Papers by plaban bhowmick
Communications of the ACM
In this paper, we propose a system for automatic segmentation and semantic annotation of verbose ... more In this paper, we propose a system for automatic segmentation and semantic annotation of verbose queries with predefined metadata fields. The problem of generating optimal segmentation has been modeled as a simulated annealing problem with proposed solution cost function and neighborhood function. The annotation problem has been modeled as a sequence labeling problem and has been implemented with Hidden Markov Model (HMM). Component-wise and holistic evaluation of the system have been performed using gold standard annotation developed over query log collected from National Digital Library (NDLI) (National Digital Library of India: https://ndl.iitkgp.ac.in). In component-wise evaluation, the segmentation module yields 82% F1 and the annotation module performs with 56% accuracy. In holistic evaluation, the F1 of the system has been obtained to be 33%.
Books form a significant part of the National Digital Library of India (NDLI). However, extractin... more Books form a significant part of the National Digital Library of India (NDLI). However, extracting metadata from these books is difficult owing to variations in style, graphic fonts, and use of background images. This paper presents a lightweight tool to automatically extract metadata from academic books. We also describe results of a preliminary evaluation of our tool on school books indexed in NDLI.
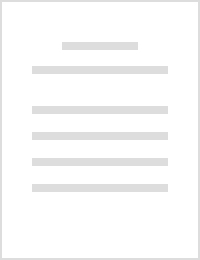
2019 ACM/IEEE Joint Conference on Digital Libraries (JCDL)
With the advancement in semantic technologies, structured data in different domains is being mode... more With the advancement in semantic technologies, structured data in different domains is being modelled as knowledge graphs. Bibliographic information has proven rather acquiescent to being formalized as knowledge graphs or information networks. In this paper, we address the problem of retrieving related resources in a bibliographic information network like SciGraph. Discovery of path patterns representing a set of example pairs of related resources forms the basis of our solution. We have adopted a bi-directional search strategy to accommodate state-of-the-art similarity measure (viz. HeteSim), relevant to Heterogeneous Information Networks (HIN). The proposed method has been evaluated based on precision and execution time. On experimenting with different datasets (YAGO and Springer Nature SciGraph), we observe an improvement in performance, compared to forward search algorithm, which is based on Path Constrained Random Walk similarity measure.
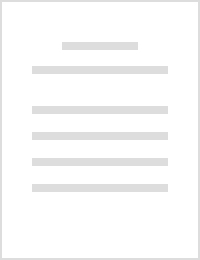
International Journal of Artificial Intelligence in Education
Video lectures are considered as one of the primary media to cater good-quality educational conte... more Video lectures are considered as one of the primary media to cater good-quality educational content to the learners. The video lectures illustrate the course-relevant concepts with necessary details. However, they sometimes fail to offer a basic understanding of off-topic concepts. Such off-topic concepts may spawn cognitive overload among the learners if those concepts are not familiar to them. To address this issue, we present a video lecture augmentation system that identifies the off-topic concepts and links them to relevant video lecture segments to furnish a basic understanding of the concerned concepts. Our augmentation system segregated the video lectures by identifying topical shifts in the lectures using a word embedding-based technique. The video segments were indexed on the basis of the underlying concepts. Identification of off-topic concepts was performed by modeling inter-concept relations in a semantic space. For each off-topic concept, appropriate video segments were fetched and re-ranked such that the top-ranked video segment offers the most basic understanding of the target off-topic concept. The proposed augmentation system was deployed as a web-based learning platform. Performance of the constituent modules was measured by using a manually curated dataset consisting of six video courses from the National Programme on Technology Enhanced Learning (NPTEL) archive. Feedback from 12 research scholars was considered to assess the quality of augmentations and usability of the learning platform. Both system and human-based evaluation indicated that the recommended augmentations were able to offer a basic understanding of the concerned off-topic concepts.
SN Computer Science, 2022
2021 ACM/IEEE Joint Conference on Digital Libraries (JCDL), 2021
Digital libraries generally need to process a large volume of diverse document types. The collect... more Digital libraries generally need to process a large volume of diverse document types. The collection and tagging of metadata is a long, error-prone, workforce-consuming task. We are attempting to build an automatic metadata extractor for digital libraries. In this work, we present the Heterogeneous Learning Resources (HLR) dataset for document image classification. The individual learning resource is first decomposed into its constituent document images (sheets) which are then passed through an OCR tool to obtain the textual representation. The document image and its textual content are classified with state-of-the-art classifiers. Finally, the labels of the constituent document images are used to predict the label of the overall document.

