly in image recognition ones, there are often a large number of features available, but the number of training examples for each pattern is significantly less than the dimension of the feature space. This statement implies that the sample group covariance matrices often used in the Gaussian maximum probability classifier are singular. A common solution to this problem is to assume that all groups have equal covariance matrices and to use as their estimates the pooled covariance matrix calculated from the whole training set. This paper uses an alternative estimate for the sample group covariance matrices, here called the mixture covariance, given by an appropriate linear combination of the sample group and pooled covariance matrices. Experiments were carried out to evaluate the performance associated with this estimate in two biometric applications: face and facial expression. The average recognition rates obtained by using the mixture covariance matrices were higher than the usual estimates.
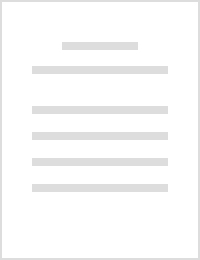
International Journal of Pattern Recognition and Artificial Intelligence, Apr 10, 2017
Multilinear principal component analysis (MPCA) has been applied for tensor decomposition and dim... more Multilinear principal component analysis (MPCA) has been applied for tensor decomposition and dimensionality reduction in image databases modeled through higher order tensors. Despite the well-known attractive properties of MPCA, the traditional approach does not incorporate prior information in order to steer its subspace computation. In this paper, we propose a method to explicitly incorporate such semantics in the MPCA framework to allow an automatic selective treatment of the variables that compose the patterns of interest. The method relies on spatial weights calculated, in this work, by separating hyperplanes and Fisher criterion. In this way, we can perform feature extraction and dimensionality reduction taking advantage of high level information in the form of labeled data. Besides, the corresponding tensor components are ranked in order to identify the principal weighted tensor subspaces for classification tasks. In the computational results we consider gender and facial expression experiments to illustrate the capabilities of the method for dimensionality reduction, classification and reconstruction of face images.
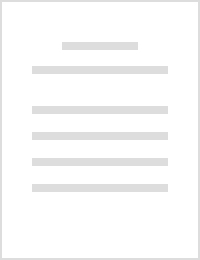
Traditionally, proficiency in chess has been measured by metrics based on accuracy and response t... more Traditionally, proficiency in chess has been measured by metrics based on accuracy and response time or performance in tournaments, but not considering how cognitive signals influence in the decision making in this complex game. In this work, we have carried out a performance analysis of chess players comparing a standard ranking measure with a novel one proposed here. Using the idea of treating participants eye movements and brain signals, when answering several on-screen valid chess questions of distinguished complexities, as high-dimensional data we have shown that expertise is consistently associated with the ability to process visual information holistically using fewer fixations rather than locally focusing on individual pieces. Results show that traditional metric to quantify proficiency presented accuracy up to 73,3%, while the proposed cognitive one reached accuracy up to 87,5% and 98,9% for the electroencephalography and eye movements, respectively. These findings might disclose new insights for teaching and predicting chess skills.
... where ji x , is the n-dimensional pattern j from class i π , i N is the number of training pa... more ... where ji x , is the n-dimensional pattern j from class i π , i N is the number of training patterns from class i ... Although their experimental results have shown that CLDA improves the performance of a face recognition system compared with Liu et al.'s ... Yu and Yang's Method (DLDA) ...
Uploads
Papers by Carlos E Thomaz