Proceedings of the International Conference on Web Intelligence, 2017
The current study presents a two-stage question retrieval approach which, in the rst phase, retri... more The current study presents a two-stage question retrieval approach which, in the rst phase, retrieves similar questions for a given query using a deep learning based approach and in the second phase, re-ranks initially retrieved questions on the basis of interquestion similarities. The suggested deep learning based approach is trained using several surface features of texts and the associated weights are pre-trained using a deep generative model for better initialization. The proposed retrieval model outperforms standard baseline question retrieval approaches. The proposed re-ranking approach performs inference over a similarity graph constructed with the initially retrieved questions and re-ranks the questions based on their similarity with other relevant questions. Suggested re-ranking approach signi cantly improves the precision for the retrieval task.
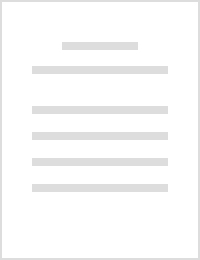
Proceedings of the 18th ACM/IEEE on Joint Conference on Digital Libraries, 2018
Large digital libraries often index articles without curating their digital copies in their own r... more Large digital libraries often index articles without curating their digital copies in their own repositories. Examples include the National Digital Library of India (NDLI) and ACM Digital Library. Full text view generally requires subscription to libraries that host the contents. The problem is particularly severe for researchers, given high journal subscription charges. However, authors often keep a free copy in preprint servers. Sometimes a conference paper behind a paywall has a closely resembling journal version freely available on the Web. These open access surrogates are immensely valuable to researchers who cannot afford to access the original publications. We present a lightweight tool called Surrogator to automatically identify open access surrogates of access-restricted scholarly papers present in a digital library. Its focus on approximate matches makes it different from many existing applications. In this poster, we describe the design and interface of the tool and our initial experiences of using it on articles indexed in NDLI.
2019 IEEE 16th India Council International Conference (INDICON), 2019
The National Digital Library of India (NDLI) is envisioned as a national educational asset to ena... more The National Digital Library of India (NDLI) is envisioned as a national educational asset to enable 24x7 learning for learners of all ages and disciplines. It indexes research papers from various publishers, but the full text is often access-restricted and therefore, not freely available to users of NDLI. However, full texts of many papers are available in institutional digital repositories and preprint servers. We have developed a browser extension Illumine that allows NDLI users to automatically search the web and retrieve full texts of papers whenever they are available. We describe the design of the tool and report experiments done on a corpus of papers indexed in NDLI. The tool is freely available to all NDLI users.
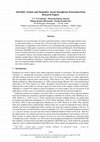
Keyphrases in a research paper succinctly capture the primary content of the paper and also assis... more Keyphrases in a research paper succinctly capture the primary content of the paper and also assist in indexing the paper at a concept level. Given the huge rate at which scientific papers are published today, it is important to have effective ways of automatically extracting keyphrases from a research paper. In this paper, we present a novel method, Syntax and Semantics Aware Keyphrase Extraction (SaSAKE), to extract keyphrases from research papers. It uses a transformer architecture, stacking up sentence encoders to incorporate sequential information, and graph encoders to incorporate syntactic and semantic dependency graph information. Incorporation of these dependency graphs helps to alleviate long-range dependency problems and identify the boundaries of multi-word keyphrases effectively. Experimental results on three benchmark datasets show that our proposed method SaSAKE achieves state-of-the-art performance in keyphrase extraction from scientific papers.
Proceedings of the 18th ACM/IEEE on Joint Conference on Digital Libraries, 2018
In this paper, we present preliminary results on a novel task of extracting comparison points for... more In this paper, we present preliminary results on a novel task of extracting comparison points for a pair of entities from the text articles describing them. The task is challenging as comparison points in a typical pair of articles tend to be sparse. We presented a multi-level document analysis (viz. document, paragraph and sentence level) for extracting the comparisons. For extracting sentence level comparisons, which is the hardest task among three, we have used Convolutional Neural Network (CNN) with features extracted around triple. Experiments conducted on a small dataset provide encouraging performance.
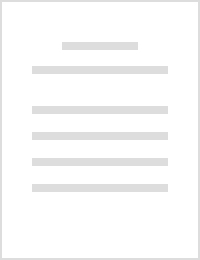
This paper presents a Query Auto-Completion (QAC) framework that aims at assisting users in a dig... more This paper presents a Query Auto-Completion (QAC) framework that aims at assisting users in a digital library to specify their search intent with reduced effort. The proposed system suggests metadata-based facets to users as they specify their queries into the system. In this work, we model the facet-based QAC problem as frequent pattern mining problem where the system aims at leveraging association among different facet combinations. Among several frequent pattern mining algorithms, the present work make use of FP-Growth to discover facet patterns at large-scale. These facet patterns represented in form of association rules are used for online query auto-completion or suggestion. A prototype QAC augmented digital library search system is implemented by considering a limited bibliographic dataset (35K resources) of the National Digital Library of India (NDLI: https://ndl.iitkgp.ac.in) portal. We perform extensive experiments to measure the quality of query suggestions and QAC augmen...
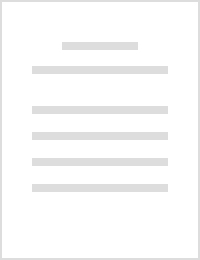
According to the concept of emotional intelligence, a person exhibits truly intelligent behavior ... more According to the concept of emotional intelligence, a person exhibits truly intelligent behavior if he is able to understand emotions of other persons and reciprocate with proper responses. In the drive for developing intelligent machines, this new paradigm of intelligence, emotional intelligence is being taken into consideration. The area of human computer interaction (HCI) focuses on developing intuitive interfaces for enabling natural communication between human and computing systems. The naturalness of communication depends on the recognition and expression of emotions. Thus, in very recent days, efforts are being put forward for developing natural interfaces that are emotionally intelligent. Emotion can be expressed through different modes of communication like facial expression, vocal or speech expression, different bio-signals etc. Apart from these modes, language is another important mode through which emotions are often communicated. Thus, the interfaces that use natural language as the communication mode must understand the emotional contents in the users’ interactions. As compared to emotion recognition from facial and speech expressions, the problem of emotion recognition in linguistic expressions has not received much attention. The problem of emotion classification in natural language text is the task of classifying text segments (word, sentence, paragraph or document) into different emotion categories like happiness, sadness, fear etc. Emotion in text can be studied in two different views. The first view adheres to the emotions expressed by the writer in a text segment and the second view concerns about the emotions that may be evoked in a reader’s mind in response to a text stimulus. In this work, we focus on the second view where for a given sentence the task is to identify the emotions that are possibly evoked. We have adopted a supervised machine learning based text categorization approach to solve the said task. In order to perform supervised classification, a corpus of sentences has been collected. Sometimes the news articles are written in such a way that they evoke some emotions in readers’ minds. This is why the news domain has been selected as the corpus source in this study. The collected corpus is annotated with basic emotion categories. As evocation of multiple emotions is possible, fuzzy and multi-label classification is most natural where a sentence may evoke multiple emotions simultaneously with different degrees of membership in respective emotion categories. Accordingly, a fuzzy and multi-label annotation scheme has been adopted. As emotion is a very subjective entity, the responses of the readers may vary. Thus, corpus annotated by a single reader or annotator may not be reliable to develop emotion classifiers. In order to circumvent the problem of subjectivity, the corpus has been annotated by multiple annotators independently. The reliability in annotation has been determined by measuring the extent of agreement among the annotators. Two types of classification tasks have been addressed in this work. The first task determines whether a sentence evokes a particular emotion or not, i.e., a crisp classification. On the other hand, the second task determines to what extent a particular emotion is evoked, i.e., a fuzzy classification. In this work, we have computed the reliability in crisp or categorical annotation by means of a proposed agreement measure. The reliability in fuzzy annotation has been measured using a standard reliability measure, Cronbach’s alpha. The gold standard corpus for training and validating the emotion classifiers has been generated using proposed aggregation techniques. As there are a limited number of studies regarding emotion evocation, the features suitable for emotion classification has not been explored much. As words present in the sentences are the most obvious features, they have been considered in the baseline study. Besides word feature, two new features have been proposed. The polarity based features consist of the polarity of the subject, object and verb phrases of the sentences. As the evocation of emotion depends on the reader’s understanding the text, semantics based features has also been considered. The methods for extracting these features have been proposed. The generated gold standard corpus has been used to develop emotion classifiers. The emotion classification task has been performed in two frameworks: crisp multi-label and fuzzy multi-label classification framework. Three different research questions have been addressed in this work. The first investigation has been performed to find the most discriminating feature combination for emotion classification. In this study, it is observed that polarity and semantics based feature combination performs best. Experiments have been performed to identify important word and semantics based features by using statistical feature selection technique. It has been observed that for both word…

In this paper, we propose a framework that facilitates the use of heterogeneous data sources to r... more In this paper, we propose a framework that facilitates the use of heterogeneous data sources to represent a plan state. The traditional planners use monolithic predicate based schema for plan state representation where the world state is described as a set of predicates that are currently true. However, this approach is not efficient because, firstly, in reality, the world state can be obtained by aggregating information from different modular sources represented through multiple knowledge representation techniques and secondly, the performance of a planner can be affected when the size of state is enormously large. To overcome the stated limitations, we redefine the notion of a plan state and represent it as the combination of state predicates as well as the references to non-predicate data sources like databases, ontologies etc. The main challenge of our work is to handle plan state representation using distributed heterogeneous data sources, without altering planning algorithm in concern. Though we have based our idea around HTN planning, the approach is applicable to other planners, without any additional overhead.
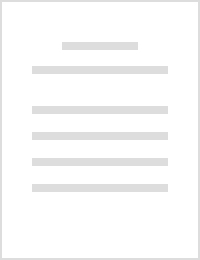
Proceedings of the ACM/IEEE Joint Conference on Digital Libraries in 2020, 2020
Author name ambiguity is a common problem in digital libraries. The problem occurs because multip... more Author name ambiguity is a common problem in digital libraries. The problem occurs because multiple individuals may share the same name and the same individual may be represented by various names. Researchers have proposed various techniques for author name disambiguation (AND). In this paper, we study AND in the context of research publications indexed in the PubMed citation database. We perform an empirical study where we experiment with two ensemble-based classification algorithms, namely, random forest and gradient boosted decision trees, on a publicly available corpus of manually disambiguated author names from PubMed. Results show that random forest produces higher accuracy, precision, recall and F1-score, but gradient boosted trees perform competitively. We also determine which features are most discriminative given the feature set and the classifiers.
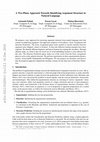
We propose a new approach for extracting argument structure from natural language texts that cont... more We propose a new approach for extracting argument structure from natural language texts that contain an underlying argument. Our approach comprises of two phases: Score Assignment and Structure Prediction. The Score Assignment phase trains models to classify relations between argument units (Support, Attack or Neutral). To that end, different training strategies have been explored. We identify different linguistic and lexical features for training the classifiers. Through ablation study, we observe that our novel use of word-embedding features is most effective for this task. The Structure Prediction phase makes use of the scores from the Score Assignment phase to arrive at the optimal structure. We perform experiments on three argumentation datasets, namely, AraucariaDB, Debatepedia and Wikipedia. We also propose two baselines and observe that the proposed approach outperforms baseline systems for the final task of Structure Prediction.
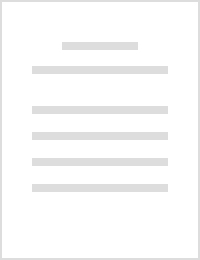
Journal of Information Science
Author names in bibliographic databases often suffer from ambiguity owing to the same author appe... more Author names in bibliographic databases often suffer from ambiguity owing to the same author appearing under different names and multiple authors possessing similar names. It creates difficulty in associating a scholarly work with the person who wrote it, thereby introducing inaccuracy in credit attribution, bibliometric analysis, search-by-author in a digital library and expert discovery. A plethora of techniques for disambiguation of author names has been proposed in the literature. In this article, we focus on the research efforts targeted to disambiguate author names specifically in the PubMed bibliographic database. We believe this concentrated review will be useful to the research community because it discusses techniques applied to a very large real database that is actively used worldwide. We make a comprehensive survey of the existing author name disambiguation (AND) approaches that have been applied to the PubMed database: we organise the approaches into a taxonomy; descri...
Uploads
Papers by plaban bhowmick